Improving Subseasonal-to-Seasonal forecasts in predicting the occurrence of extreme precipitation events over the contiguous US using machine learning models
Atmospheric Research(2023)
摘要
The precipitation forecasts at the Subseasonal-to-Seasonal (S2S) scale are valuable information to assist water resources planning and decision-making at an extended range. But the raw S2S precipitation forecasts are rather limited regarding their predictive skills, which hinders further hydrological applications. Many previous studies were carried out to validate the S2S precipitation forecasts from the perspective of the ensemble "mean", while existing study, which evaluates and improves the ability of S2S forecasts in predicting extreme precipitation events with their ensemble spreads, is rarely reported. This study aims to improve the S2S forecasts in predicting the occurrence of weekly extreme precipitation events above 99% over the contiguous United States (CONUS). The Random Forest Classifiers (RF) were employed and additional forecasts variables (i.e., surface air temper-ature, geopotential heights at 500 hPa and 850 hPa) were included to post-process the raw S2S forecasts from the NASA's Goddard Earth Observation System model version five (GEOS5). Different RF training inputs and RF hyperparameter sensitivity analysis are examined. We found that (1) using S2S precipitation forecast as the only inputs to RF, the forecast quality is improved significantly only at week 1; (2) When additional forecasts variables are included in RF training, the forecast skill tend to improve slightly at longer lead times after weeks 2; (3) The tunning of the maximum tree depth of RFs combine with the inclusion of additional forecasts variables as inputs to RF can improve the forecasts skill at all lead times over CONUS. In short, this study demonstrated the effectiveness of the application of RF as well as the effectiveness of additional forecast variables in improving the S2S extreme precipitation forecasts, which could be potentially useful for flood and river ensemble forecasting. Multiple statistical metrics, including the ensemble probability of detections (EPOD), ensemble false alarm ratios (EFAR), ensemble critical success index (ECSI), Brier Sill Score (BSS), and Area Under the Receiver Operating Characteristics Curves (AUROC) are employed for a comprehensive evaluation of the predictive performances of S2S extreme precipitation forecasts under different experiment scenarios.
更多查看译文
关键词
S2S,Extreme,Precipitation,Ensemble forecast,Machine learning,CONUS
AI 理解论文
溯源树
样例
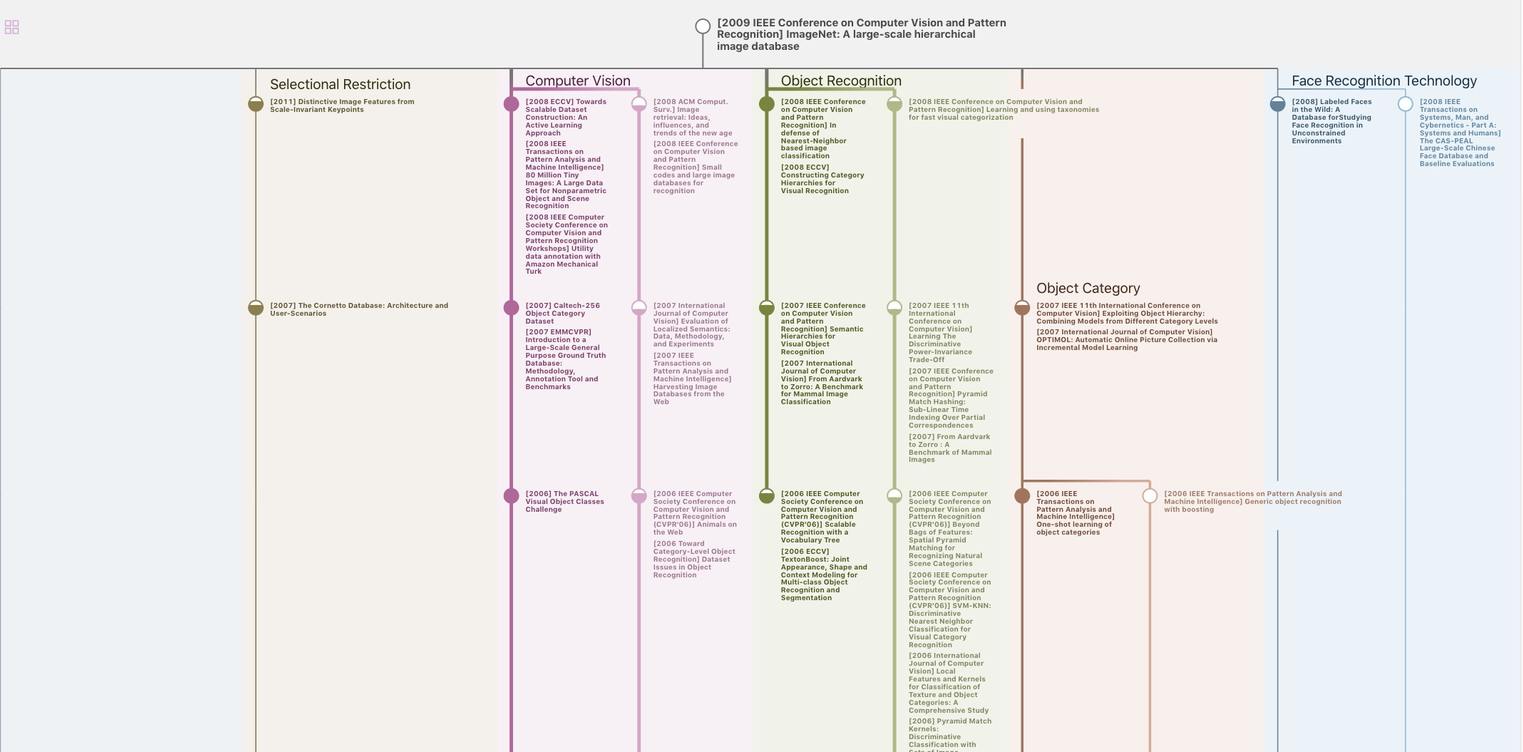
生成溯源树,研究论文发展脉络
Chat Paper
正在生成论文摘要