Walking Speed Classification from Marker-Free Video Images in Two-Dimension Using Optimum Data and a Deep Learning Method.
Bioengineering (Basel, Switzerland)(2022)
摘要
Walking speed is considered a reliable assessment tool for any movement-related functional activities of an individual (i.e., patients and healthy controls) by caregivers and clinicians. Traditional video surveillance gait monitoring in clinics and aged care homes may employ modern artificial intelligence techniques to utilize walking speed as a screening indicator of various physical outcomes or accidents in individuals. Specifically, ratio-based body measurements of walking individuals are extracted from marker-free and two-dimensional video images to create a walk pattern suitable for walking speed classification using deep learning based artificial intelligence techniques. However, the development of successful and highly predictive deep learning architecture depends on the optimal use of extracted data because redundant data may overburden the deep learning architecture and hinder the classification performance. The aim of this study was to investigate the optimal combination of ratio-based body measurements needed for presenting potential information to define and predict a walk pattern in terms of speed with high classification accuracy using a deep learning-based walking speed classification model. To this end, the performance of different combinations of five ratio-based body measurements was evaluated through a correlation analysis and a deep learning-based walking speed classification test. The results show that a combination of three ratio-based body measurements can potentially define and predict a walk pattern in terms of speed with classification accuracies greater than 92% using a bidirectional long short-term memory deep learning method.
更多查看译文
关键词
bi-LSTM,deep learning,marker-free video,optimal feature,ratio-based body measurement,redundant feature,two-dimensional (2D) image,walking speed,walking speed classification
AI 理解论文
溯源树
样例
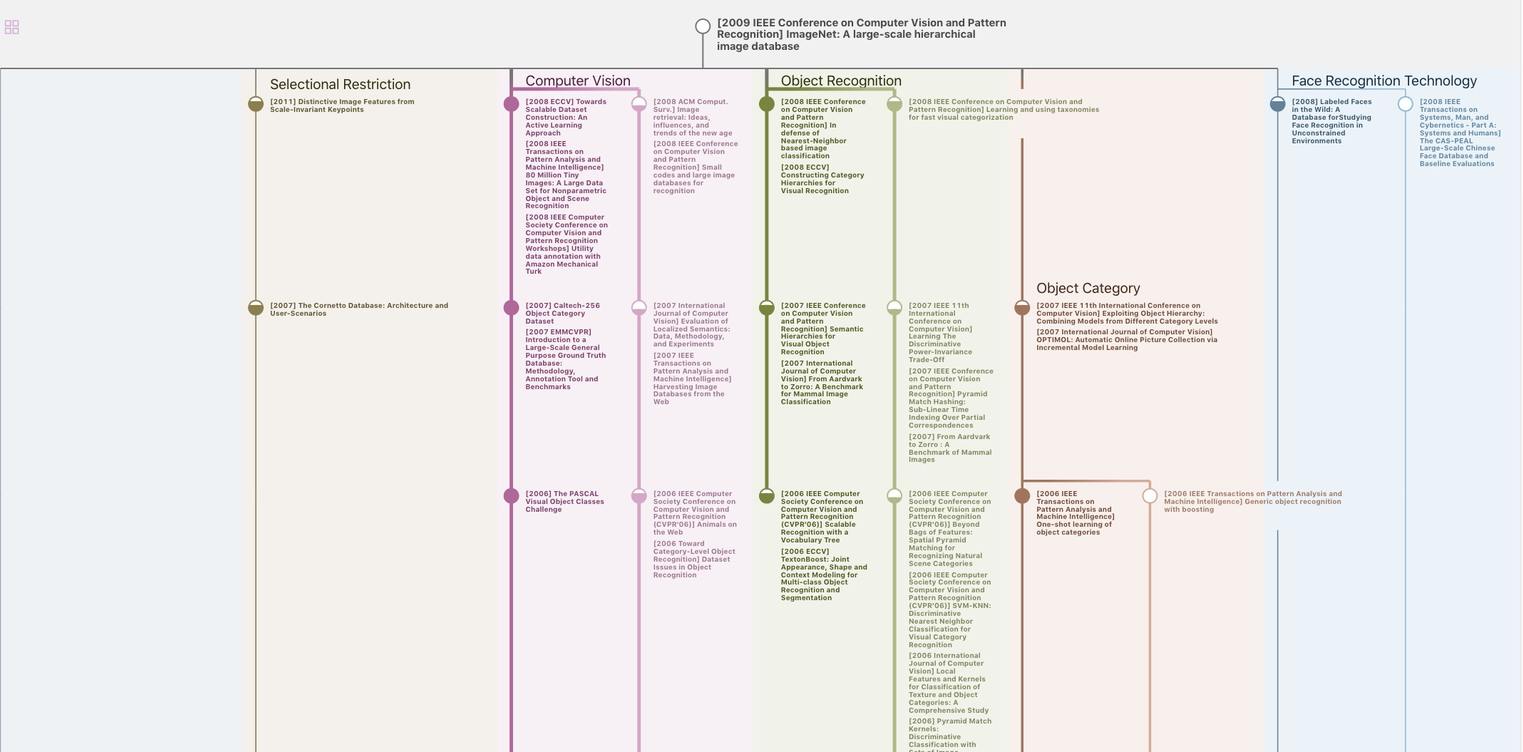
生成溯源树,研究论文发展脉络
Chat Paper
正在生成论文摘要