Improved One-Stage Detectors with Neck Attention Block for Object Detection in Remote Sensing
REMOTE SENSING(2022)
摘要
Object detection in remote sensing is becoming a conspicuous challenge with the rapidly increasing quantity and quality of remote sensing images. Although the application of Deep Learning has obtained remarkable performance in Computer Vision, detecting multi-scale targets in remote sensing images is still an unsolved problem, especially for small instances which possess limited features and intricate backgrounds. In this work, we managed to cope with this problem by designing a neck attention block (NAB), a simple and flexible module which combines the convolutional bottleneck structure and the attention mechanism, different from traditional attention mechanisms that focus on designing complicated attention branches. In addition, Vehicle in High-Resolution Aerial Imagery (VHRAI), a diverse, dense, and challenging dataset, was proposed for studying small object detection. To validate the effectiveness and generalization of NAB, we conducted experiments on a variety of datasets with the improved YOLOv3, YOLOv4-Tiny, and SSD. On VHRAI, the improved YOLOv3 and YOLOv4-Tiny surpassed the original models by 1.98% and 1.89% mAP, respectively. Similarly, they exceeded the original models by 1.12% and 3.72% mAP on TGRS-HRRSD, a large multi-scale dataset. Including SSD, these three models also showed excellent generalizability on PASCAL VOC.
更多查看译文
关键词
remote sensing,multi-scale object detection,small object detection,attention mechanism,YOLOv3,YOLOv4-Tiny,SSD
AI 理解论文
溯源树
样例
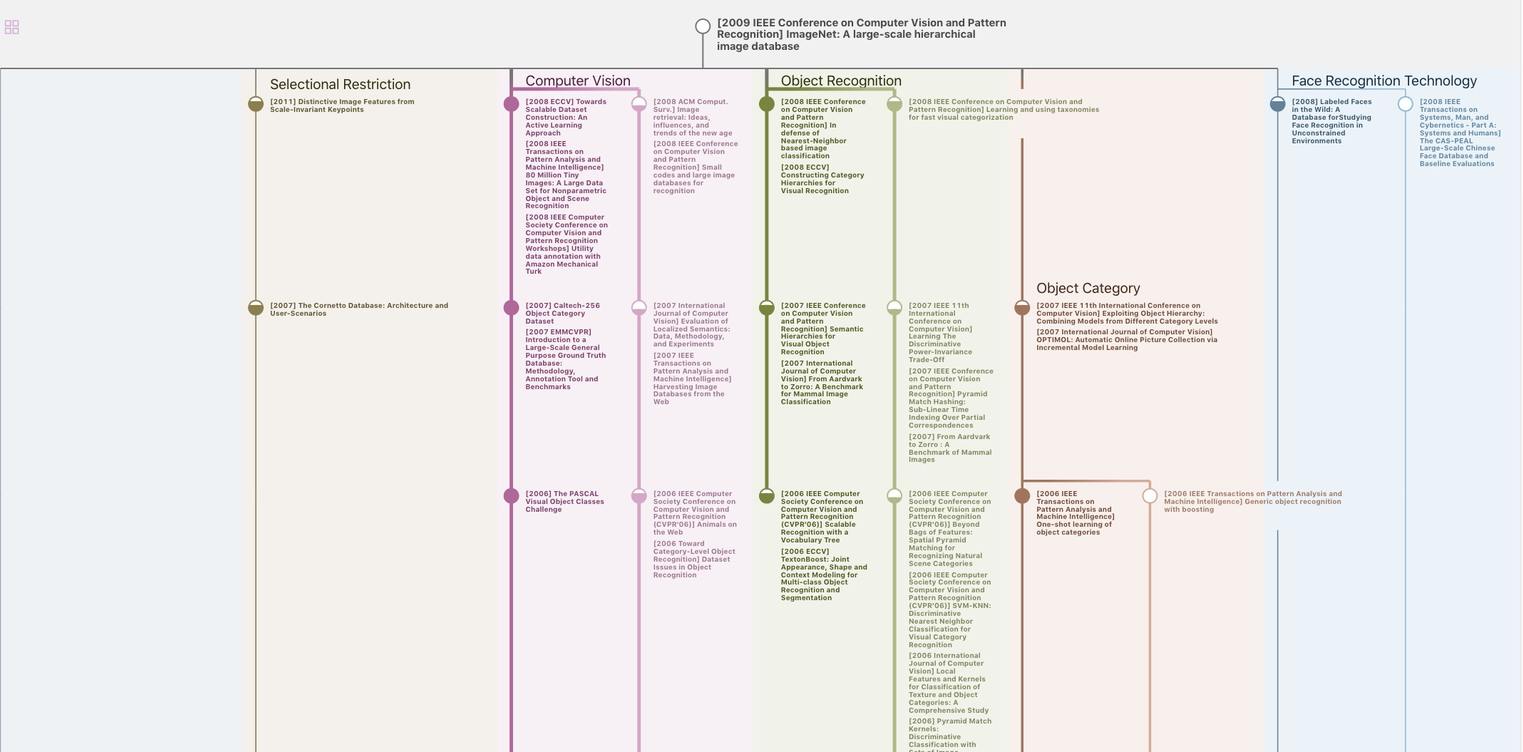
生成溯源树,研究论文发展脉络
Chat Paper
正在生成论文摘要