Loop Optimization Noise-Reduced LSTM Based Classifier for PD Detection
IEEE Transactions on Industry Applications(2023)
摘要
Partial discharge (PD) is an important inducement of power failures such as insulation degradation. Effective, timely, and economical detection of the PD is the basis for maintaining power system stability. This paper proposes a loop optimization noise-reduced LSTM based classifier for PD detection of large-scale data disturbed with measurement noise. It establishes the loop optimization to greatly coordinate the data processing and data-driven based machine learning algorithm. The noise reduction, feature extraction, and the long short-term memory (LSTM) neural network are combined as a whole with internal linkages. The proposed method combines these three necessary steps to achieve a better solution for the PD detection, while other methods are in general single ones, and not dedicated designed for the solution. The combination of the different methods has combined advantages and can overcome each individual's drawback. This comprehensive solution is verified using large real trial data measured from medium voltage (MV) overhead power lines. It exhibits superior performance and great practicality for PD detection of MV overhead power lines with an accuracy of 95.4%. It outperforms other techniques in the literature based on the experimental results. This paper also summarizes the loop optimization rules for PD detection solutions based on extensive experiments and analysis. The loop optimization rules are competent for the optimization of wider engineering applications of PD detection.
更多查看译文
关键词
Detection solution,feature extraction,loop optimization,neural network applications,noise reduction,partial discharges,signal processing
AI 理解论文
溯源树
样例
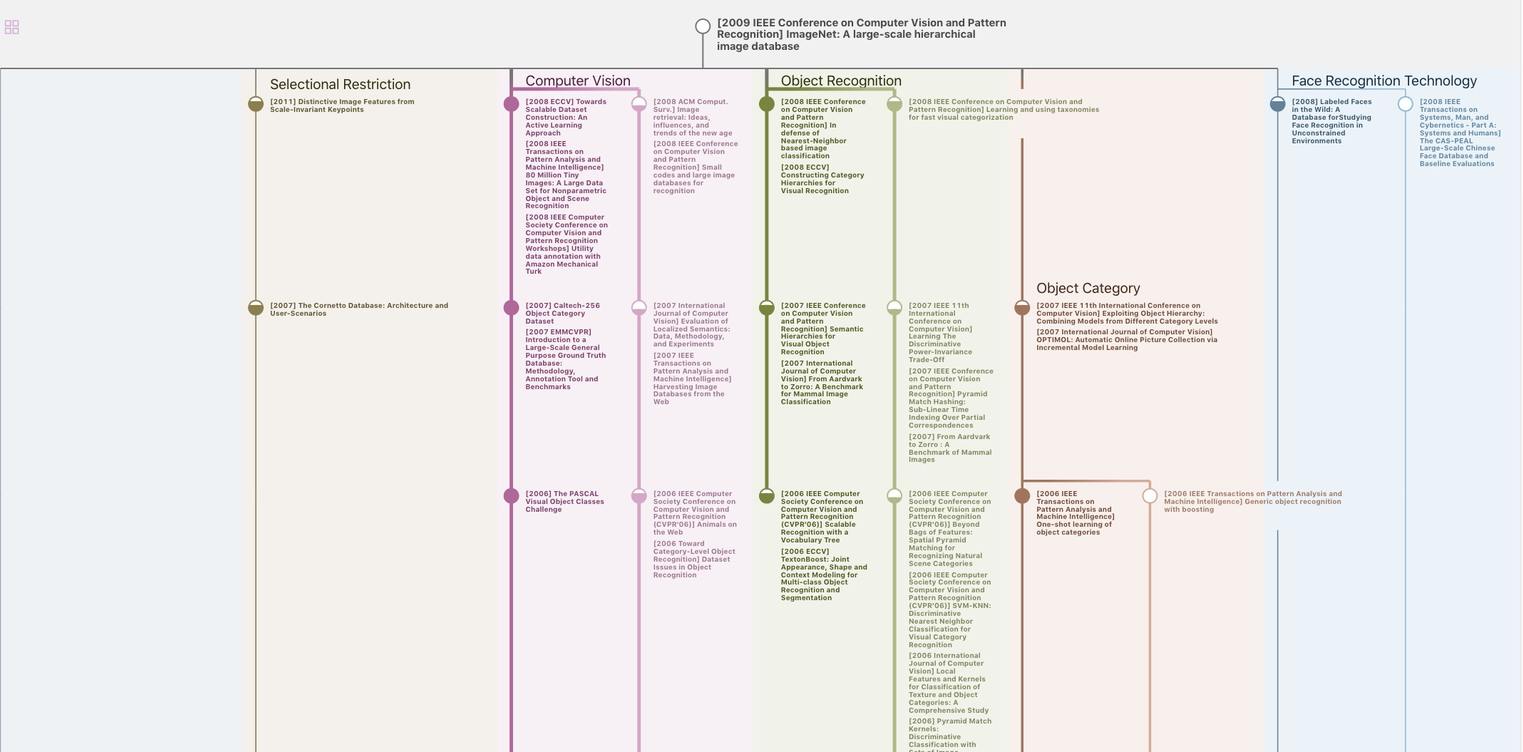
生成溯源树,研究论文发展脉络
Chat Paper
正在生成论文摘要