Short-Term Time Series Forecasting based on Edge Machine Learning Techniques for IoT devices
2022 7th International Conference on Smart and Sustainable Technologies (SpliTech)(2022)
摘要
As the effects of climate change are becoming more and more evident, the importance of improved situation awareness is also gaining more attention, both in the context of preventive environmental monitoring and in the context of acute crisis response. One important aspect of situation awareness is the correct and thorough monitoring of air pollutants. The monitoring is threatened by sensor faults, power or network failures, or other hazards leading to missing or incorrect data transmission. For this reason, in this work we propose two complementary approaches for predicting missing sensor data and a combined technique for detecting outliers. The proposed solution can enhance the performance of low-cost sensor systems, closing the gap of missing measurements due to network unavailability, detecting drift and outliers thus paving the way to its use as an alert system for reportable events. The techniques have been deployed and tested also in a low power microcontroller environment, verifying the suitability of such a computing power to perform the inference locally, leading the way to an edge implementation of a virtual sensor digital twin.
更多查看译文
关键词
LSTM,Random Forest,Machine learning,Environmental data
AI 理解论文
溯源树
样例
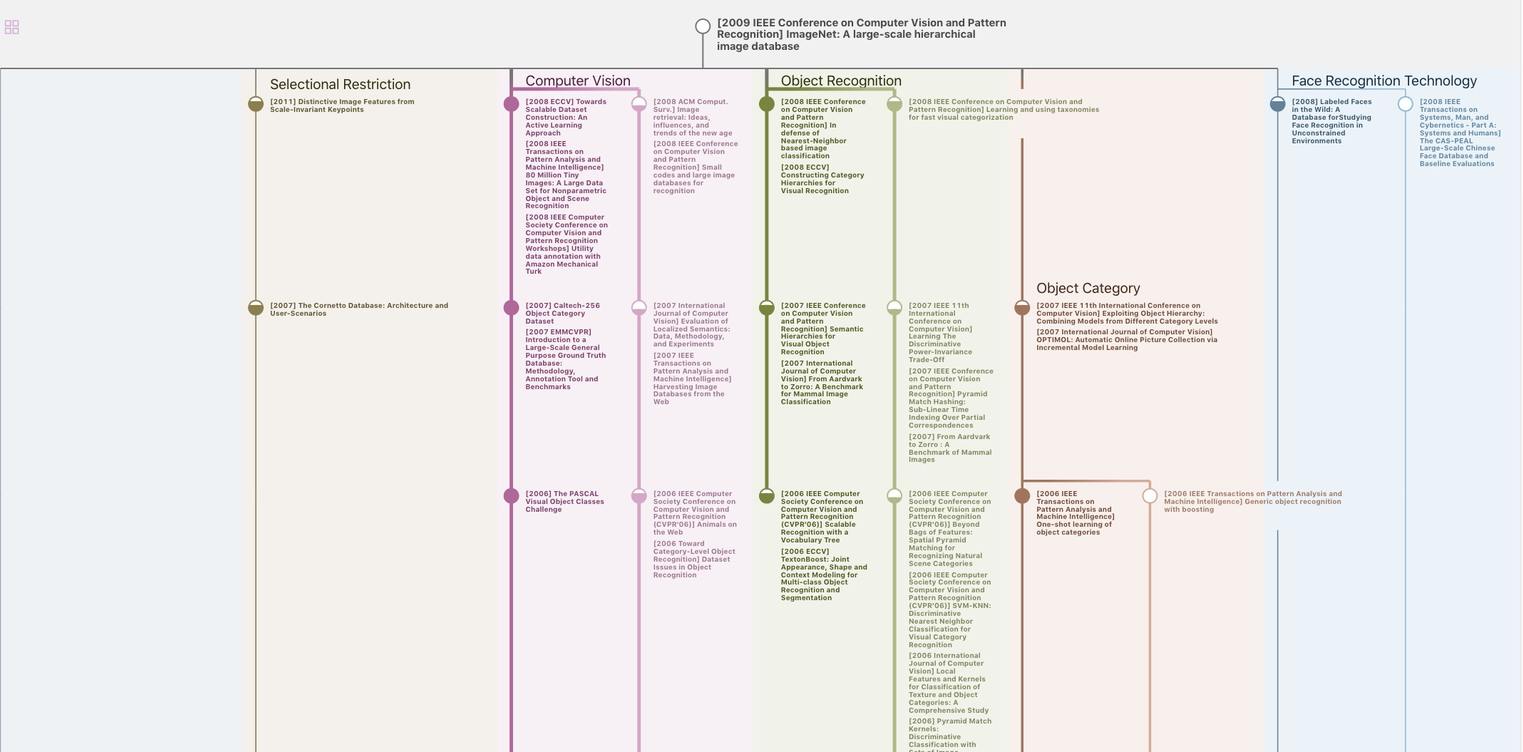
生成溯源树,研究论文发展脉络
Chat Paper
正在生成论文摘要