Distinctive Fire and Smoke Detection with Self-Similar
arxiv(2022)
摘要
Deep learning based object detection is demonstrating a preponderance in the practical artificial intelligence. However, there still are some objects that are difficult to be recognized such as fire and smoke because of their non-solid shapes. However, these objects have a mathematical fractal feature of self-similar that can relieve us from struggling with their various shapes. To this end, we propose to utilize the Hausdorff distance to evaluate the self-similarity and accordingly tailored a loss function to improve the detection accuracy of fire and smoke. Moreover, we proposed a general labeling criterion for these objects based on their geometrical features. Our experiments on commonly used baseline networks for object detection have verified that our method is valid and have improved the detecting accuracy by 2.23%.
更多查看译文
AI 理解论文
溯源树
样例
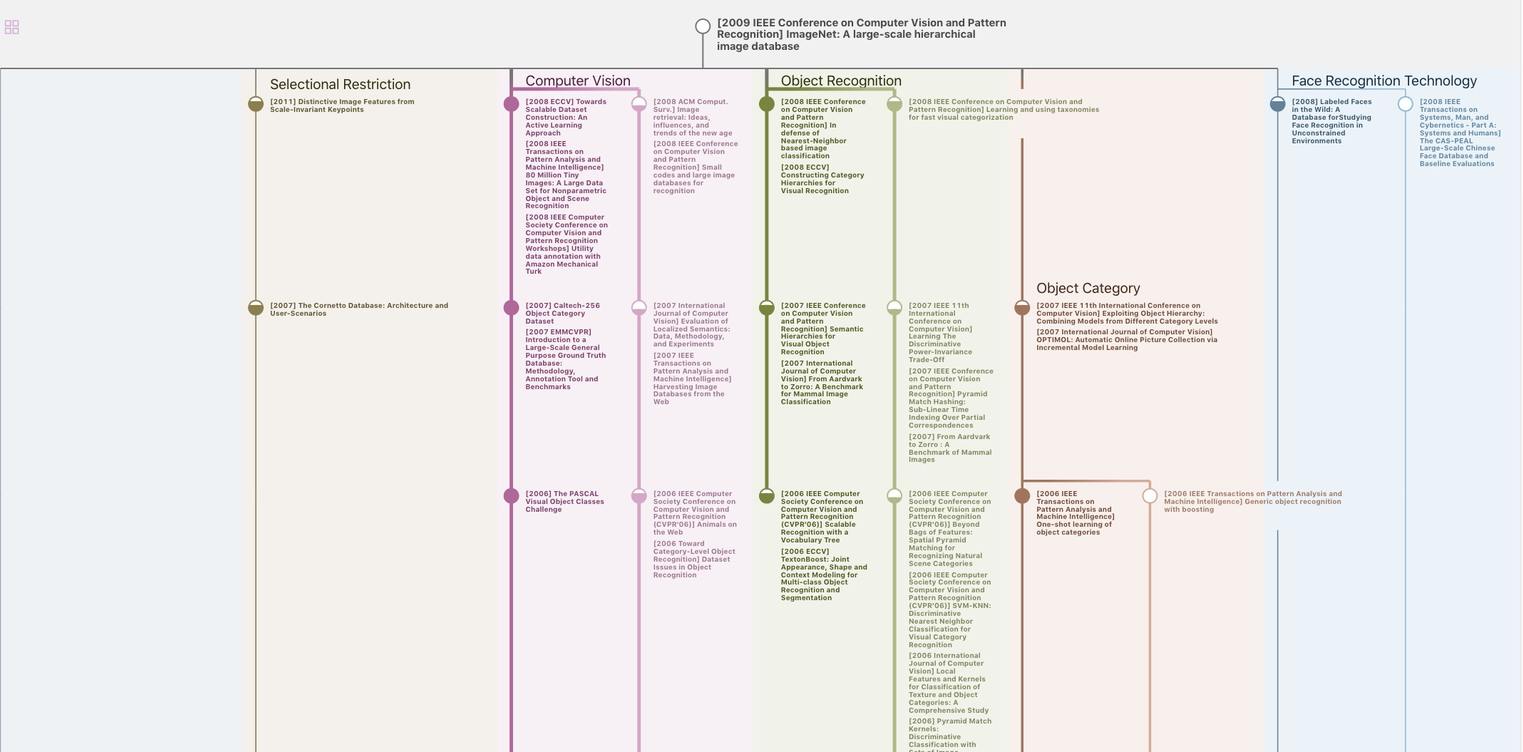
生成溯源树,研究论文发展脉络
Chat Paper
正在生成论文摘要