Path reliability-based graph attention networks
NEURAL NETWORKS(2023)
摘要
Self-attention mechanism has been successfully introduced in Graph Neural Networks (GNNs) for graph representation learning and achieved state-of-the-art performances in tasks such as node classification and node attacks. In most existing attention-based GNNs, attention score is only computed between two directly connected nodes with their representation at a single layer. However, this attention score computation method cannot account for its multi-hop neighbors, which supply graph structure information and have influence on many tasks such as link prediction, knowledge graph completion, and adversarial attack as well. In order to address this problem, in this paper, we propose Path Reliability-based Graph Attention Networks (PRGATs), a novel method to incorporate multi-hop neighboring context into attention score computation, enabling to capture longer-range dependencies and large-scale structural information within a single layer. Moreover, path reliability-based attention layer, a core layer of PRGATs, uses a resource-constrain allocation algorithm to compute the reliable path and its attention scores from neighboring nodes to non-neighboring nodes, increasing the receptive field for every message-passing layer. Experimental results on real-world datasets show that, as compared with baselines, our model outperforms existing methods up to 3% on standard node classification and 12% on graph universal adversarial attack.(c) 2022 Elsevier Ltd. All rights reserved.
更多查看译文
关键词
graph attention networks,reliability-based
AI 理解论文
溯源树
样例
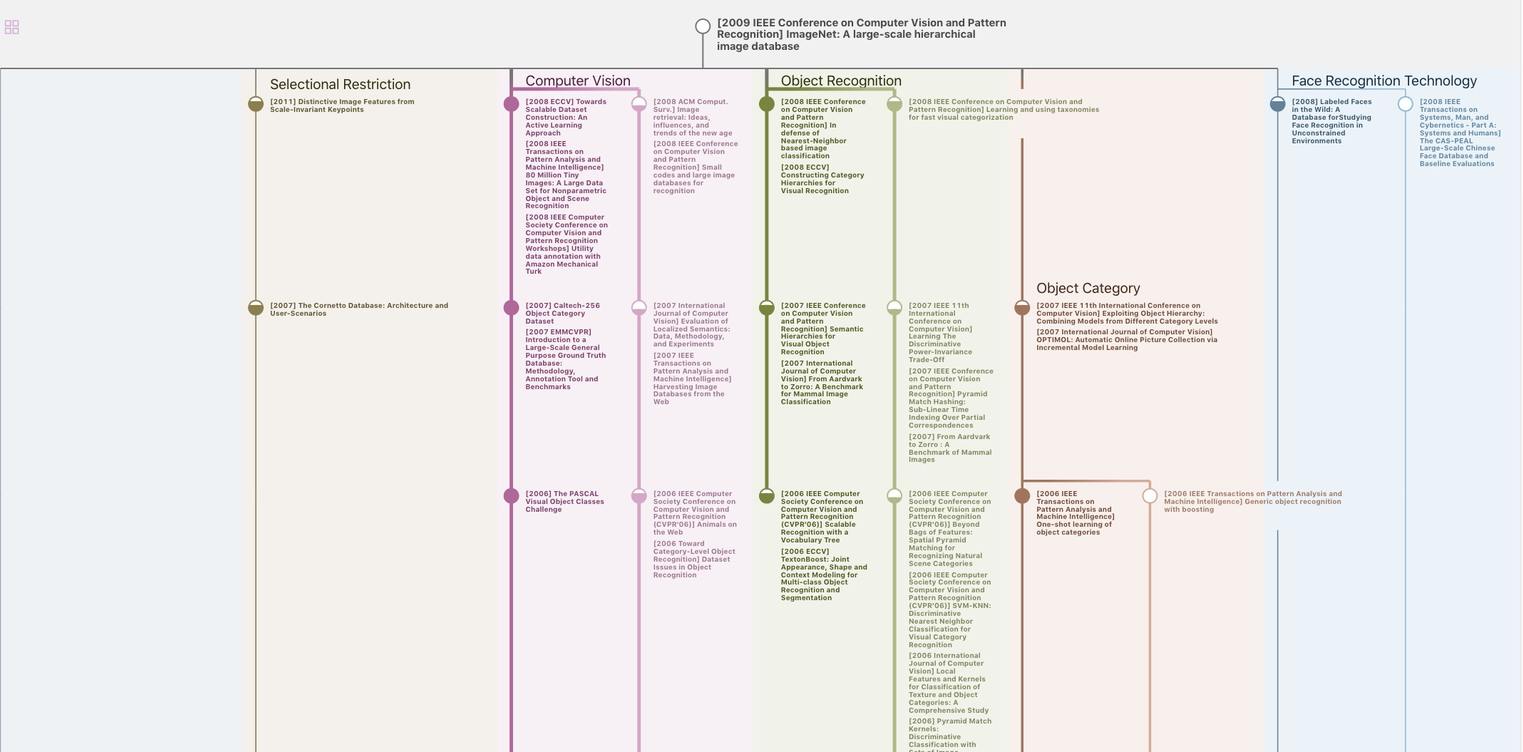
生成溯源树,研究论文发展脉络
Chat Paper
正在生成论文摘要