Comparing and combining data from immune assays based on left-censored multivariate normal model assuming common assay differences across settings.
Statistics in medicine(2023)
摘要
In vaccine research towards the prevention of infectious diseases, immune response biomarkers serve as an important tool for comparing and ranking vaccine candidates based on their immunogenicity and predicted protective effect. However, analyses of immune response outcomes can be complicated by differences across assays when immune response data are acquired from multiple groups/laboratories. Motivated by a real-world problem to accommodate the use of two different neutralization assays in COVID-19 vaccine trials, we propose methods based on left-censored multivariate normal model assuming common assay differences across settings, to adjust for differences between assays with respect to measurement error and the lower limit of detection. Our proposed methods integrate external paired-sample data with bridging assumptions to achieve two objectives, both using pooled data acquired from different assays: (i) comparing immunogenicity between vaccine regimens, and (ii) evaluating correlates of risk. In simulation studies, for the first objective, our method leads to unbiased calibrated assay mean with good coverage of bootstrap confidence interval, as well as valid test for immunogenicity comparison, while the alternative method assuming constant calibration model between assays leads to biased estimate of assay mean with undercoverage problem and invalid test with inflated type-I error; for the second objective, in the presence of noticeable left-censoring rate, our proposed method can drastically outperform the existing method that ignores left-censoring, in terms of reduced bias and improved precision. We apply the proposed methods to SARS-CoV-2 spike-pseudotyped virus neutralization assay data generated in vaccine and convalescent samples by two different laboratories.
更多查看译文
关键词
assays,bridging,correlates analysis,left-censoring,lower limit of detection,meta-analysis
AI 理解论文
溯源树
样例
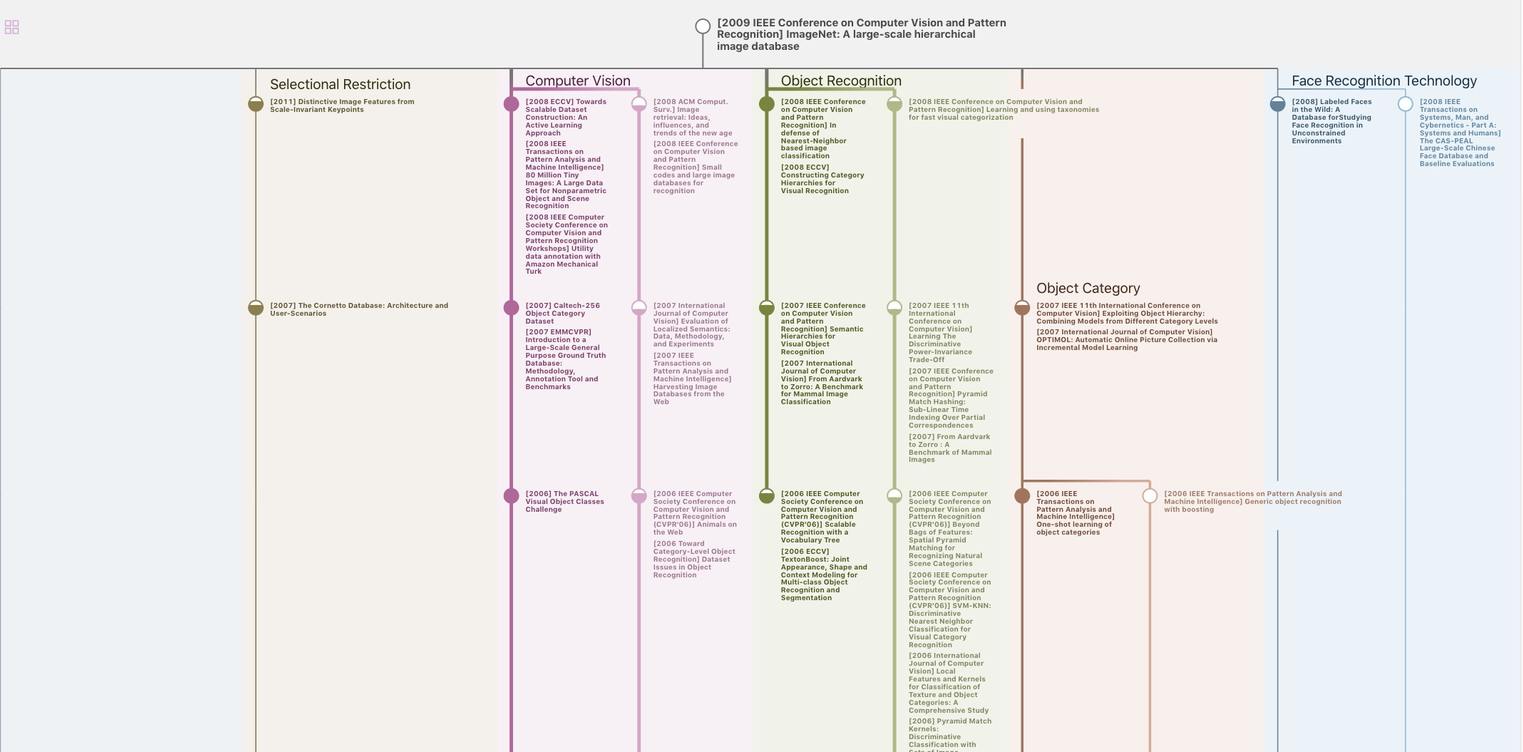
生成溯源树,研究论文发展脉络
Chat Paper
正在生成论文摘要