Light-Weight EPINET Architecture for Fast Light Field Disparity Estimation
2022 IEEE 24th International Workshop on Multimedia Signal Processing (MMSP)(2022)
摘要
Recent deep learning-based light field disparity estimation algorithms require millions of parameters, which demand high computational cost and limit the model deployment. In this paper, an investigation is carried out to analyze the effect of depthwise separable convolution and ghost modules on state-of-the-art EPINET architecture for disparity estimation. Based on this investigation, four convolutional blocks are proposed to make the EPINET architecture a fast and light-weight network for disparity estimation. The experimental results exhibit that the proposed convolutional blocks have significantly reduced the computational cost of EPINET architecture by up to a factor of 3.89, while achieving comparable disparity maps on HCI Benchmark dataset.
更多查看译文
关键词
Light Field,Deep Learning,Disparity Estimation,Compression,Depthwise Separable Convolution
AI 理解论文
溯源树
样例
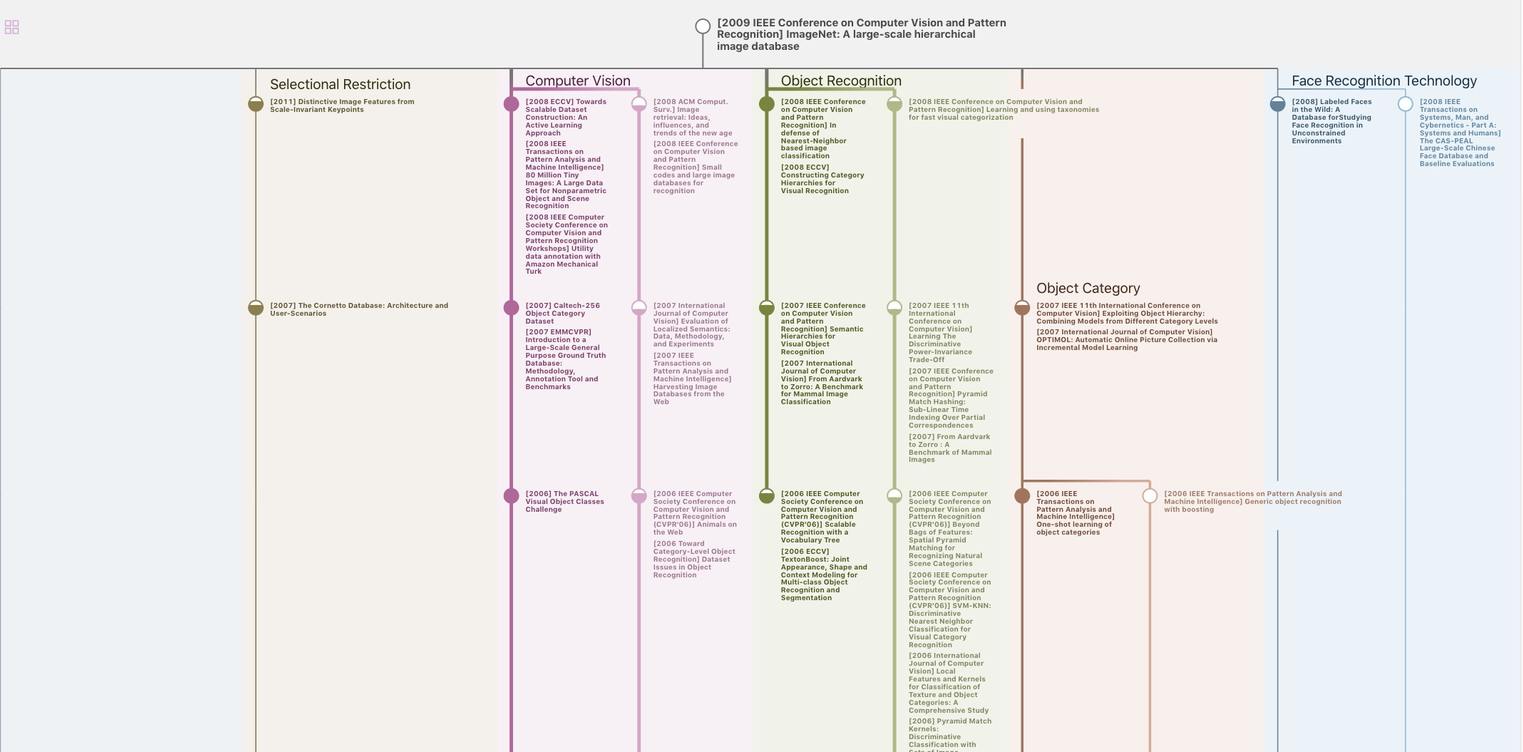
生成溯源树,研究论文发展脉络
Chat Paper
正在生成论文摘要