PERCEIVER-VL: Efficient Vision-and-Language Modeling with Iterative Latent Attention
WACV(2023)
摘要
We present PERCEIVER-VL, a vision-and-language framework that efficiently handles high-dimensional multimodal inputs such as long videos and text. Powered by the iterative latent-cross-attention of Perceiver, our framework scales with linear complexity, in contrast to the quadratic complexity of self-attention used in many state-of-the-art transformer-based models. To further improve the efficiency of our framework, we also study applying LayerDrop on cross-attention layers and introduce a mixedstream architecture for cross-modal retrieval. We evaluate PERCEIVER-VL on diverse video-text and image-text benchmarks, where PERCEIVER-VL achieves the lowest GFLOPs and latency, while maintaining competitive performance. In addition, we also provide comprehensive analyses over various aspects of our framework, including pretraining data, scalability of latent size and input size, dropping cross-attention layers at inference to reduce latency, modality aggregation strategy, positional encoding, and weight initialization strategy.(1)
更多查看译文
关键词
iterative latent attention,vision-and-language
AI 理解论文
溯源树
样例
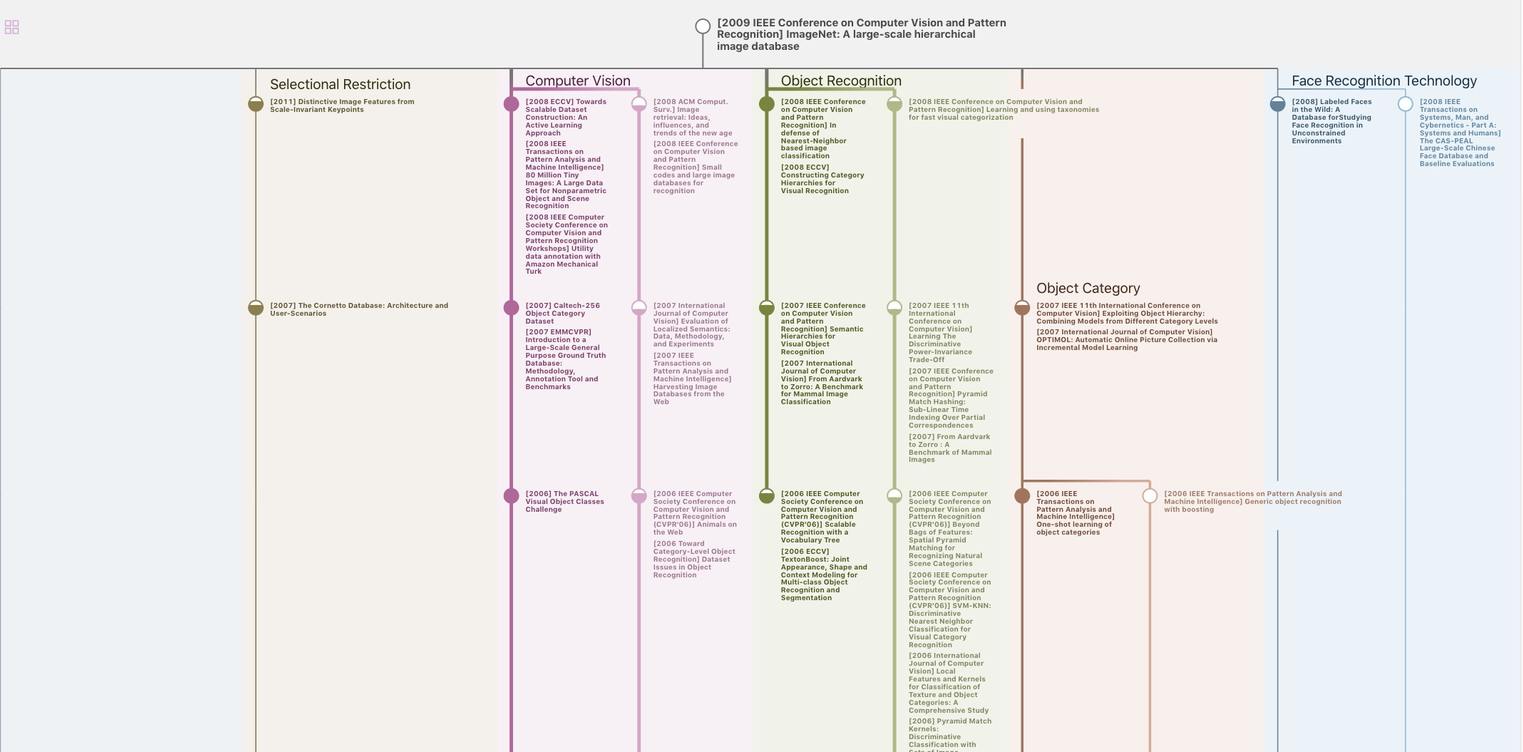
生成溯源树,研究论文发展脉络
Chat Paper
正在生成论文摘要