A Communication Channel Density Estimating Generative Adversarial Network
2019 IEEE Cognitive Communications for Aerospace Applications Workshop (CCAAW)(2019)
摘要
Autoencoder-based communication systems use neural network channel models to backwardly propagate message reconstruction error gradients across an approximation of the physical communication channel. In this work, we develop and test a new generative adversarial network (GAN) architecture for the purpose of training a stochastic channel approximating neural network. In previous research, investigators have focused on additive white Gaussian noise (AWGN) channels and/or simplified Rayleigh fading channels, both of which are linear and have well defined analytic solutions. Given that training a neural network is computationally expensive, channel approximation networks-and more generally the autoencoder systems-should be evaluated in communication environments that are traditionally difficult. To that end, our investigation focuses on channels that contain a combination of non-linear amplifier distortion, pulse shape filtering, intersymbol interference, frequency-dependent group delay, multipath, and non-Gaussian statistics. Each of our models are trained without any prior knowledge of the channel. We show that the trained models have learned to generalize over an arbitrary amplifier drive level and constellation alphabet. We demonstrate the versatility of our GAN architecture by comparing the marginal probability density function of several channel simulations with that of their corresponding neural network approximations.
更多查看译文
关键词
machine learning,neural network,generative adversarial network,communication,channel approximation
AI 理解论文
溯源树
样例
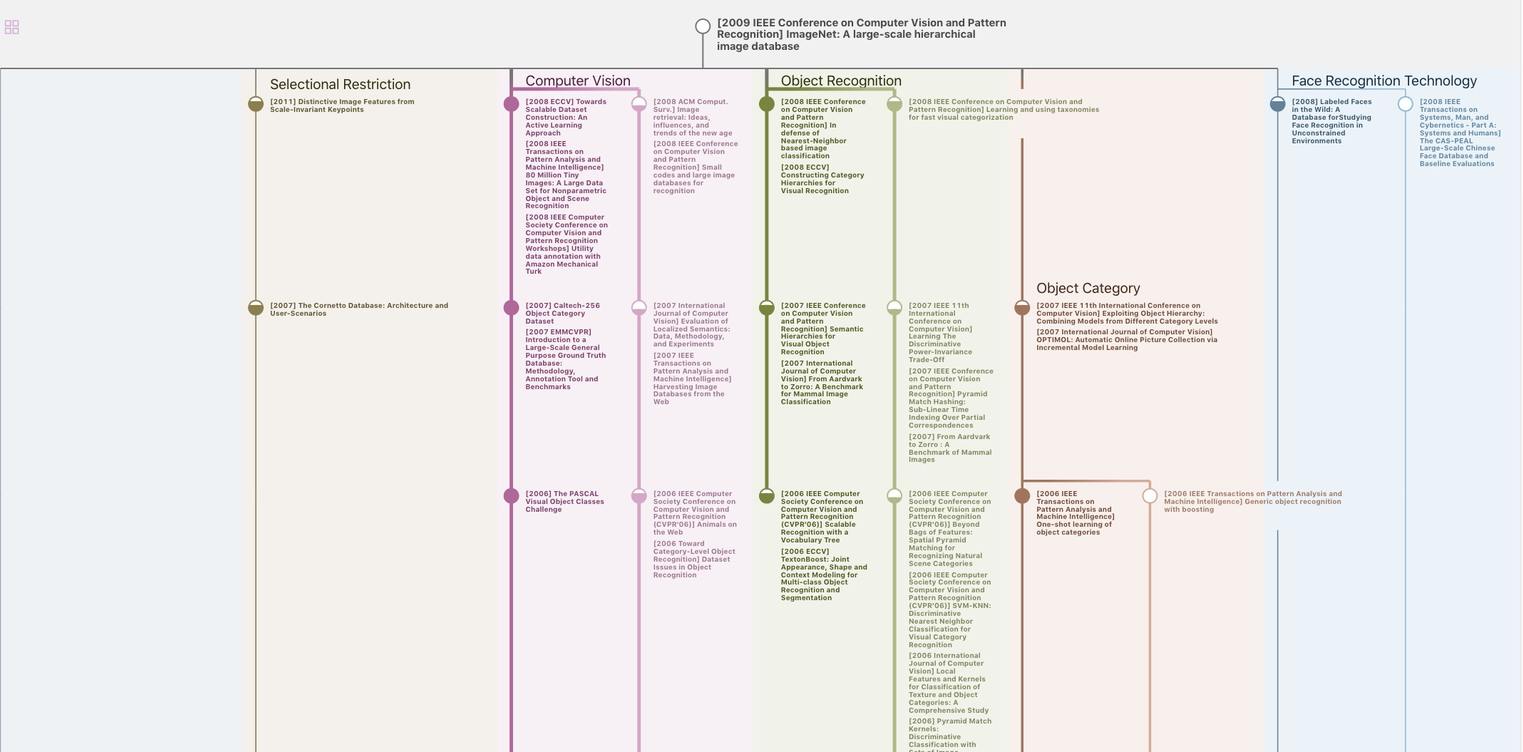
生成溯源树,研究论文发展脉络
Chat Paper
正在生成论文摘要