MECCH: Metapath Context Convolution-based Heterogeneous Graph Neural Networks
NEURAL NETWORKS(2024)
摘要
Heterogeneous graph neural networks (HGNNs) were proposed for representation learning on structural data with multiple types of nodes and edges. To deal with the performance degradation issue when HGNNs become deep, researchers combine metapaths into HGNNs to associate nodes closely related in semantics but far apart in the graph. However, existing metapath-based models suffer from either information loss or high computation costs. To address these problems, we present a novel Metapath Context Convolution-based Heterogeneous Graph Neural Network (MECCH). MECCH leverages metapath contexts, a new kind of graph structure that facilitates lossless node information aggregation while avoiding any redundancy. Specifically, MECCH applies three novel components after feature preprocessing to extract comprehensive information from the input graph efficiently: (1) metapath context construction, (2) metapath context encoder, and (3) convolutional metapath fusion. Experiments on five real-world heterogeneous graph datasets for node classification and link prediction show that MECCH achieves superior prediction accuracy compared with state-of-the-art baselines with improved computational efficiency. The code is available at https://github.com/cynricfu/MECCH.
更多查看译文
关键词
Graph neural networks,Heterogeneous information networks,Graph representation learning
AI 理解论文
溯源树
样例
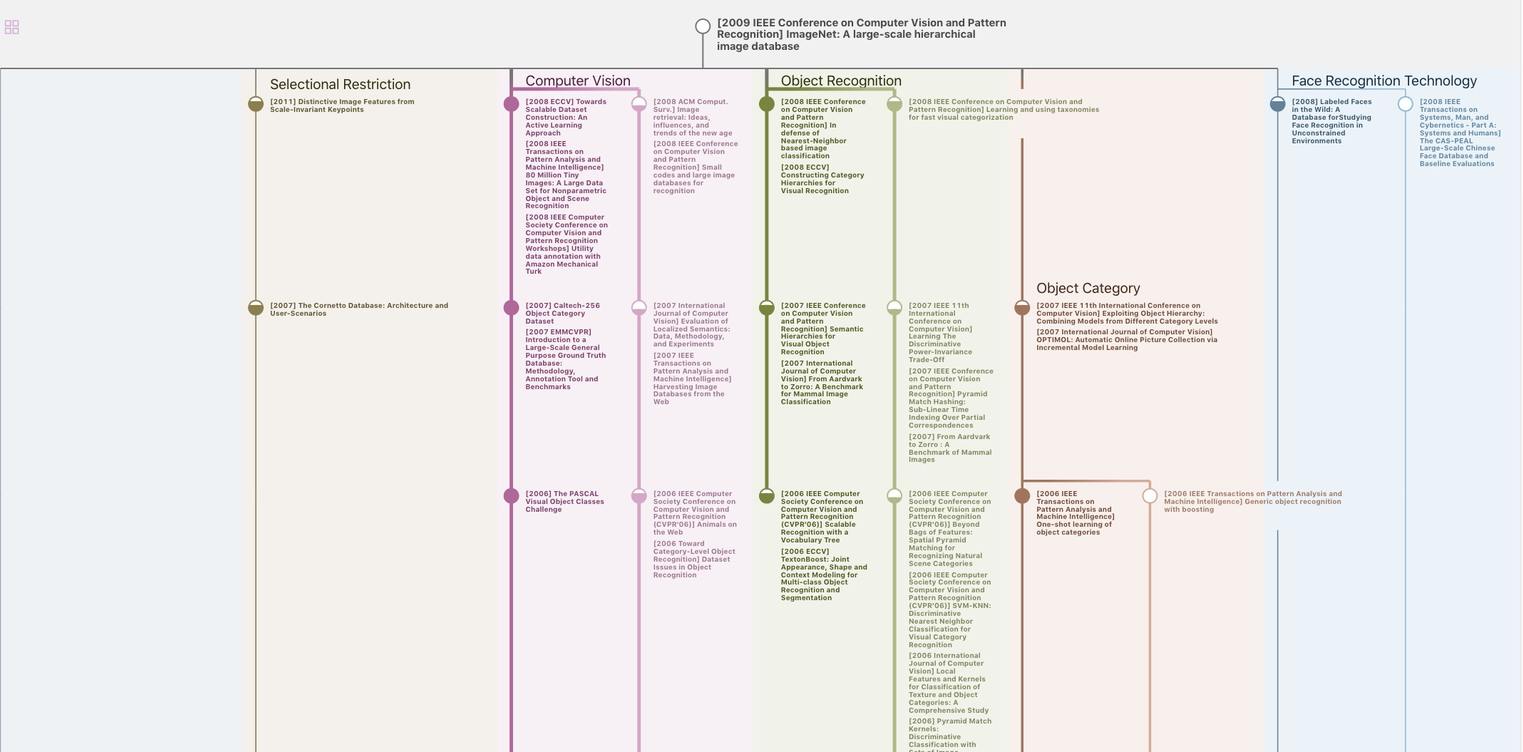
生成溯源树,研究论文发展脉络
Chat Paper
正在生成论文摘要