Neural Network Optimization with Biologically Inspired Low-Dimensional Manifold Learning
2021 International Conference on Computational Science and Computational Intelligence (CSCI)(2021)
摘要
Neural Networks learn to recognize and leverage patterns in data. In most cases, while data is represented in a high-dimensional space, the patterns within the data exist along a manifold in a small subset of those dimensions. In this paper, we show that by using a biologically inspired algorithm called Geometric Multi-Resolution Analysis (GMRA), these low-dimensional manifolds can be computed and can be used to convert datasets into more useful forms for learning. We also show that, thanks to the lower-dimensional representation of the converted datasets, that smaller networks can achieve state-of-the-art performance while using significantly fewer parameters.
更多查看译文
关键词
manifold learning,GMRA,shallow network
AI 理解论文
溯源树
样例
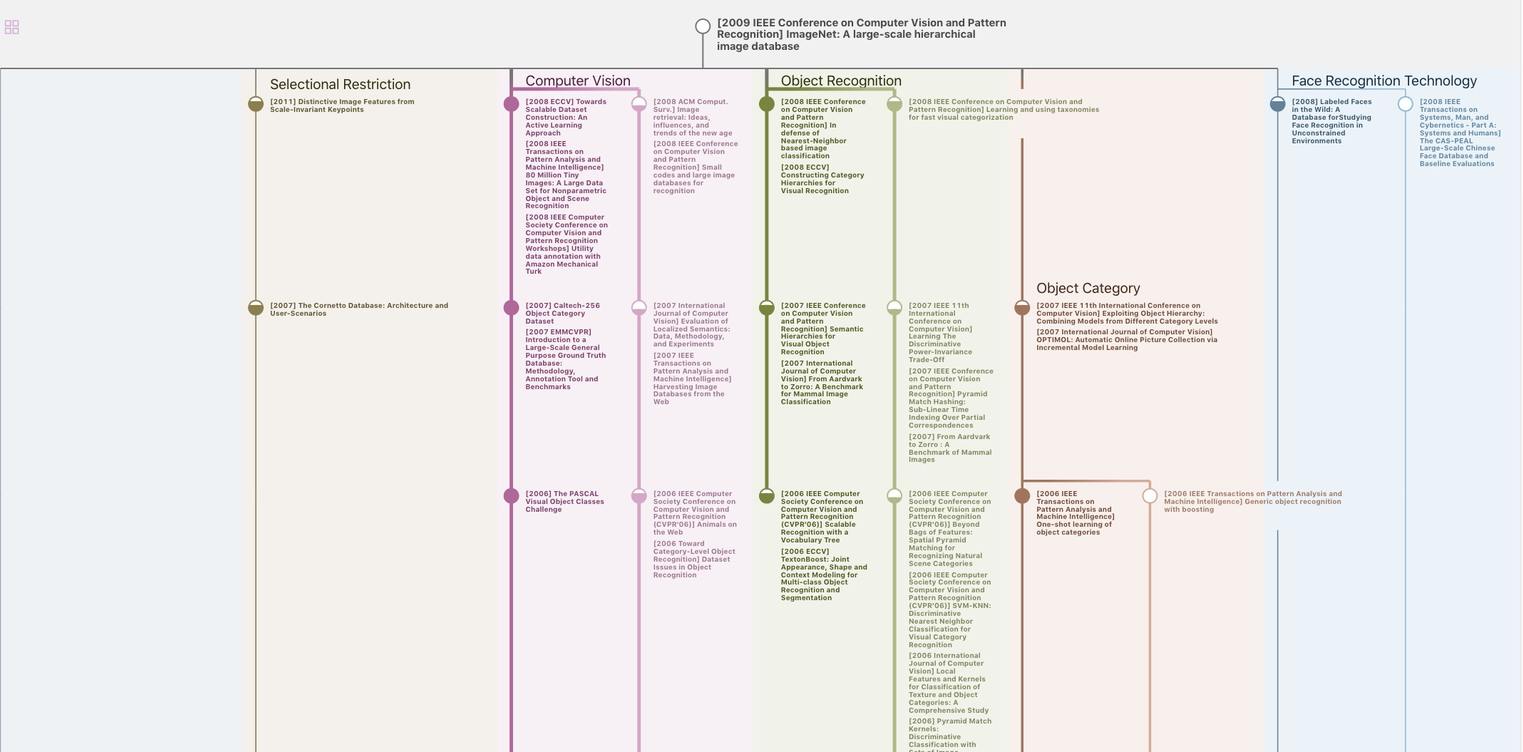
生成溯源树,研究论文发展脉络
Chat Paper
正在生成论文摘要