Learnable Spectral Wavelets on Dynamic Graphs to Capture Global Interactions
arxiv(2022)
摘要
Learning on evolving(dynamic) graphs has caught the attention of researchers as static methods exhibit limited performance in this setting. The existing methods for dynamic graphs learn spatial features by local neighborhood aggregation, which essentially only captures the low pass signals and local interactions. In this work, we go beyond current approaches to incorporate global features for effectively learning representations of a dynamically evolving graph. We propose to do so by capturing the spectrum of the dynamic graph. Since static methods to learn the graph spectrum would not consider the history of the evolution of the spectrum as the graph evolves with time, we propose a novel approach to learn the graph wavelets to capture this evolving spectra. Further, we propose a framework that integrates the dynamically captured spectra in the form of these learnable wavelets into spatial features for incorporating local and global interactions. Experiments on eight standard datasets show that our method significantly outperforms related methods on various tasks for dynamic graphs.
更多查看译文
关键词
learnable spectral wavelets,dynamic graphs,interactions
AI 理解论文
溯源树
样例
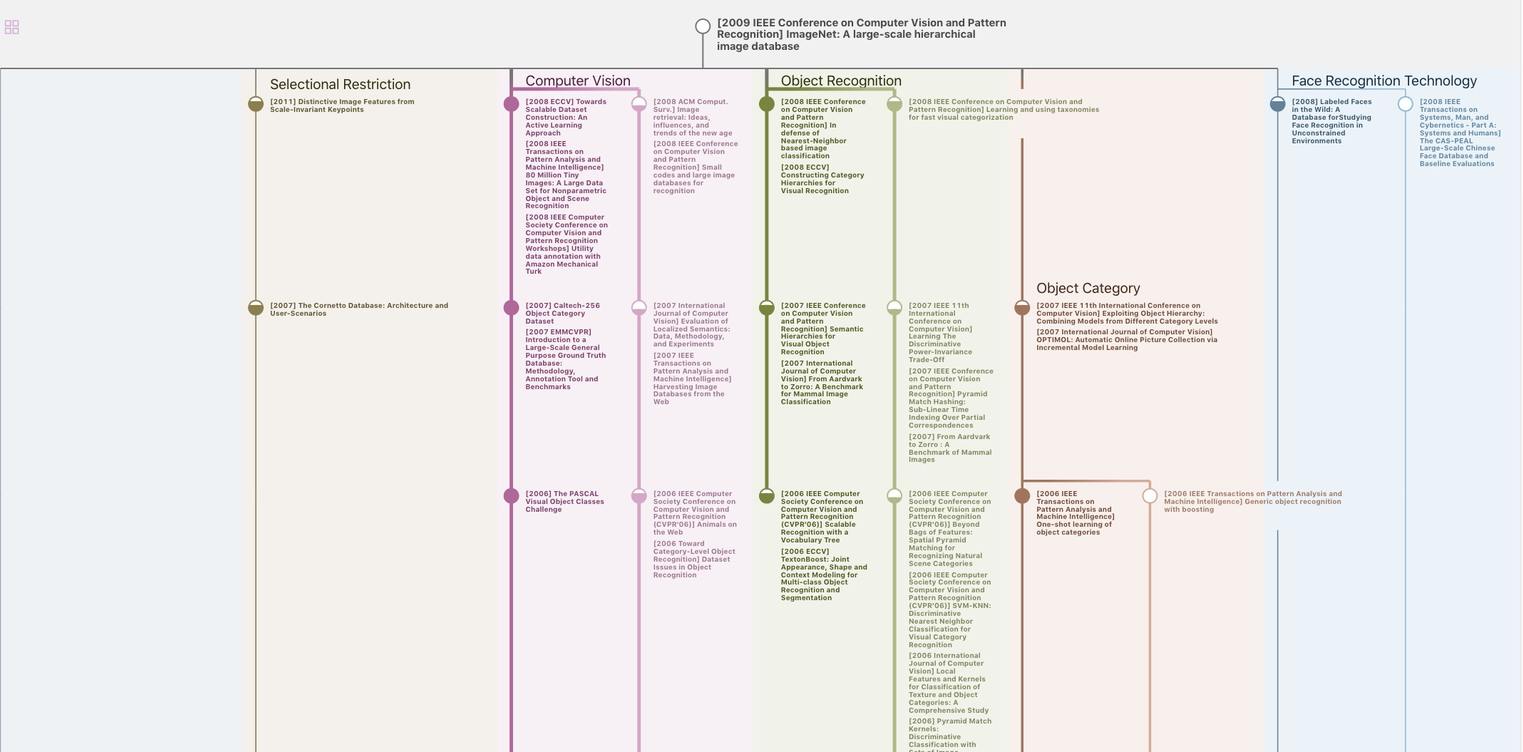
生成溯源树,研究论文发展脉络
Chat Paper
正在生成论文摘要