RGN: Residue-Based Graph Attention and Convolutional Network for Protein-Protein Interaction Site Prediction
JOURNAL OF CHEMICAL INFORMATION AND MODELING(2022)
摘要
The prediction of a protein-protein interaction site (PPI site) plays a very important role in the biochemical process, and lots of computational methods have been proposed in the past. However, the majority of the past methods are time consuming and lack accuracy. Hence, coming up with an effective computational method is necessary. In this article, we present a novel computational model called RGN (residue-based graph attention and convolutional network) to predict PPI sites. In our paper, the protein is treated as a graph. The amino acid can be seen as the node in the graph structure. The position-specific scoring matrix, hidden Markov model, hydrogen bond estimation algorithm, and ProtBert are applied as node features. The edges are decided by the spatial distance between the amino acids. Then, we utilize a residue-based graph convolutional network and graph attention network to further extract the deeper feature. Finally, the processed node feature is fed into the prediction layer. We show the superiority of our model by comparing it with the other four protein structure-based methods and five protein sequence-based methods. Our model obtains the best performance on all the evaluation metrics (accuracy, precision, recall, F1 score, Matthews correlation coefficient, area under the receiver operating characteristic curve, and area under the precision recall curve). We also conduct a case study to demonstrate that extracting the protein information from the protein structure perspective is effective and points out the difficult aspect of PPI site prediction.
更多查看译文
关键词
protein–protein interaction site prediction,graph attention,convolutional network,residue-based
AI 理解论文
溯源树
样例
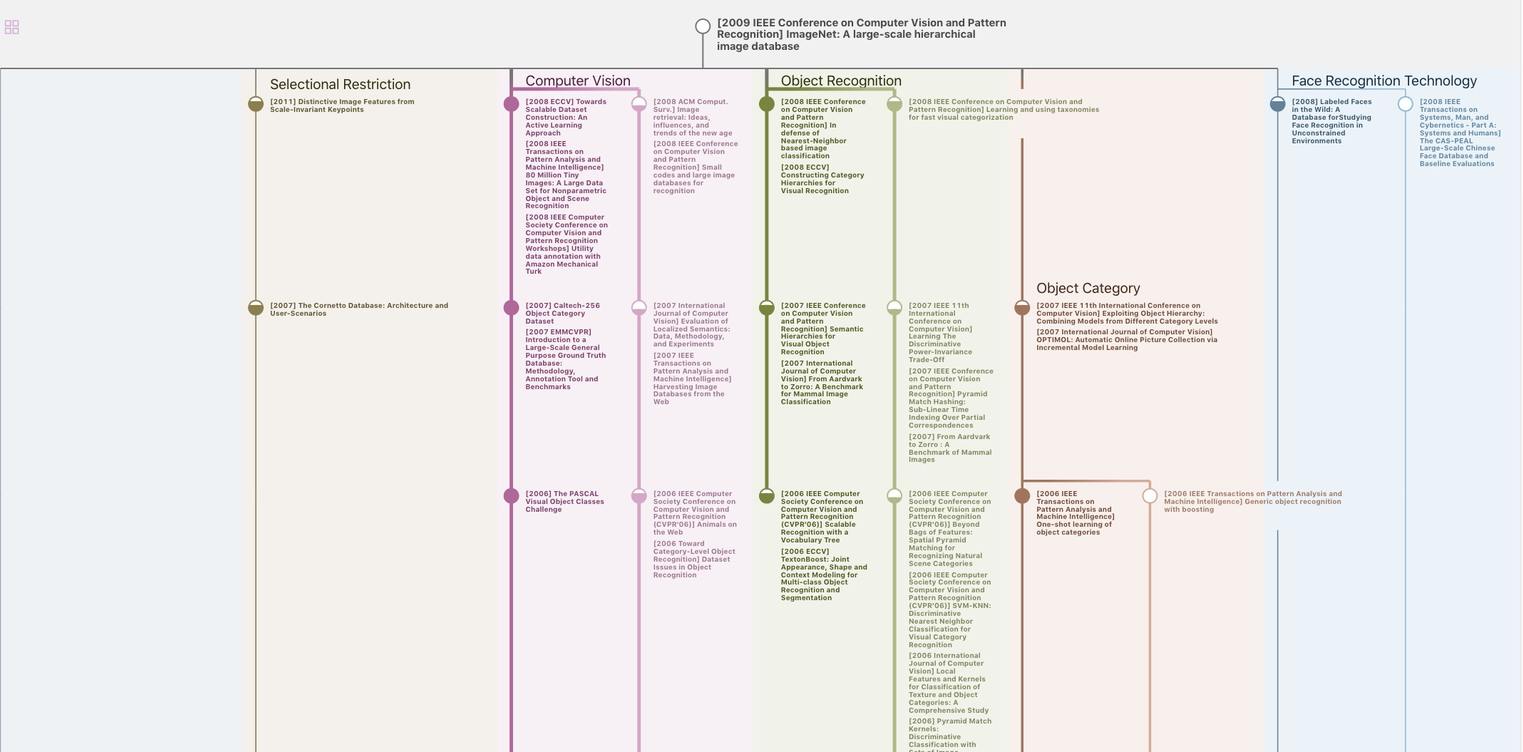
生成溯源树,研究论文发展脉络
Chat Paper
正在生成论文摘要