A Novel Automated Approach to Mutation-Cancer Relation Extraction by Incorporating Heterogeneous Knowledge
IEEE Journal of Biomedical and Health Informatics(2023)
摘要
Automatic extraction of relations between gene mutations and cancer entities occurring in the cancer literature using text mining can rapidly provide vital information to support precision cancer medicine. However, mutation-cancer relation extraction is more challenging than general relation extraction from free text, since it is often not possible without cancer-specific background knowledge and thus the model replies on a deeper understanding of complex surrounding tokens. We propose a deep learning model that jointly extracts mutations and their associated cancers. Background knowledge comes from two different knowledge bases which store different types of information about mutations. Given the different ways in which knowledge is stored in these two resources, we propose two separate methods for embedding knowledge, namely sentence-based knowledge integration and attribute-aware knowledge integration. The evaluation demonstrated that our model outperforms a number of baseline models and gains 96.00%, 92.57% and 94.57% F1 scores on three public datasets, EMU BCa, EMU PCa, and BRONCO, thus illustrating the effectiveness of our knowledge integration approach. The auxiliary experiments show that our models can utilize more informative text from the KBs and link the mutations to their corresponding cancer disease although the input text provides insufficient context.
更多查看译文
关键词
Information extraction,relation extraction,biomedical text mining,deep learning
AI 理解论文
溯源树
样例
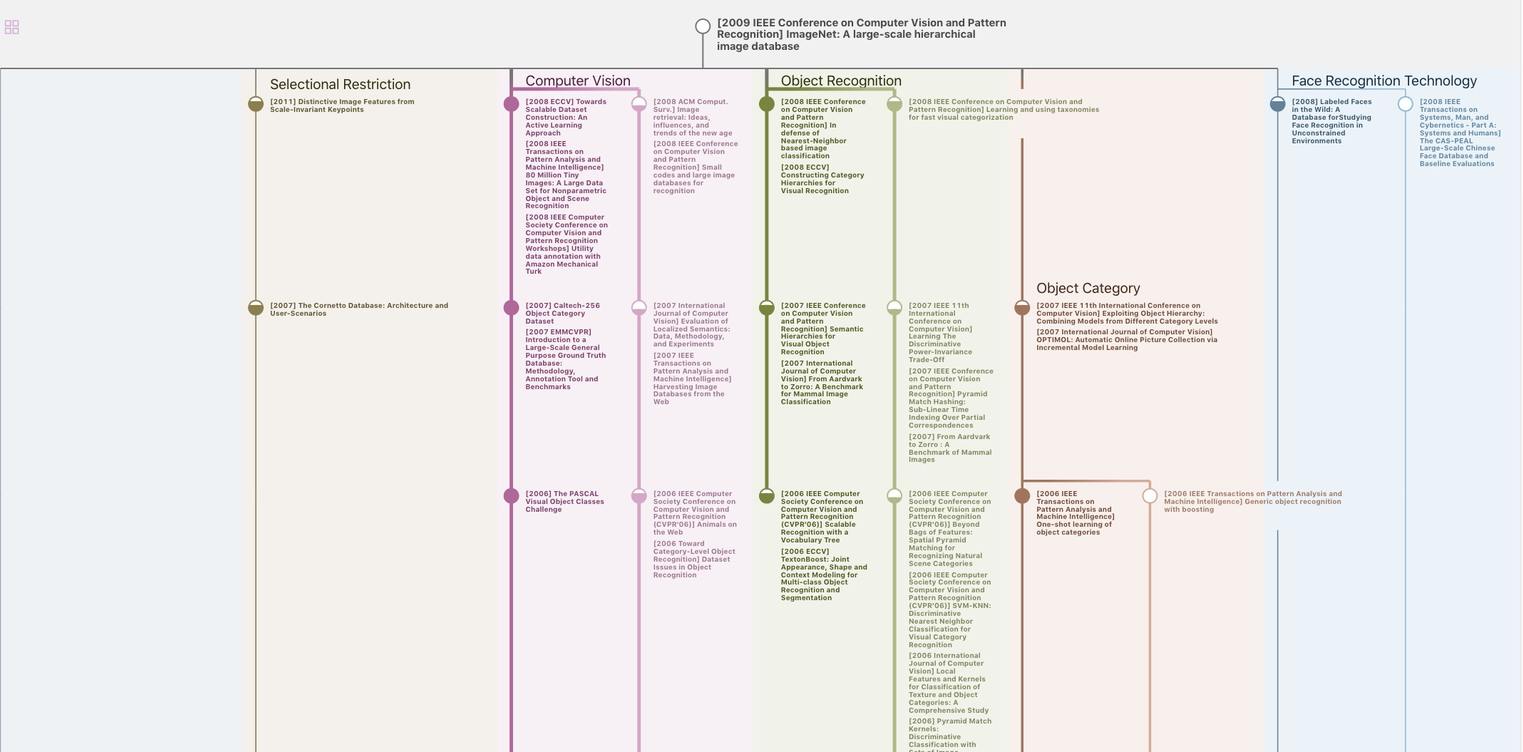
生成溯源树,研究论文发展脉络
Chat Paper
正在生成论文摘要