Just a Matter of Scale? Reevaluating Scale Equivariance in Convolutional Neural Networks
arxiv(2023)
摘要
The widespread success of convolutional neural networks may largely be attributed to their intrinsic property of translation equivariance. However, convolutions are not equivariant to variations in scale and fail to generalize to objects of different sizes. Despite recent advances in this field, it remains unclear how well current methods generalize to unobserved scales on real-world data and to what extent scale equivariance plays a role. To address this, we propose the novel Scaled and Translated Image Recognition (STIR) benchmark based on four different domains. Additionally, we introduce a new family of models that applies many re-scaled kernels with shared weights in parallel and then selects the most appropriate one. Our experimental results on STIR show that both the existing and proposed approaches can improve generalization across scales compared to standard convolutions. We also demonstrate that our family of models is able to generalize well towards larger scales and improve scale equivariance. Moreover, due to their unique design we can validate that kernel selection is consistent with input scale. Even so, none of the evaluated models maintain their performance for large differences in scale, demonstrating that a general understanding of how scale equivariance can improve generalization and robustness is still lacking.
更多查看译文
关键词
convolution,scale,equivariance,invariance
AI 理解论文
溯源树
样例
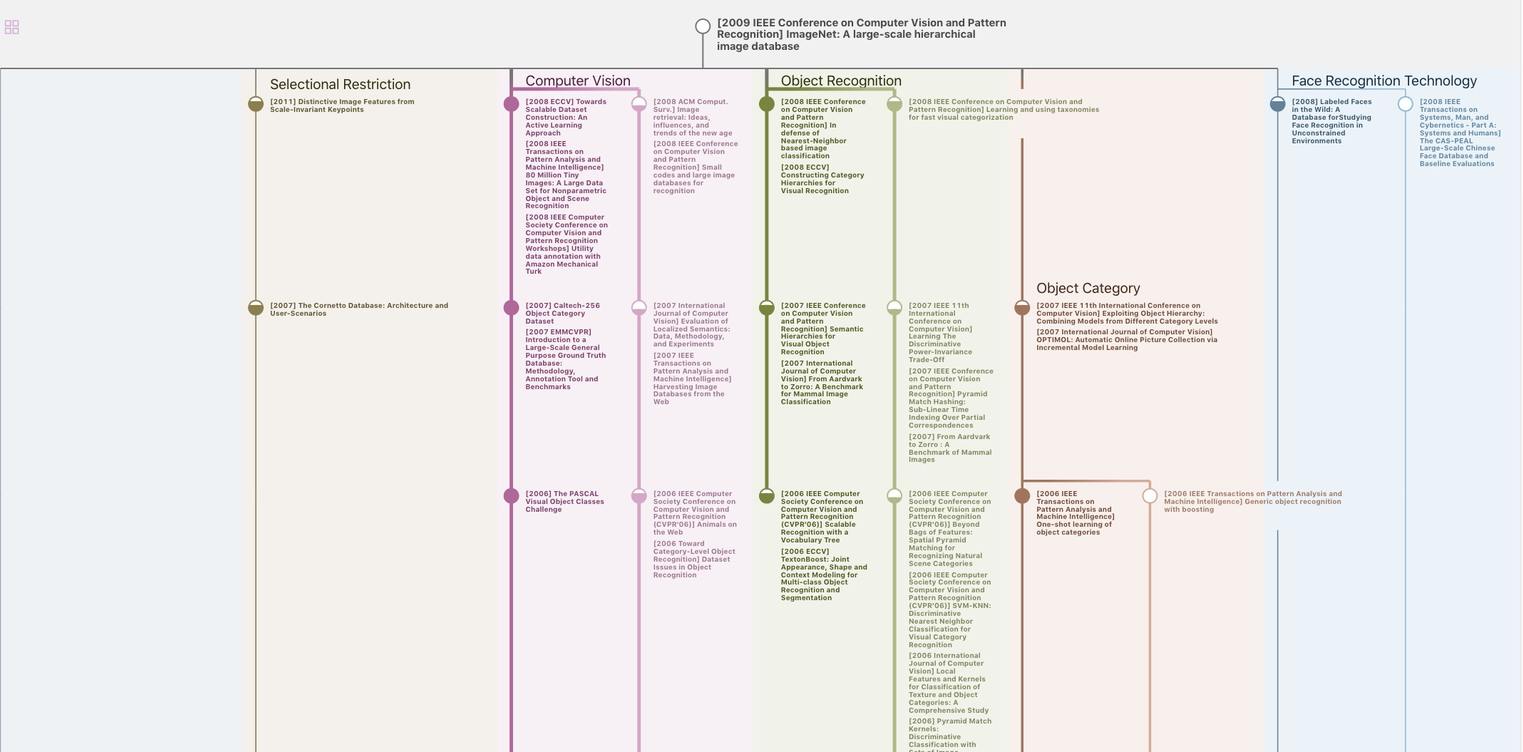
生成溯源树,研究论文发展脉络
Chat Paper
正在生成论文摘要