Unsupervised Deep Event Stereo for Depth Estimation
IEEE Transactions on Circuits and Systems for Video Technology(2022)
摘要
Bio-inspired event cameras have been considered effective alternatives to traditional frame-based cameras for stereo depth estimation, especially in challenging conditions such as low-light or high-speed environments. Recently, deep learning-based supervised event stereo matching methods have achieved significant performance improvements over the traditional event stereo methods. However, the supervised methods depend on ground-truth disparity maps for training, and it is difficult to secure a large amount of ground-truth disparity maps. A feasible alternative is to devise an unsupervised event stereo method that can be trained without ground-truth disparity maps. To this end, we propose the first unsupervised event stereo matching method that can predict dense disparity maps, and is trained by transforming the depth estimation problem into a warping-based reconstruction problem. We propose a novel unsupervised loss function that enforces the network to minimize the feature-level epipolar correlation difference between the ground-truth intensity images and warped images. Moreover, we propose a novel event embedding mechanism that utilizes both temporal and spatial neighboring events to capture spatio-temporal relationships among the events for stereo matching. Experimental results reveal that the proposed method outperforms the baseline unsupervised methods by significant margins (e.g., up to 16.88% improvement) and achieves comparable results with the existing supervised methods. Extensive ablation studies validate the efficacy of the proposed modules and architectural choices.
更多查看译文
关键词
Event camera,stereo matching,depth estimation,unsupervised deep learning
AI 理解论文
溯源树
样例
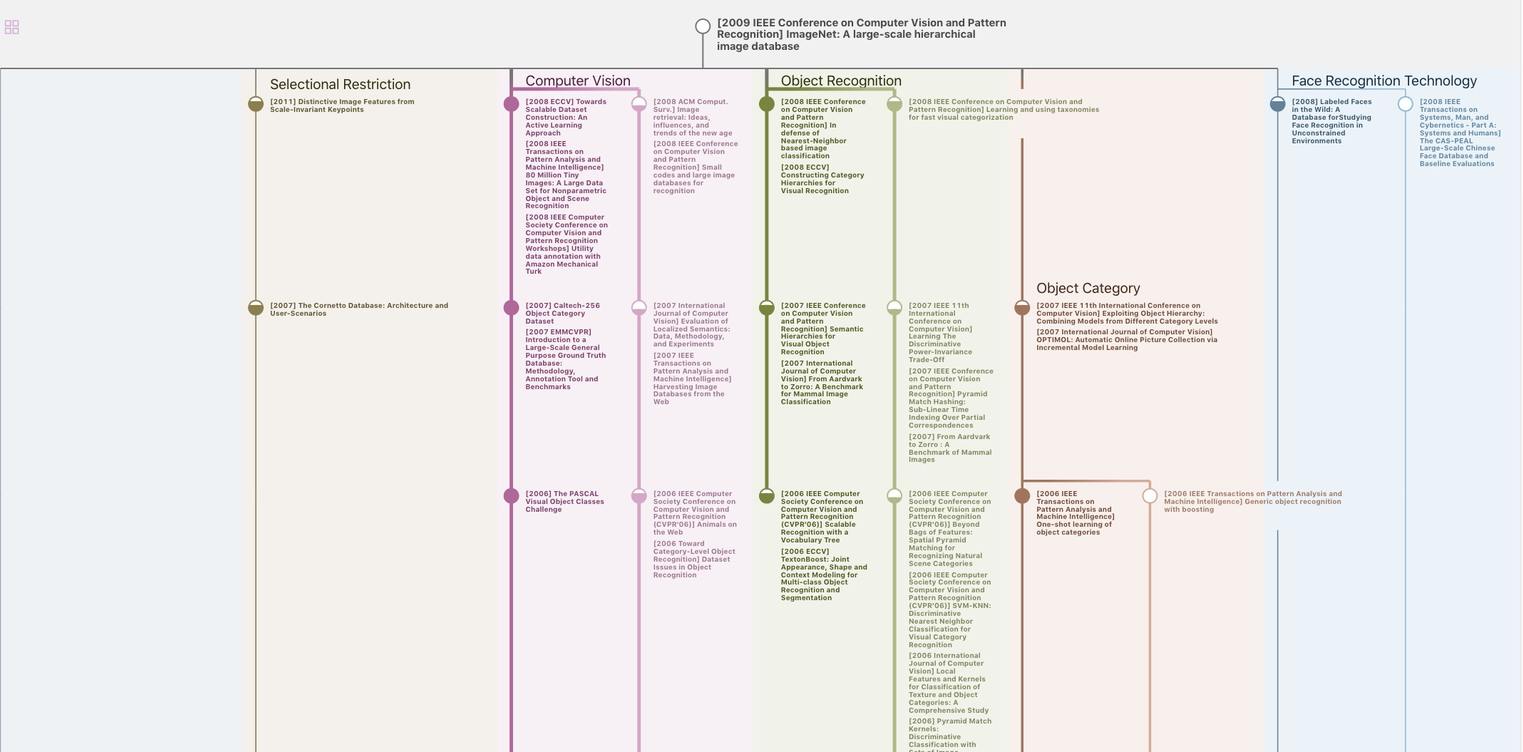
生成溯源树,研究论文发展脉络
Chat Paper
正在生成论文摘要