Speaker Overlap-aware Neural Diarization for Multi-party Meeting Analysis
emnlp 2022(2022)
摘要
Recently, hybrid systems of clustering and neural diarization models have been successfully applied in multi-party meeting analysis. However, current models always treat overlapped speaker diarization as a multi-label classification problem, where speaker dependency and overlaps are not well considered. To overcome the disadvantages, we reformulate overlapped speaker diarization task as a single-label prediction problem via the proposed power set encoding (PSE). Through this formulation, speaker dependency and overlaps can be explicitly modeled. To fully leverage this formulation, we further propose the speaker overlap-aware neural diarization (SOND) model, which consists of a context-independent (CI) scorer to model global speaker discriminability, a context-dependent scorer (CD) to model local discriminability, and a speaker combining network (SCN) to combine and reassign speaker activities. Experimental results show that using the proposed formulation can outperform the state-of-the-art methods based on target speaker voice activity detection, and the performance can be further improved with SOND, resulting in a 6.30% relative diarization error reduction.
更多查看译文
关键词
meeting
AI 理解论文
溯源树
样例
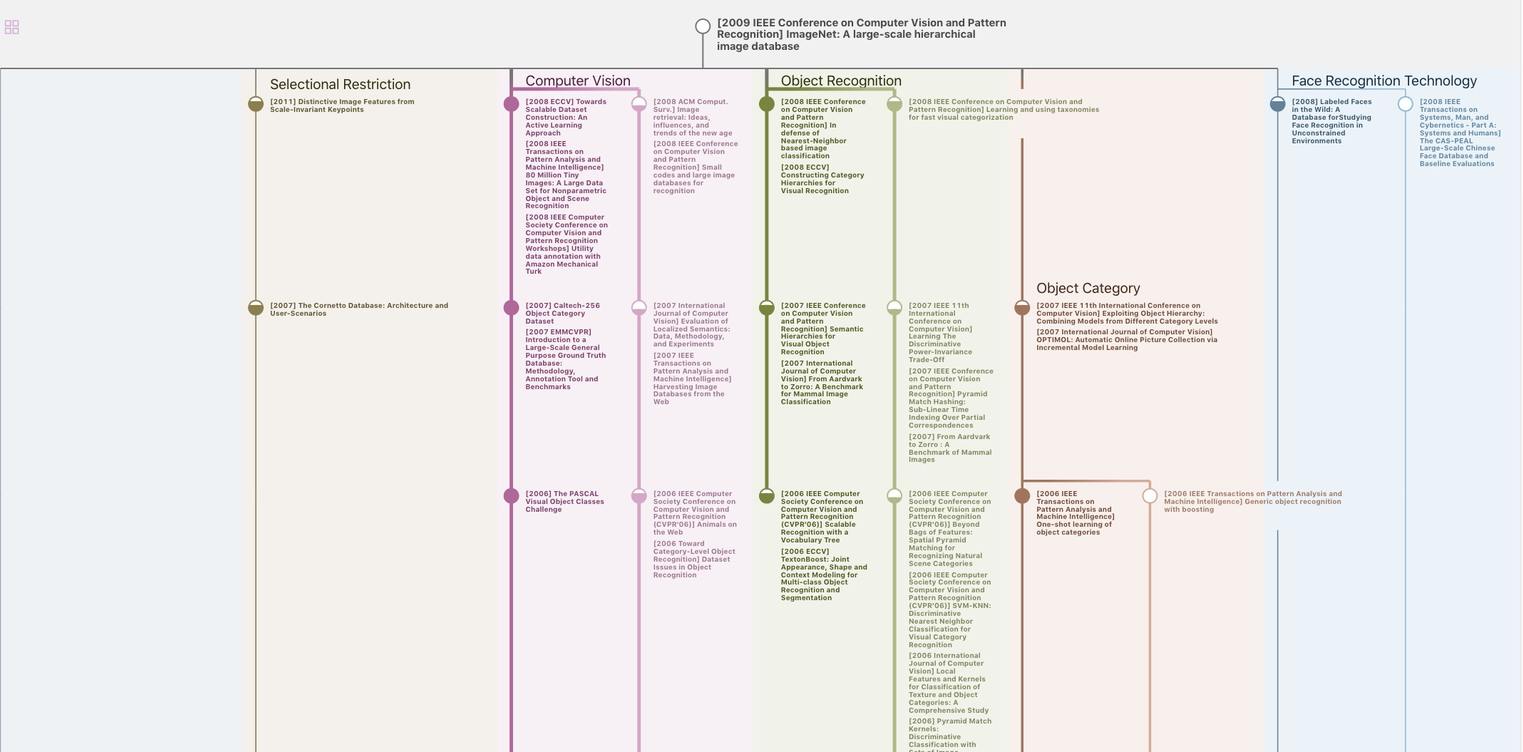
生成溯源树,研究论文发展脉络
Chat Paper
正在生成论文摘要