Reinforcement Learning for Traffic Signal Control in Hybrid Action Space
IEEE TRANSACTIONS ON INTELLIGENT TRANSPORTATION SYSTEMS(2024)
摘要
The prevailing reinforcement-learning-based traffic signal control methods are typically staging-optimizable or duration-optimizable, depending on the action spaces. In this paper, we use hybrid proximal policy optimization to synchronously optimize the stage specification and green interval duration. Under reformulated traffic demands, the intrinsic imperfections of (implementing optimization in) discrete or continuous action spaces are revealed. By comparison, hybrid action space offers a unified search space, in which our proposed method is able to better balance the trade-off between frequent switching and unsaturated release. Experiments in both single-agent and multi-agent scenarios are given to demonstrate that the proposed method reduces queue length and delay by an average of 12.72% and 11.89%, compared to the state-of-the-art RL methods. Furthermore, by calculating the Gini coefficients of right-of-way, we reveal that the proposed method does not harm fairness while improving efficiency.
更多查看译文
关键词
Traffic signal control,deep reinforcement learning,proximal policy optimization,hybrid action space
AI 理解论文
溯源树
样例
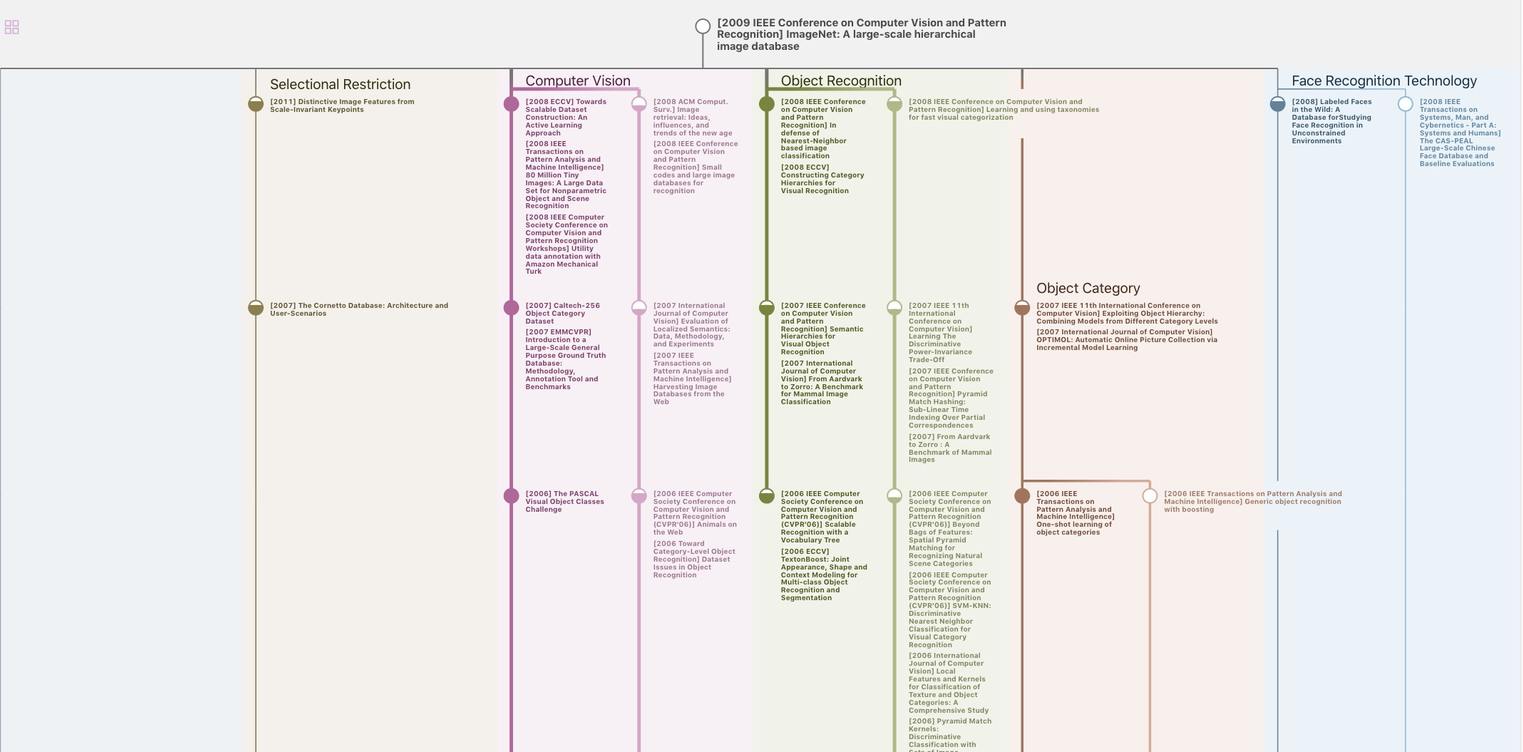
生成溯源树,研究论文发展脉络
Chat Paper
正在生成论文摘要