Computational platform for doctor-AI cooperation in PAH prognostication: a pilot study
ERJ Open Research(2022)
摘要
BackgroundPulmonary arterial hypertension (PAH) is a heterogenous and complex pulmonary vascular disease associated with substantial morbidity. Machine learning algorithms (used in many PAH risk calculators) can combine established parameters with thousands of circulating biomarkers to optimize PAH prognostication, but these approaches do not offer the clinician insight into what parameters drove the prognosis. The approach proposed in this study diverges from other contemporary phenotyping methods by identifying patient-specific parameters driving clinical risk.MethodsWe trained a random forest (RF) algorithm to predict 4-year survival risk in a cohort of 167 adult PAH patients evaluated at Stanford university, with 20% withheld for (internal) validation. Another cohort of 38 patients from Sheffield university were used as a secondary (external) validation. Shapley values, borrowed from game theory, were computed to rank the input parameters based on their importance to the predicted risk score for the entire trained RF model (global importance) and for an individual patient (local importance).ResultsBetween the internal and external validation cohorts, the RF model predicted 4-year risk of death/transplant with a sensitivity and specificity between 71.0–100% and 81.0–89.0%, respectively. The model reinforced the importance of established prognostic markers, but also identified novel inflammatory biomarkers that predict risk in some PAH patients.ConclusionThese results stress the need for advancing individualized phenotyping strategies that integrate clinical and biochemical data with outcome. The computational platform presented in this study offers a critical step towards personalized medicine in which a clinician can interpret an algorithm's assessment of an individual patient.
更多查看译文
AI 理解论文
溯源树
样例
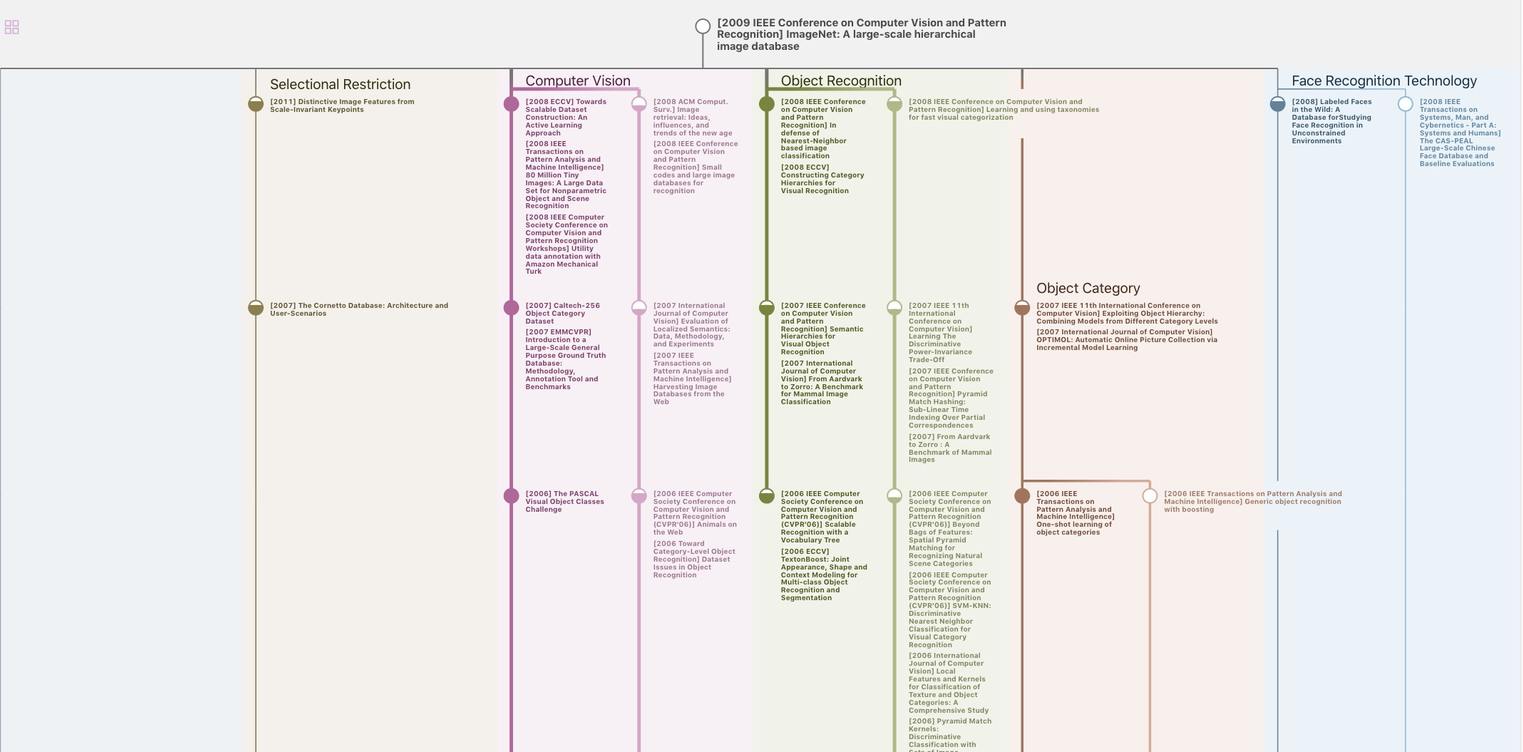
生成溯源树,研究论文发展脉络
Chat Paper
正在生成论文摘要