Adaptive Context Modeling for Arithmetic Coding Using Perceptrons.
IEEE Signal Process. Lett.(2022)
摘要
Arithmetic coding is used in most media compression methods. Context modeling is usually done through frequency counting and look-up tables (LUTs). For long-memory signals, probability modeling with large context sizes is often infeasible. Recently, neural networks have been used to model probabilities of large contexts in order to drive arithmetic coders. These neural networks have been trained offline. We introduce an online method for training a perceptron-based context-adaptive arithmetic coder on-the-fly, called adaptive perceptron coding, which continuously learns the context probabilities and quickly converges to the signal statistics. We test adaptive perceptron coding over a binary image database, with results always exceeding the performance of LUT-based methods for large context sizes and of recurrent neural networks. We also compare the method to a version requiring offline training, which leads to equally satisfactory results.
更多查看译文
关键词
arithmetic coding,adaptive context modeling,perceptrons
AI 理解论文
溯源树
样例
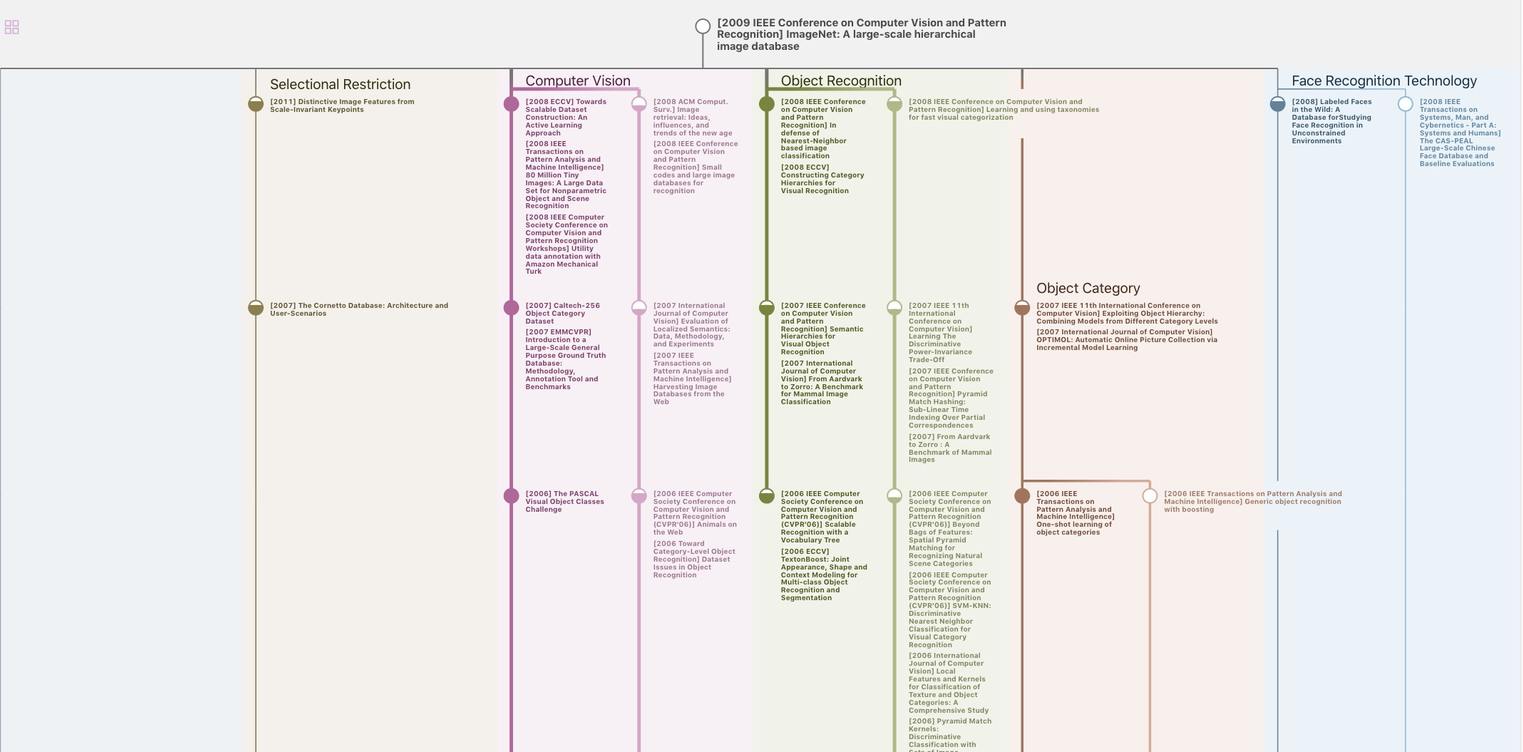
生成溯源树,研究论文发展脉络
Chat Paper
正在生成论文摘要