Enhancing Local Context of Histology Features in Vision Transformers.
AIIIMA/MIABID@MICCAI(2022)
摘要
Predicting complete response to radiotherapy in rectal cancer patients using deep learning approaches from morphological features extracted from histology biopsies provides a quick, low-cost and effective way to assist clinical decision making. We propose adjustments to the Vision Transformer (ViT) network to improve the utilisation of contextual information present in whole slide images (WSIs). Firstly, our position restoration embedding (PRE) preserves the spatial relationship between tissue patches, using their original positions on a WSI. Secondly, a clustering analysis of extracted tissue features explores morphological motifs which capture fundamental biological processes found in the tumour micro-environment. This is introduced into the ViT network in the form of a cluster label token, helping the model to differentiate between tissue types. The proposed methods are demonstrated on two large independent rectal cancer datasets of patients selectively treated with radiotherapy and capecitabine in two UK clinical trials. Experiments demonstrate that both models, PREViT and ClusterViT, show improvements in the prediction over baseline models.
更多查看译文
关键词
Vision Transformer,Histology,Whole slide image,Clustering
AI 理解论文
溯源树
样例
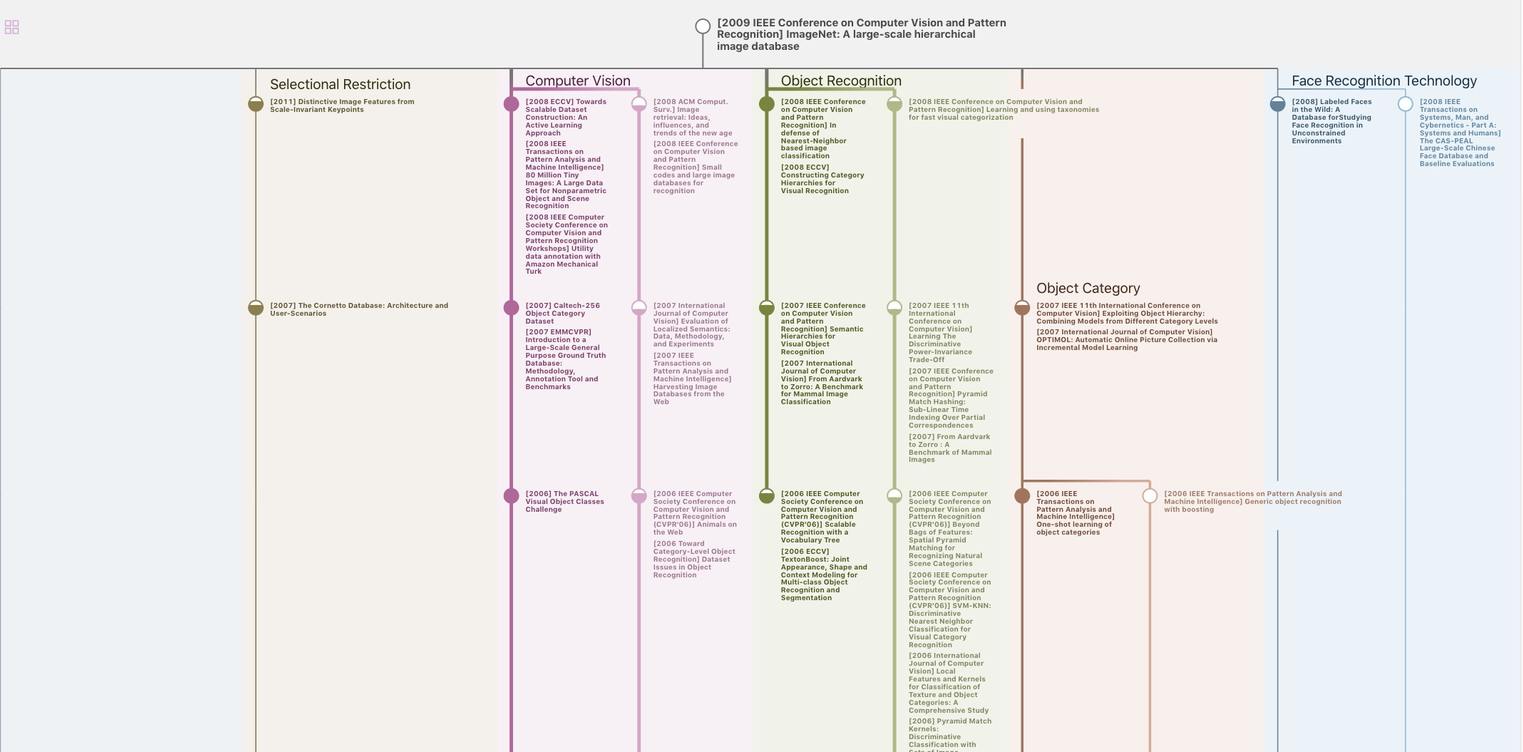
生成溯源树,研究论文发展脉络
Chat Paper
正在生成论文摘要