Accounting for Bias in the Estimation of r 2 between Two Sets of Noisy Neural Responses.
The Journal of neuroscience : the official journal of the Society for Neuroscience(2022)
摘要
The Pearson correlation coefficient squared, , is an important tool used in the analysis of neural data to quantify the similarity between neural tuning curves. Yet this metric is biased by trial-to-trial variability; as trial-to-trial variability increases, measured correlation decreases. Major lines of research are confounded by this bias, including those involving the study of invariance of neural tuning across conditions and the analysis of the similarity of tuning across neurons. To address this, we extend an estimator, [Formula: see text], that was recently developed for estimating model-to-neuron correlation, in which a noisy signal is compared with a noise-free prediction, to the case of neuron-to-neuron correlation, in which two noisy signals are compared with each other. We compare the performance of our novel estimator to a prior method developed by Spearman, commonly used in other fields but widely overlooked in neuroscience, and find that our method has less bias. We then apply our estimator to demonstrate how it avoids drastic confounds introduced by trial-to-trial variability using data collected in two prior studies (macaque, both sexes) that examined two different forms of invariance in the neural encoding of visual inputs-translation invariance and fill-outline invariance. Our results quantify for the first time the gradual falloff with spatial offset of translation-invariant shape selectivity within visual cortical neuronal receptive fields and offer a principled method to compare invariance in noisy biological systems to that in noise-free models. Quantifying the similarity between two sets of averaged neural responses is fundamental to the analysis of neural data. A ubiquitous metric of similarity, the correlation coefficient, is attenuated by trial-to-trial variability that arises from many irrelevant factors. Spearman recognized this problem and proposed corrected methods that have been extended over a century. We show this method has large asymptotic biases that can be overcome using a novel estimator. Despite the frequent use of the correlation coefficient in neuroscience, consensus on how to address this fundamental statistical issue has not been reached. We provide an accurate estimator of the correlation coefficient and apply it to gain insight into visual invariance.
更多查看译文
关键词
V4,confound,correlation,invariance,sensory,statistics
AI 理解论文
溯源树
样例
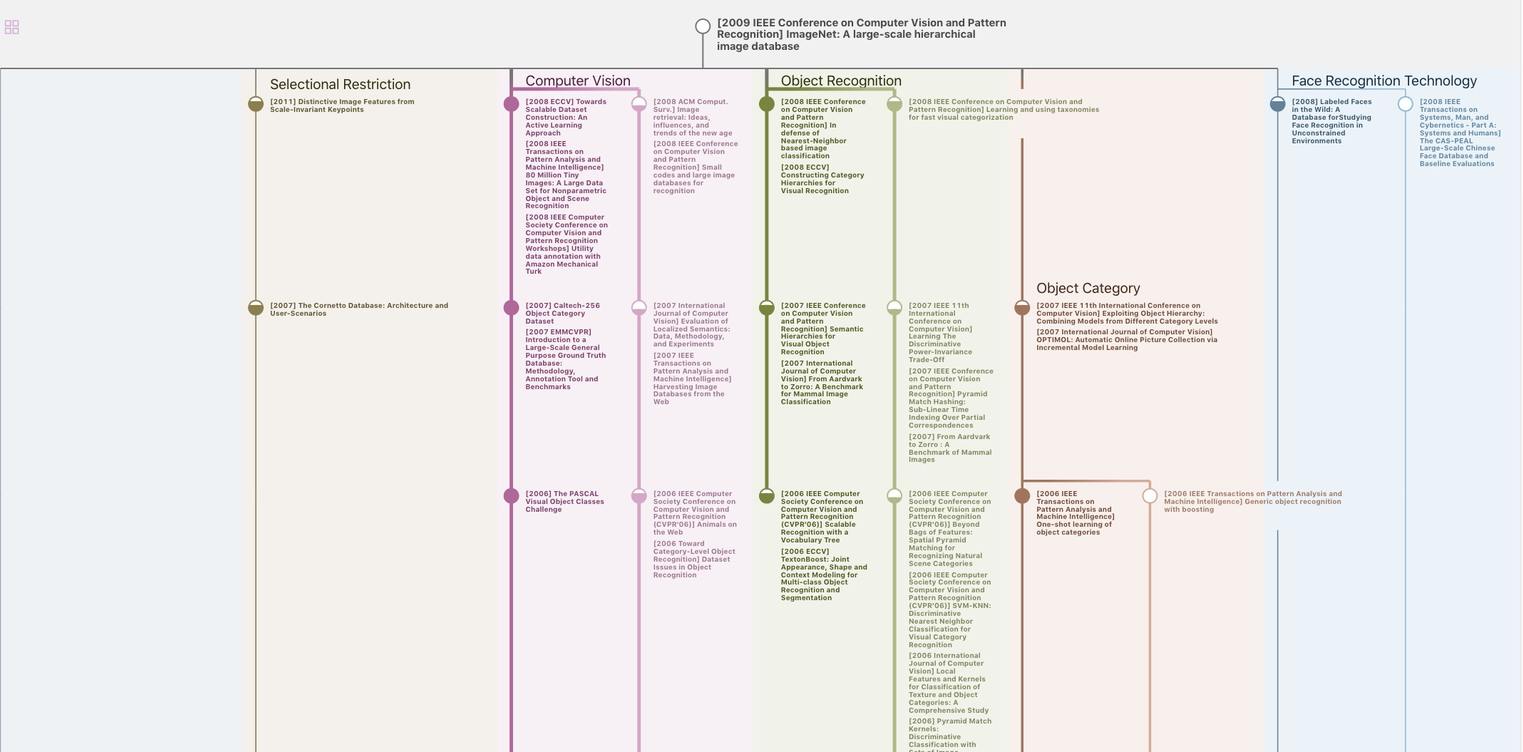
生成溯源树,研究论文发展脉络
Chat Paper
正在生成论文摘要