Multi-Sequence Dilated Network for Object Detection
2022 IEEE 24TH INTERNATIONAL WORKSHOP ON MULTIMEDIA SIGNAL PROCESSING (MMSP)(2022)
摘要
Scale variation is one of the key challenges in the object detection. Most previous object detectors remedy this by using dilated convolution to enlarge the receptive fields of the vanilla convolutional layers. However, these methods focus on either the spatial information of small objects or the semantics of middle and large objects, which still fail to effectively adapt the scale variance of different objects, resulting in a sub-optimal performance for the object detection. In this paper, we propose a novel Multi-Sequence Dilated Network (MSDN) that stacks different dilated convolutions with different orders in parallel for improving the performance of the object detection. Concretely, MSDN contains a sequential dilated module and a dilated attention module. The former aims to generate scale-specific feature maps with fine-spatial and semantic information of objects at different scales, while the latter further selects more powerful information to adaptively enlarge the receptive fields of object features at different scales. Facilitated with these modules, MSDN well obtains the fine-spatial and semantic information of objects at different scales, thus solving the problem of the scale variation. Comprehensive experimental results over two public object detection benchmarks clearly demonstrate the effectiveness of our proposed MSDN. Particularly, on the COCO dataset, the mAP value of MSDN is 48.7%, outperforming existing state-of-the-art methods in a single model manner.
更多查看译文
关键词
scale variation,dilated convolution,object detection,FPN
AI 理解论文
溯源树
样例
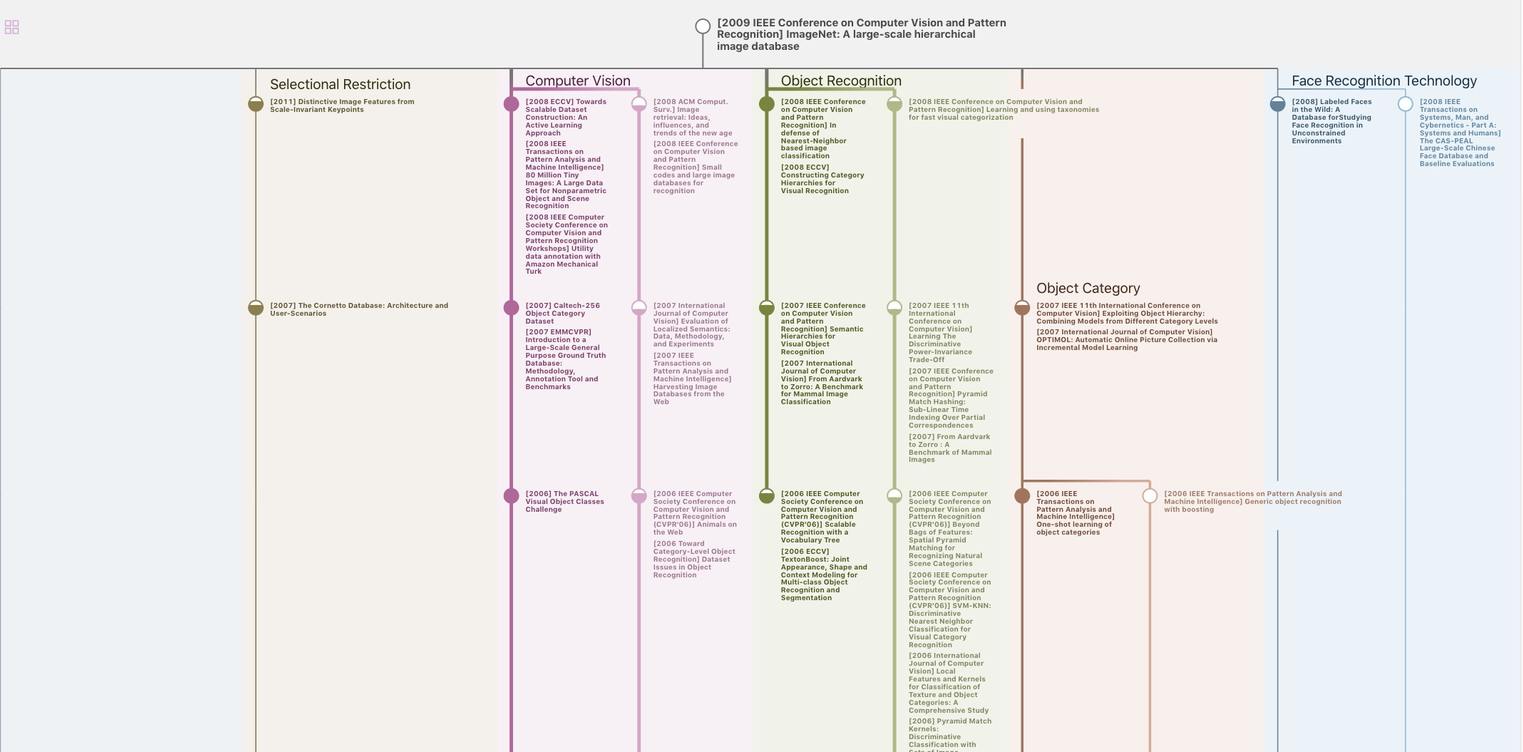
生成溯源树,研究论文发展脉络
Chat Paper
正在生成论文摘要