Multi-Granularity Anchor-Contrastive Representation Learning for Semi-Supervised Skeleton-Based Action Recognition.
IEEE transactions on pattern analysis and machine intelligence(2023)
摘要
In the semi-supervised skeleton-based action recognition task, obtaining more discriminative information from both labeled and unlabeled data is a challenging problem. As the current mainstream approach, contrastive learning can learn more representations of augmented data, which can be considered as the pretext task of action recognition. However, such a method still confronts three main limitations: 1) It usually learns global-granularity features that cannot well reflect the local motion information. 2) The positive/negative pairs are usually pre-defined, some of which are ambiguous. 3) It generally measures the distance between positive/negative pairs only within the same granularity, which neglects the contrasting between the cross-granularity positive and negative pairs. Toward these limitations, we propose a novel Multi-granularity Anchor-Contrastive representation Learning (dubbed as MAC-Learning) to learn multi-granularity representations by conducting inter- and intra-granularity contrastive pretext tasks on the learnable and structural-link skeletons among three types of granularities covering local, context, and global views. To avoid the disturbance of ambiguous pairs from noisy and outlier samples, we design a more reliable Multi-granularity Anchor-Contrastive Loss (dubbed as MAC-Loss) that measures the agreement/disagreement between high-confidence soft-positive/negative pairs based on the anchor graph instead of the hard-positive/negative pairs in the conventional contrastive loss. Extensive experiments on both NTU RGB+D and Northwestern-UCLA datasets show that the proposed MAC-Learning outperforms existing competitive methods in semi-supervised skeleton-based action recognition tasks.
更多查看译文
关键词
Skeleton, Task analysis, Loss measurement, Joints, Semantics, Data models, Pattern recognition, Action recognition, skeleton, semi-supervised, contrastive learning, anchor graph
AI 理解论文
溯源树
样例
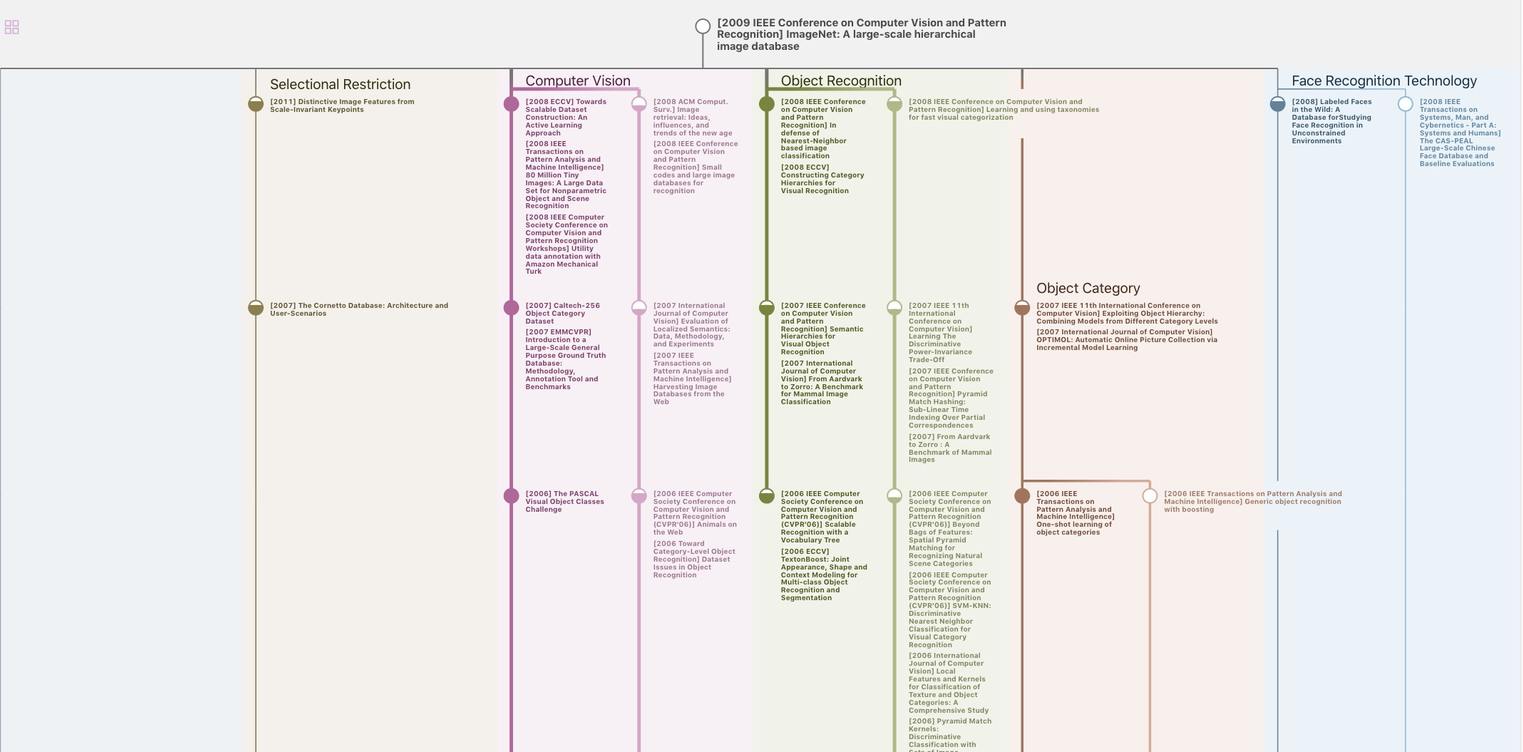
生成溯源树,研究论文发展脉络
Chat Paper
正在生成论文摘要