Model-Independent Learning of Quantum Phases of Matter with Quantum Convolutional Neural Networks
arxiv(2023)
摘要
Quantum convolutional neural networks (QCNNs) have been introduced as classifiers for gapped quantum phases of matter. Here, we propose a model-independent protocol for training QCNNs to discover order parameters that are unchanged under phase-preserving perturbations. We initiate the training sequence with the fixed-point wave functions of the quantum phase and add translation-invariant noise that respects the symmetries of the system to mask the fixed-point structure on short length scales. We illustrate this approach by training the QCNN on phases protected by time-reversal symmetry in one dimension, and test it on several time-reversal symmetric models exhibiting trivial, symmetry-breaking, and symmetryprotected topological order. The QCNN discovers a set of order parameters that identifies all three phases and accurately predicts the location of the phase boundary. The proposed protocol paves the way toward hardware-efficient training of quantum phase classifiers on a programmable quantum processor.
更多查看译文
关键词
quantum convolutional neural networks,quantum phases,convolutional neural networks,model-independent
AI 理解论文
溯源树
样例
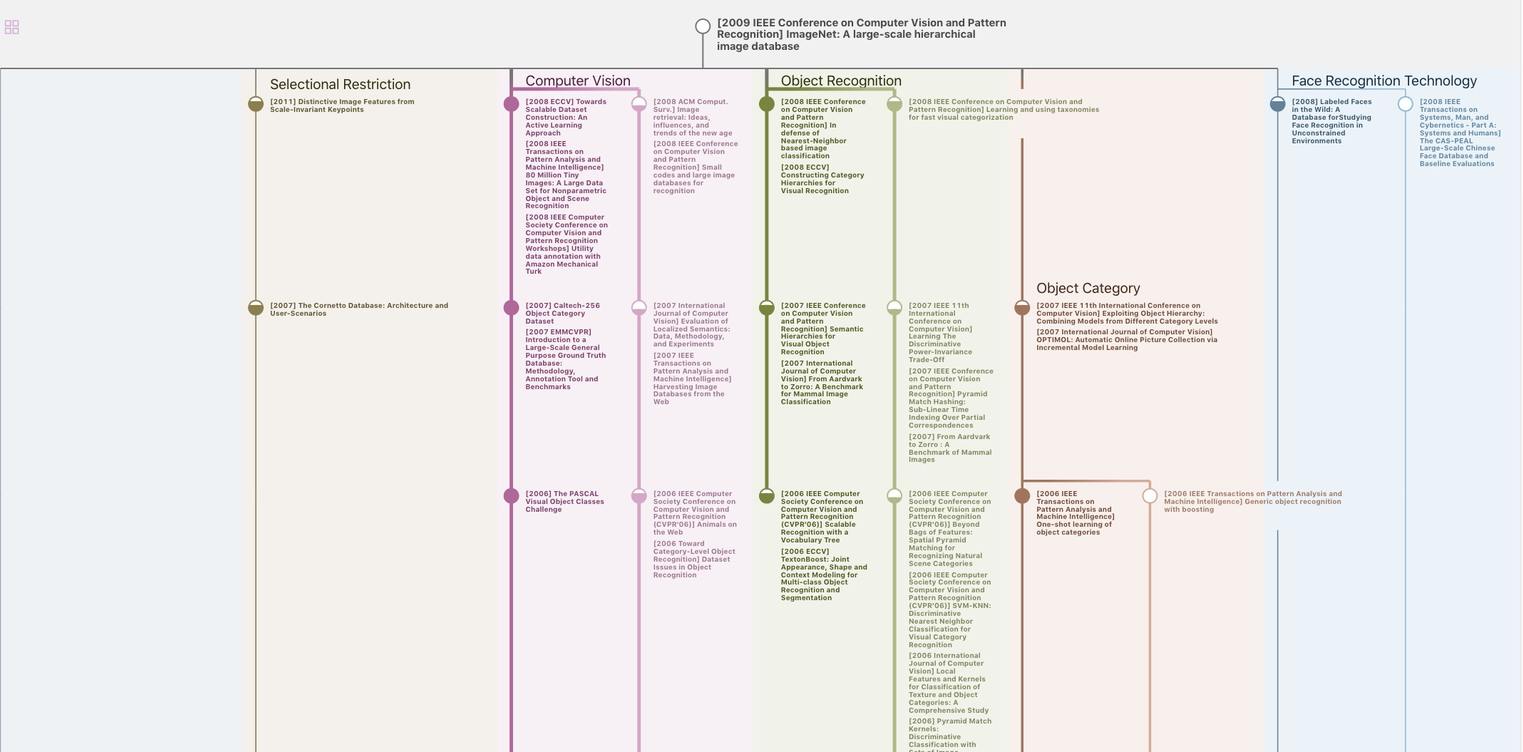
生成溯源树,研究论文发展脉络
Chat Paper
正在生成论文摘要