Leveraging memory effects and gradient information in consensus-based optimisation: On global convergence in mean-field law
arxiv(2023)
摘要
In this paper, we study consensus-based optimisation (CBO), a versatile, flexible and customisable optimisation method suitable for performing nonconvex and nonsmooth global optimisations in high dimensions. CBO is a multi-particle metaheuristic, which is effective in various applications and at the same time amenable to theoretical analysis thanks to its minimalistic design. The underlying dynamics, however, is flexible enough to incorporate different mechanisms widely used in evolutionary computation and machine learning, as we show by analysing a variant of CBO which makes use of memory effects and gradient information. We rigorously prove that this dynamics converges to a global minimiser of the objective function in mean-field law for a vast class of functions under minimal assumptions on the initialisation of the method. The proof in particular reveals how to leverage further, in some applications advantageous, forces in the dynamics without loosing provable global convergence. To demonstrate the benefit of the herein investigated memory effects and gradient information in certain applications, we present numerical evidence for the superiority of this CBO variant in applications such as machine learning and compressed sensing, which en passant widen the scope of applications of CBO.
更多查看译文
关键词
High-dimensional global optimisation,metaheuristics,consensus-based optimisation,mean-field limit,Fokker-Planck equations
AI 理解论文
溯源树
样例
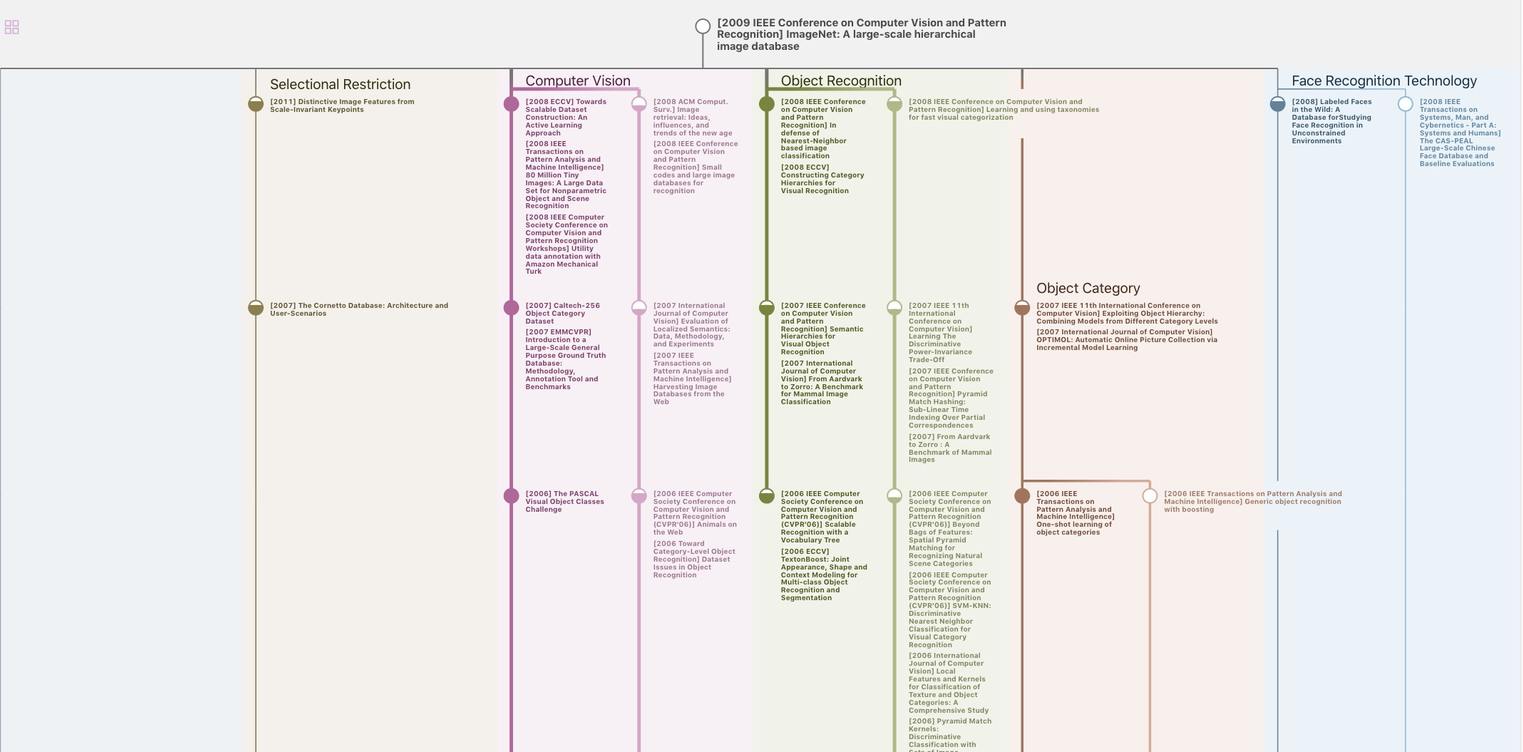
生成溯源树,研究论文发展脉络
Chat Paper
正在生成论文摘要