FBMOT: Flow Bridges the Gap between Detection and Tracking in Multiple Object Tracking
2022 18th IEEE International Conference on Advanced Video and Signal Based Surveillance (AVSS)(2022)
摘要
The detection performance is a key bottleneck to the performance of multiple object tracking (MOT) algorithms and the advanced detection algorithms contribute a large portion to the success of MOT algorithms. Even though, the detectors can still make false detections based on only the image frames, which may directly result in the loss or mismatch of tracks. In MOT tasks, we point out that the video frames are temporally correlated and the temporal relationship can be leveraged to further improve the performance of detection and subsequent tracking. In our work, we propose FBMOT which uses optical flow to compute a prior heatmap about the locations of previously tracked objects in the current frame and takes the heatmap as an additional input in detection. We further proposed a novel regularization loss to help our model distinguish useful information in the prior heatmap. As a result, our method improves 0.8, 1.0 and 2.7 MOTA in MOT16, MOT17 and MOT20 test datasets respectively.
更多查看译文
AI 理解论文
溯源树
样例
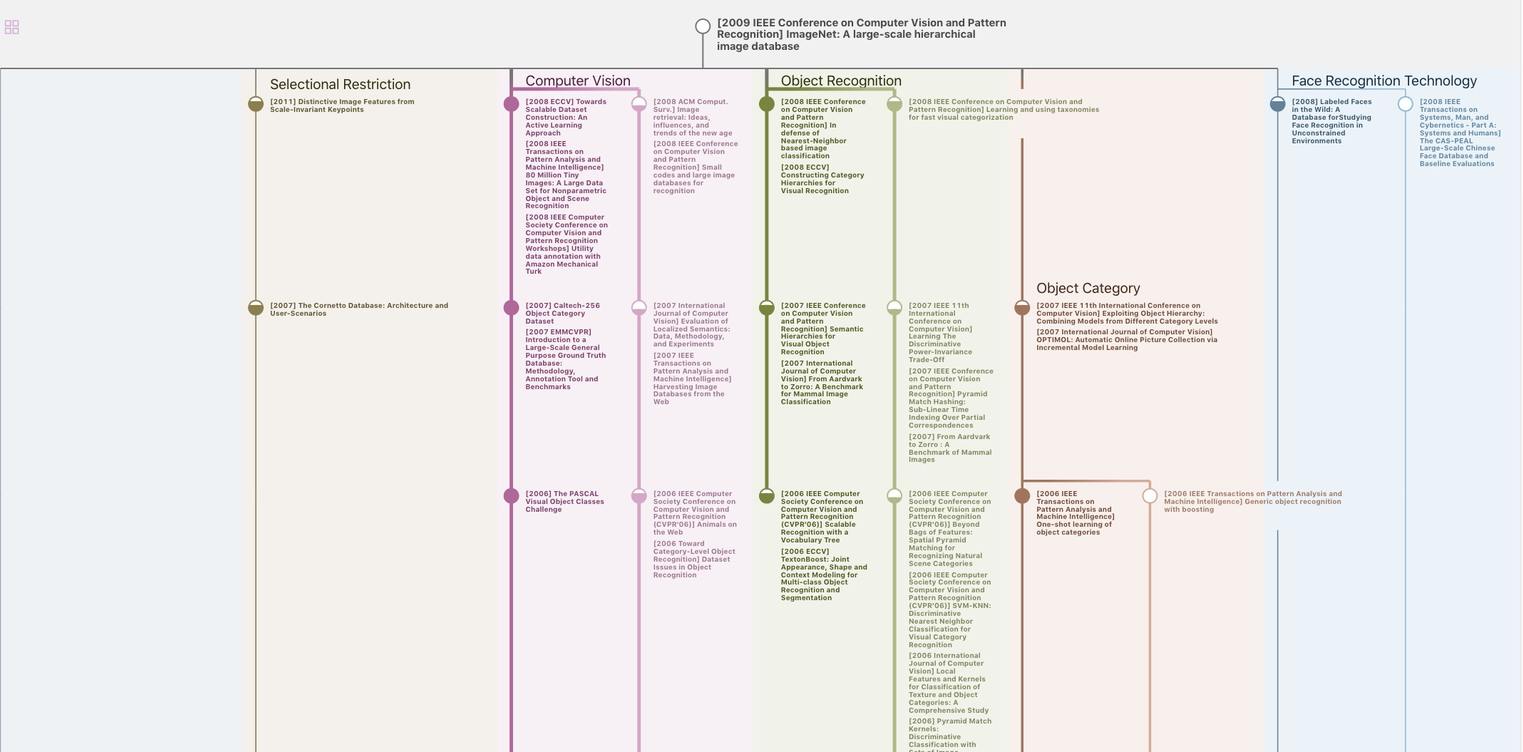
生成溯源树,研究论文发展脉络
Chat Paper
正在生成论文摘要