scFormer: A Universal Representation Learning Approach for Single-Cell Data Using Transformers
biorxiv(2022)
摘要
Single-cell sequencing has emerged as a promising technique to decode cellular heterogeneity and analyze gene functions. With the high throughput of modern techniques and resulting large-scale sequencing data, deep learning has been used extensively to learn representations of individual cells for downstream tasks. However, most existing methods rely on fully connected networks and are unable to model complex relationships between both cell and gene representations. We hereby propose scFormer, a novel transformer-based deep learning framework to jointly optimize cell and gene embeddings for single-cell biology in an unsupervised manner. By drawing parallels between natural language processing and genomics, scFormer applies self-attention to learn salient gene and cell embeddings through masked gene modelling. scFormer provides a unified framework to readily address a variety of downstream tasks such as data integration, analysis of gene function, and perturbation response prediction. Extensive experiments using scFormer show state-of-the-art performance on seven datasets across the relevant tasks. The scFormer model implementation is available at https://github.com/bowang-lab/scFormer.
### Competing Interest Statement
The authors have declared no competing interest.
更多查看译文
关键词
universal representation learning approach,transformers,single-cell
AI 理解论文
溯源树
样例
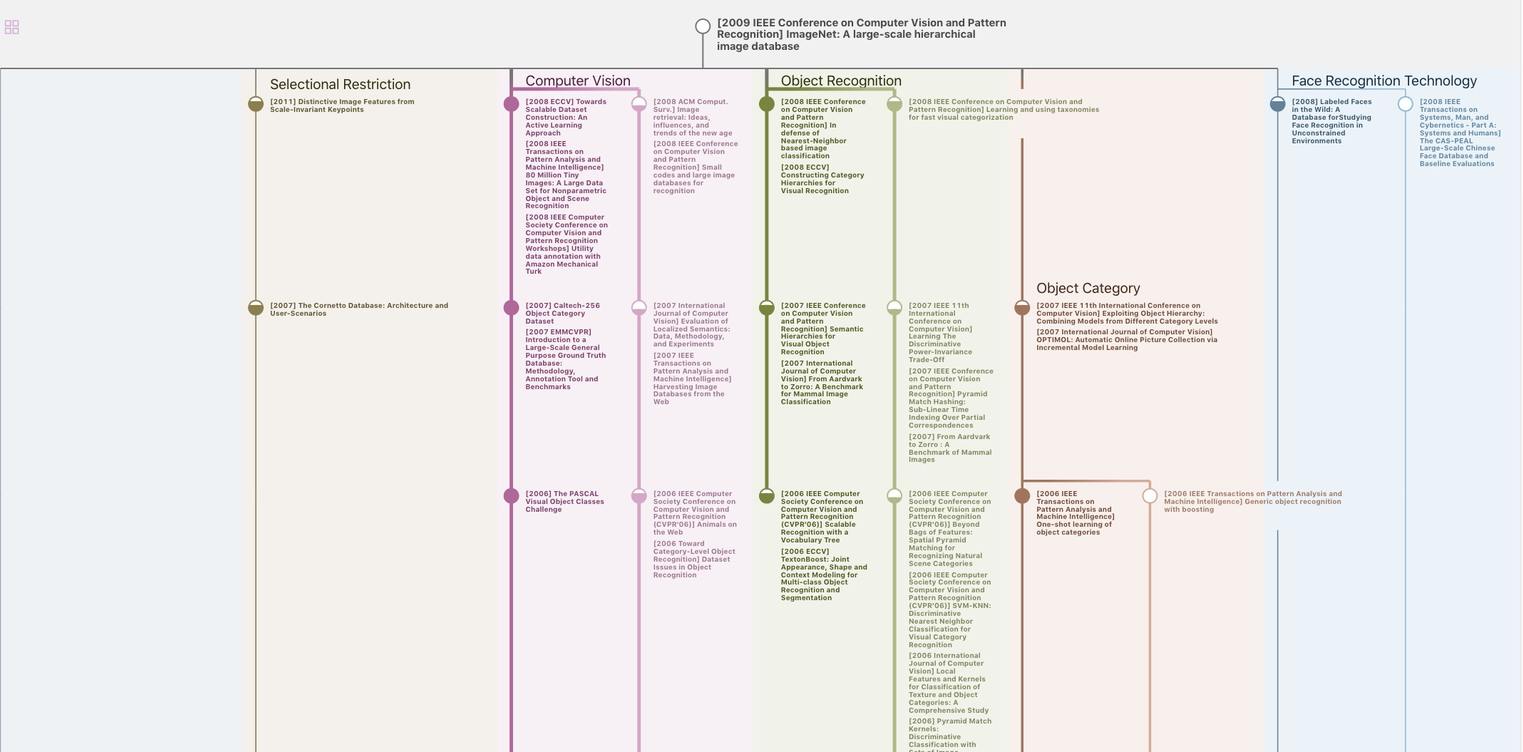
生成溯源树,研究论文发展脉络
Chat Paper
正在生成论文摘要