pmwd: A Differentiable Cosmological Particle-Mesh $N$-body Library
arxiv(2022)
摘要
The formation of the large-scale structure, the evolution and distribution of galaxies, quasars, and dark matter on cosmological scales, requires numerical simulations. Differentiable simulations provide gradients of the cosmological parameters, that can accelerate the extraction of physical information from statistical analyses of observational data. The deep learning revolution has brought not only myriad powerful neural networks, but also breakthroughs including automatic differentiation (AD) tools and computational accelerators like GPUs, facilitating forward modeling of the Universe with differentiable simulations. Because AD needs to save the whole forward evolution history to backpropagate gradients, current differentiable cosmological simulations are limited by memory. Using the adjoint method, with reverse time integration to reconstruct the evolution history, we develop a differentiable cosmological particle-mesh (PM) simulation library pmwd (particle-mesh with derivatives) with a low memory cost. Based on the powerful AD library JAX, pmwd is fully differentiable, and is highly performant on GPUs.
更多查看译文
关键词
particle-mesh
AI 理解论文
溯源树
样例
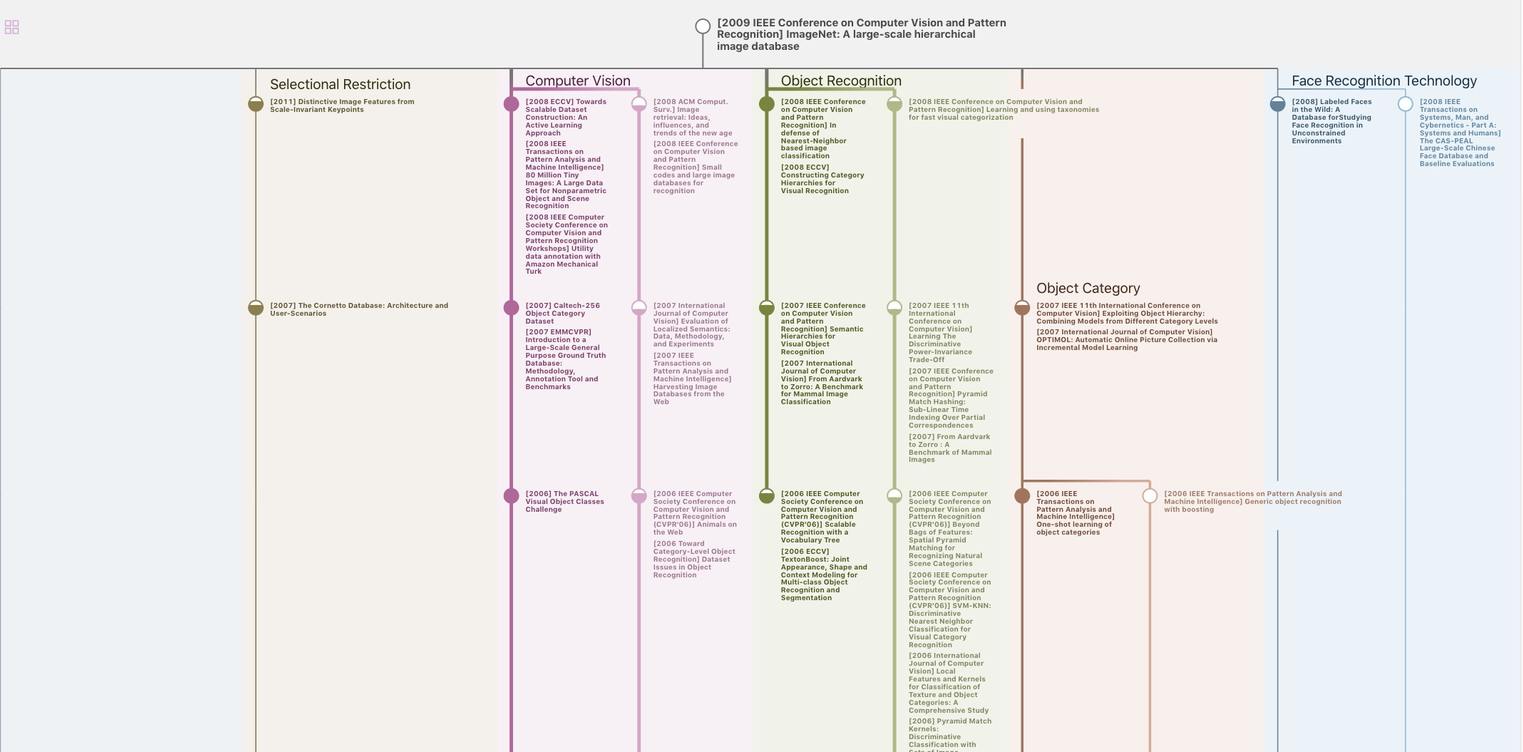
生成溯源树,研究论文发展脉络
Chat Paper
正在生成论文摘要