Automated Machine Learning in Predicting 30-Day Mortality in Patients with Non-Cholestatic Cirrhosis.
Journal of personalized medicine(2022)
摘要
The model, based on the extreme gradient boosting (XGBoost) algorithm, performed better (AUC 0.888) than the other AutoML models (logistic regression 0.673, gradient boost machine 0.886, random forest 0.866, deep learning 0.830, stacking 0.850), as well as the existing scorings (the model of end-stage liver disease [MELD] score 0.778, MELD-Na score 0.782, and albumin-bilirubin [ALBI] score 0.662). The most key variable in the XGBoost model was high-density lipoprotein cholesterol, followed by creatinine, white blood cell count, international normalized ratio, etc. Conclusion: The AutoML model based on the XGBoost algorithm presented better performance than the existing scoring systems for predicting 30-day mortality in patients with non-cholestatic cirrhosis. It shows the promise of AutoML in its future medical application.
更多查看译文
关键词
automated machine learning,local interpretable model agnostic explanation,non-cholestatic cirrhosis,partial dependence plots,shapley additive explanation
AI 理解论文
溯源树
样例
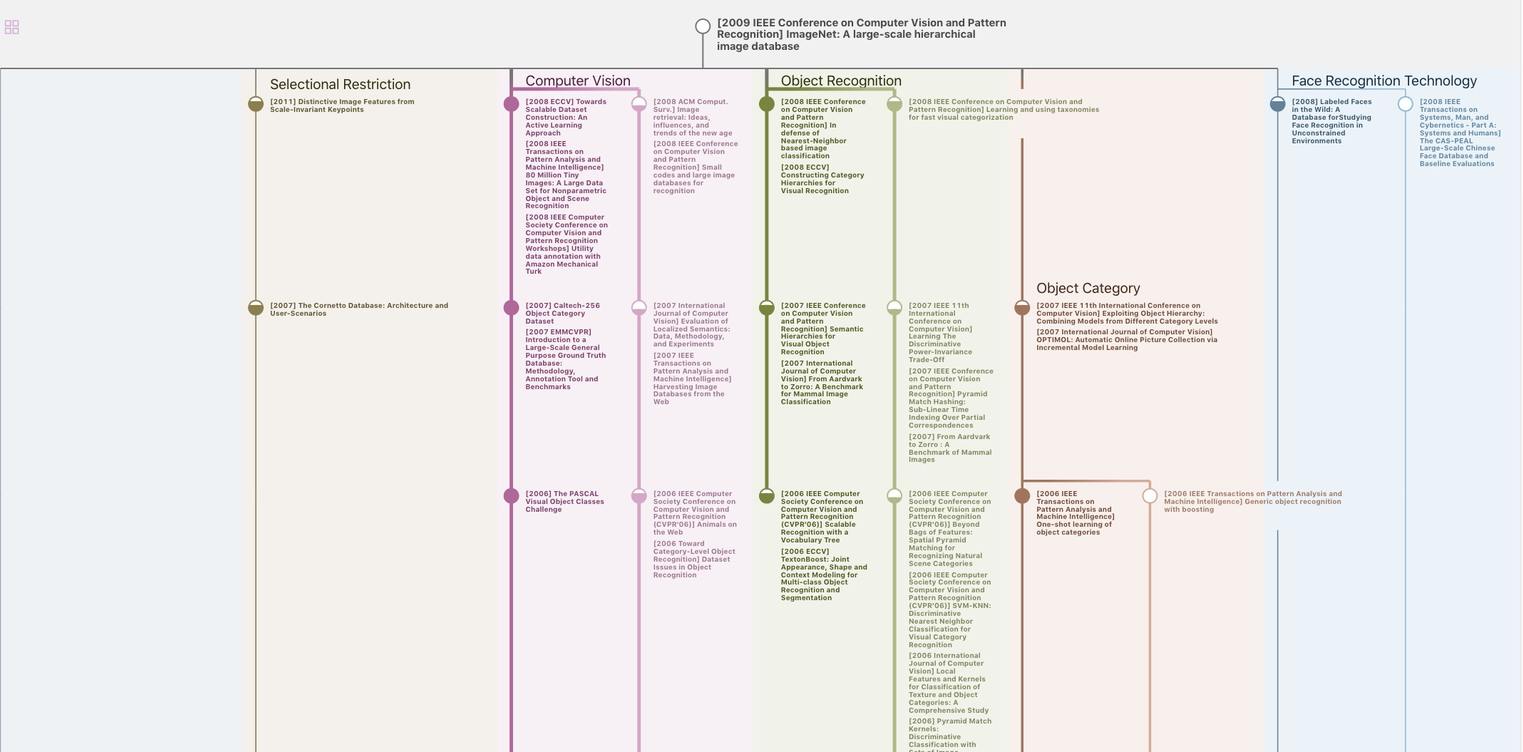
生成溯源树,研究论文发展脉络
Chat Paper
正在生成论文摘要