Forecasting Unobserved Node States with spatio-temporal Graph Neural Networks
2022 IEEE International Conference on Data Mining Workshops (ICDMW)(2022)
摘要
Forecasting future states of sensors is key to solving tasks like weather prediction, route planning, and many others when dealing with networks of sensors. But complete spatial coverage of sensors is generally unavailable and would practically be infeasible due to limitations in budget and other resources during deployment and maintenance. Currently existing approaches using machine learning are limited to the spatial locations where data was observed, causing limitations to downstream tasks. Inspired by the recent surge of Graph Neural Networks for spatio-temporal data processing, we investigate whether these can also forecast the state of locations with no sensors available. For this purpose, we develop a framework, named Forecasting Unobserved Node States (FUNS), that allows forecasting the state at entirely unobserved locations based on spatio-temporal correlations and the graph inductive bias. FUNS serves as a blueprint for optimizing models only on observed data and demonstrates good generalization capabilities for predicting the state at entirely unobserved locations during the testing stage. Our framework can be combined with any spatio-temporal Graph Neural Network, that exploits spatio-temporal correlations with surrounding observed locations by using the network's graph structure. Our employed model builds on a previous model by also allowing us to exploit prior knowledge about locations of interest, e.g. the road type. Our empirical evaluation of both simulated and real-world datasets demonstrates that Graph Neural Networks are well-suited for this task.
更多查看译文
关键词
graph neural networks,spatio-temporal,fore-casting,imputation
AI 理解论文
溯源树
样例
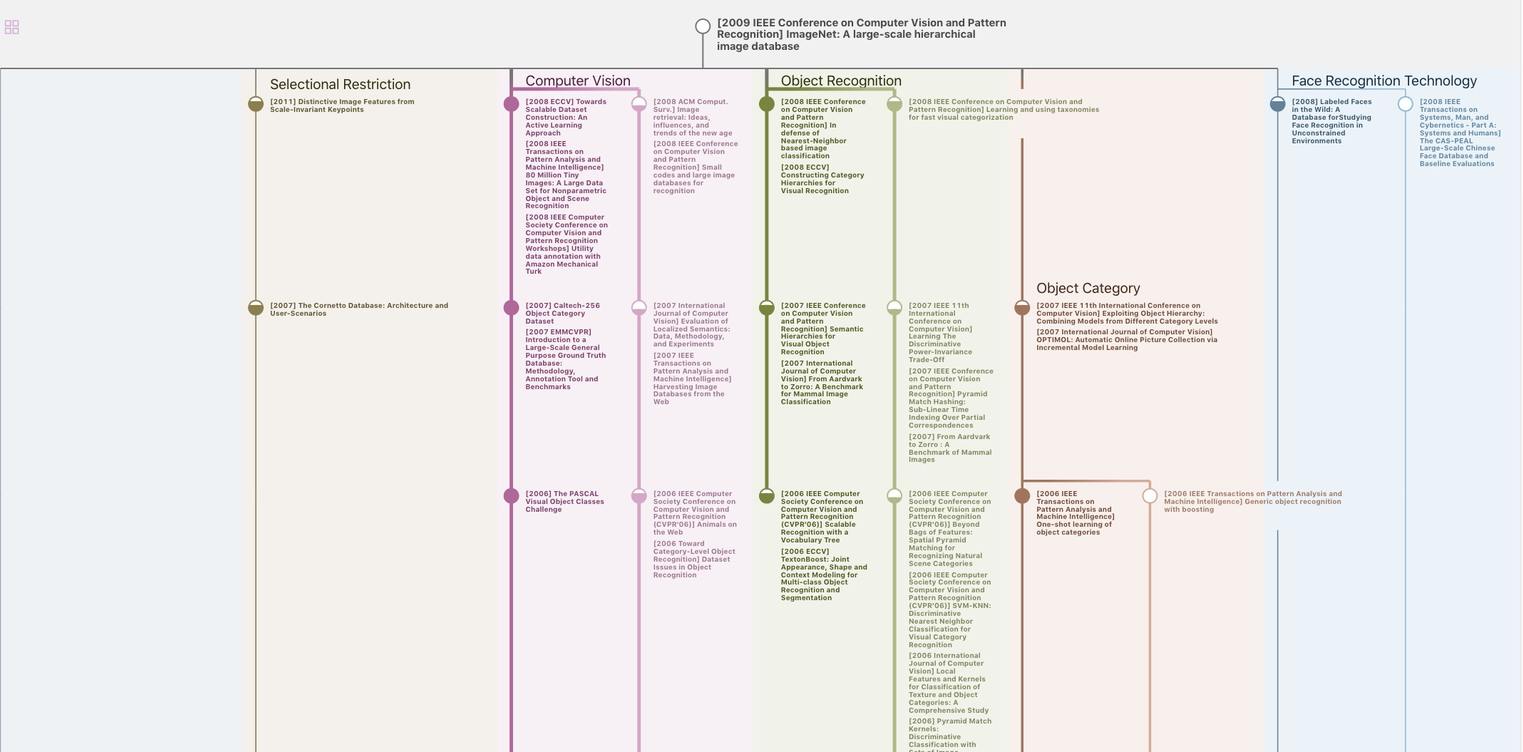
生成溯源树,研究论文发展脉络
Chat Paper
正在生成论文摘要