Performance optimizations on U-Net speech enhancement models
2022 IEEE 24th International Workshop on Multimedia Signal Processing (MMSP)(2022)
摘要
Deep learning approaches-while remarkably successful in audio enhancement-result in slower inference times which are prohibitive in real-time applications. We develop a practical strategy for compressing U-Net style deep neural network architectures. On deep noise suppression (DNS) models we achieve a state-of-the-art 7.25× inference speed up over the baseline CRUSE model, with a smooth model performance degradation. Our method is friendly to practitioners as it only requires setting a single compression parameter, while achieving non-uniform compression rates across layers. We report inference speed because a parameter or memory reduction does not necessitate speedup, and we measure model quality using an accurate non-intrusive objective speech quality metric.
更多查看译文
关键词
speech enhancement,noise reduction,real-time,inference speedup,structured pruning
AI 理解论文
溯源树
样例
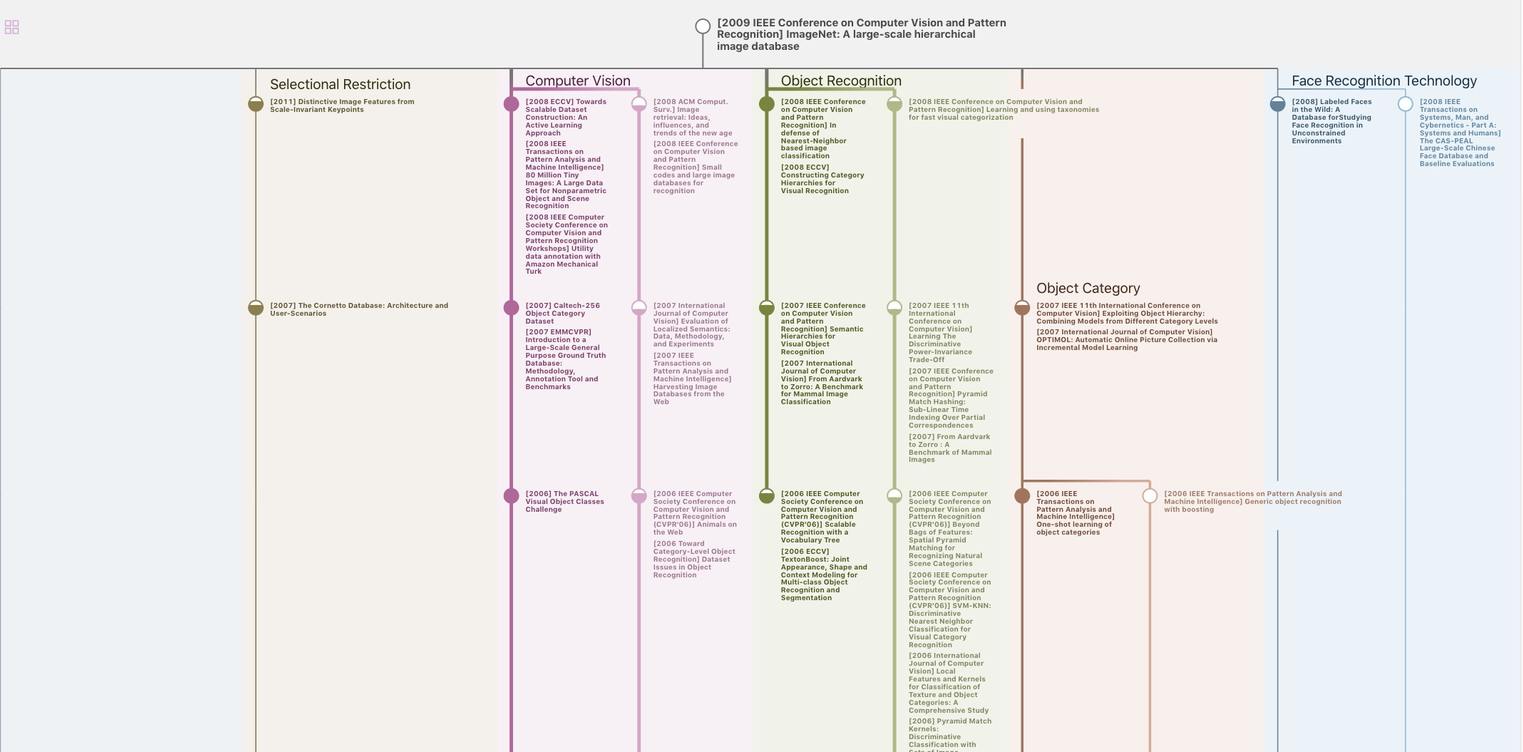
生成溯源树,研究论文发展脉络
Chat Paper
正在生成论文摘要