Addressing Parameter Uncertainty in a Health Policy Simulation Model Using Monte Carlo Sensitivity Methods
Systems(2022)
摘要
We present a practical guide and step-by-step flowchart for establishing uncertainty intervals for key model outcomes in a simulation model in the face of uncertain parameters. The process started with Powell optimization to find a set of uncertain parameters (the optimum parameter set or OPS) that minimized the model fitness error relative to historical data. Optimization also helped in refinement of parameter uncertainty ranges. Next, traditional Monte Carlo (TMC) randomization or Markov Chain Monte Carlo (MCMC) was used to create a sample of parameter sets that fit the reference behavior data nearly as well as the OPS. Under the TMC method, the entire parameter space was explored broadly with a large number of runs, and the results were sorted for selection of qualifying parameter sets (QPS) to ensure good fit and parameter distributions that were centrally located within the uncertainty ranges. In addition, the QPS outputs were graphed as sensitivity graphs or box-and-whisker plots for comparison with the historical data. Finally, alternative policies and scenarios were run against the OPS and all QPS, and uncertainty intervals were found for projected model outcomes. We illustrated the full parameter uncertainty approach with a (previously published) system dynamics model of the U.S. opioid epidemic, and demonstrated how it can enrich policy modeling results.
更多查看译文
关键词
simulation model,uncertainty analysis,optimization,sensitivity testing,Monte Carlo randomization,opioid epidemic
AI 理解论文
溯源树
样例
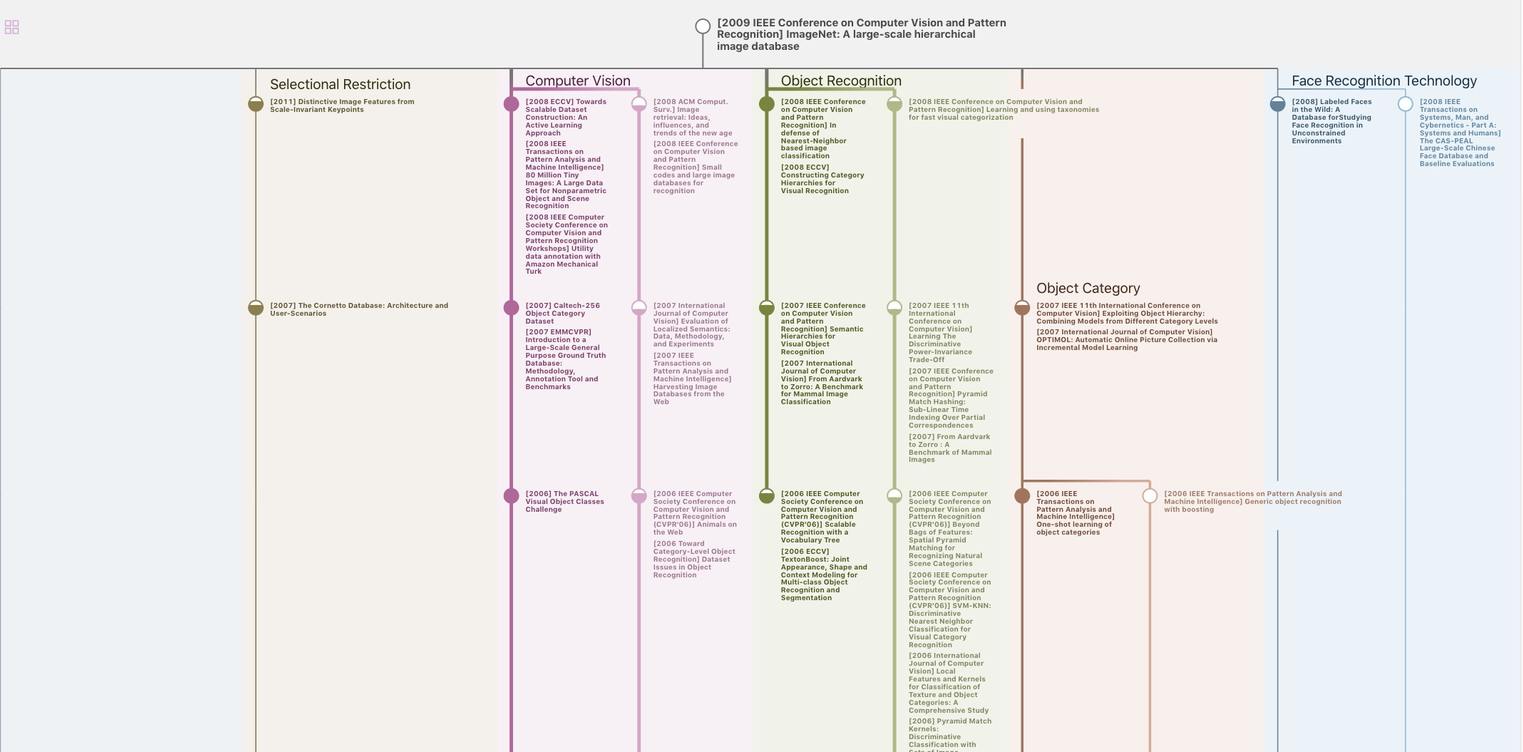
生成溯源树,研究论文发展脉络
Chat Paper
正在生成论文摘要