A Novel ML-Aided Methodology for SINS/GPS Integrated Navigation Systems during GPS Outages
REMOTE SENSING(2022)
摘要
To improve the navigation accuracy for land vehicles during global positioning system (GPS) outages, a machine learning (ML) aided methodology to integrate a strap-down inertial navigation system (SINS) and GPS system is proposed, as follows. When a GPS signal is available, an online sequential extreme learning machine with a dynamic forgetting factor (DOS-ELM) algorithm is used to train the mapping model between the SINS' acceleration, specific force, speed/position increments outputs, and the GPS' speed/position increments. When a GPS signal is unavailable, GPS speed/velocity measurements are replaced with prediction output of the well-trained DOS-ELM module's prediction output, and information fusion with the SINS reduces the degree of system error divergence. A land vehicle field experiment's actual sensor data were collected online, and the DOS-ELM-aided methodology for the SINS/GPS integrated navigation systems was applied. The simulation results indicate that the proposed methodology can reduce the degree of system error divergence and then obtain accurate and reliable navigation information during GPS outages.
更多查看译文
关键词
global positioning system (GPS) outages,strap-down inertial navigation system (SINS),machine learning (ML),online sequential extreme learning machine with dynamic forgetting factor (DOS-ELM),Kalman filtering (KF),SINS,GPS integrated systems
AI 理解论文
溯源树
样例
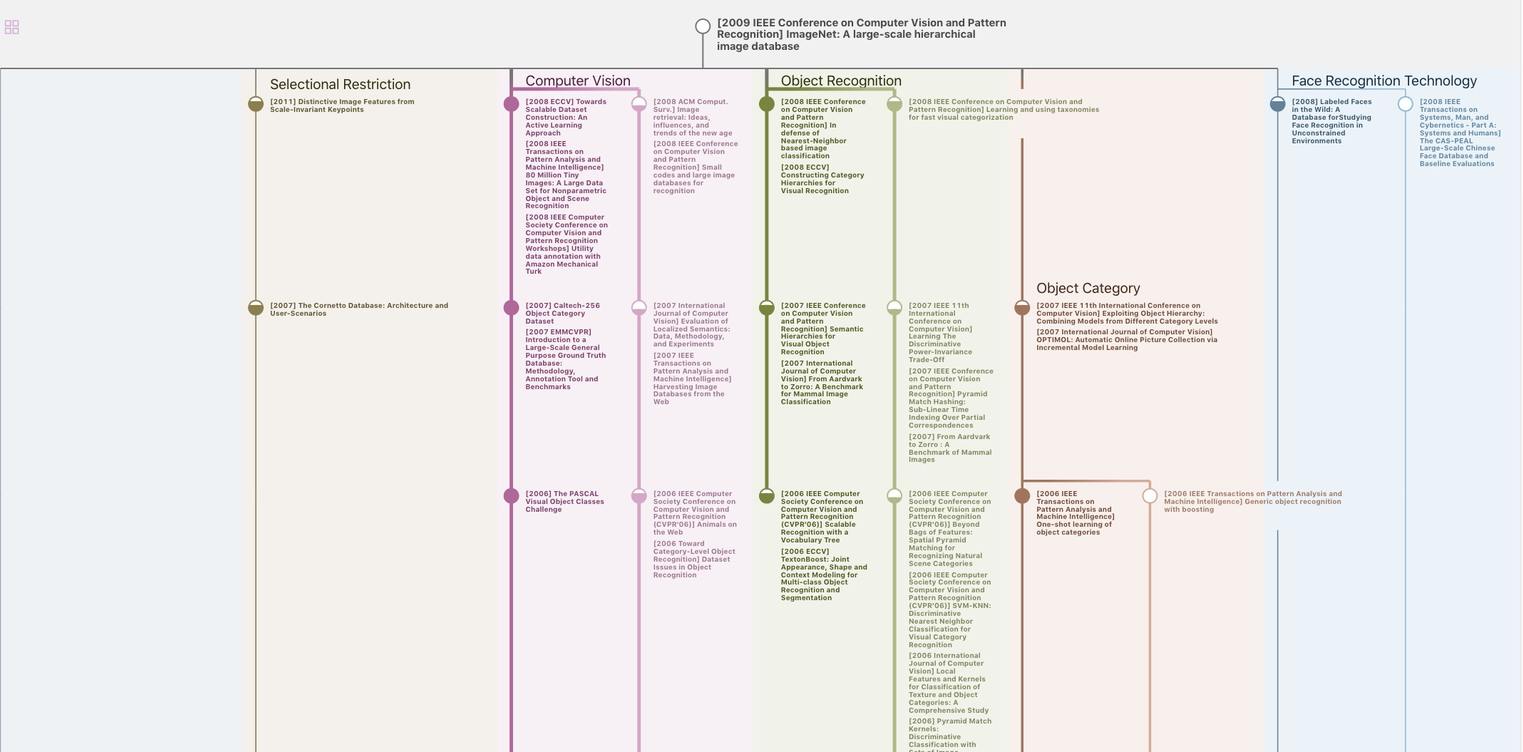
生成溯源树,研究论文发展脉络
Chat Paper
正在生成论文摘要