A Dynamic Heterogeneous Graph Convolution Network For Traffic Flow Prediction
COMPUTER JOURNAL(2024)
摘要
Traffic prediction has attracted a lot of attention in recent years. However, it is challenging due to the dynamic and heterogeneous spatial-temporal correlations. Most of the existing methods adopt the attention mechanism to capture the dynamic spatial features, but it is difficult for the attention mechanism to learn the real-time change of spatial dependencies, which restrict accurate spatial dependencies learning. Furthermore, most methods are out at elbows when solving heterogeneous spatial-temporal data. To overcome these problems, we propose a novel traffic prediction model called Dynamic spatial-temporal Heterogeneous Graph Convolution Network. Different from the existing methods, we have designed a dynamic localized graph and a corresponding adaptive localized graph convolution network, which are capable of simultaneously capturing dynamic and heterogeneous spatial correlations. We propose a gated adaptive temporal convolution network to capture the temporal heterogeneity of traffic data and enjoy global receptive fields. Finally, a global correlations fusion network is provided to incorporate the global spatial-temporal correlations. Compared with 11 baselines, our proposed model achieves state-of-the-art performance in the accuracy of prediction.
更多查看译文
关键词
spatial-temporal Graph,Traffic Prediction,Graph Neural Network,Self-Attention
AI 理解论文
溯源树
样例
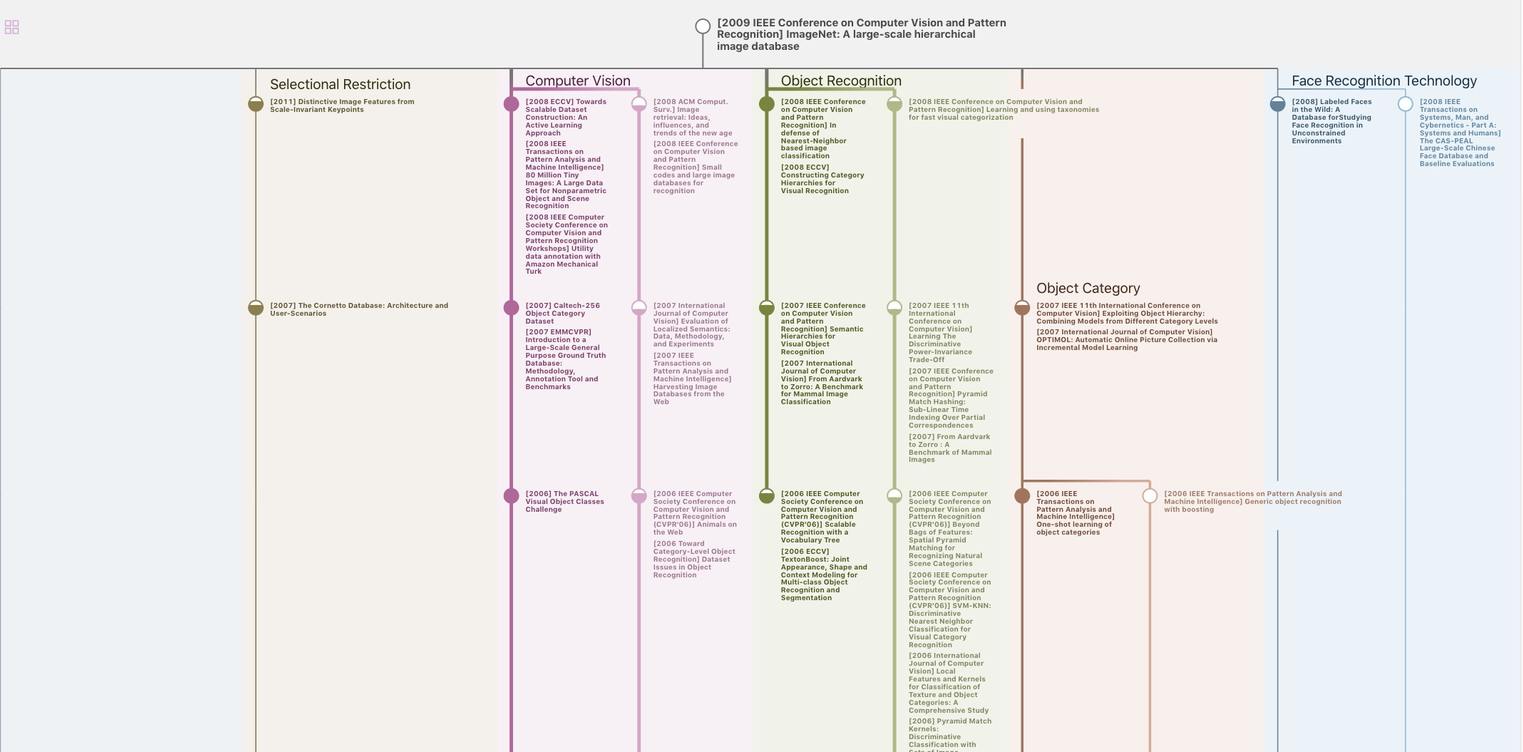
生成溯源树,研究论文发展脉络
Chat Paper
正在生成论文摘要