AligNeRF: High-Fidelity Neural Radiance Fields via Alignment-Aware Training
CVPR 2023(2023)
摘要
Neural Radiance Fields (NeRFs) are a powerful representation for modeling a 3D scene as a continuous function. Though NeRF is able to render complex 3D scenes with view-dependent effects, few efforts have been devoted to exploring its limits in a high-resolution setting. Specifically, existing NeRF-based methods face several limitations when reconstructing high-resolution real scenes, including a very large number of parameters, misaligned input data, and overly smooth details. In this work, we conduct the first pilot study on training NeRF with high-resolution data and propose the corresponding solutions: 1) marrying the multilayer perceptron (MLP) with convolutional layers which can encode more neighborhood information while reducing the total number of parameters; 2) a novel training strategy to address misalignment caused by moving objects or small camera calibration errors; and 3) a high-frequency aware loss. Our approach is nearly free without introducing obvious training/testing costs, while experiments on different datasets demonstrate that it can recover more high-frequency details compared with the current state-of-the-art NeRF models. Project page: https://yifanjiang19.github.io/alignerf.
更多查看译文
关键词
high-fidelity,alignment-aware
AI 理解论文
溯源树
样例
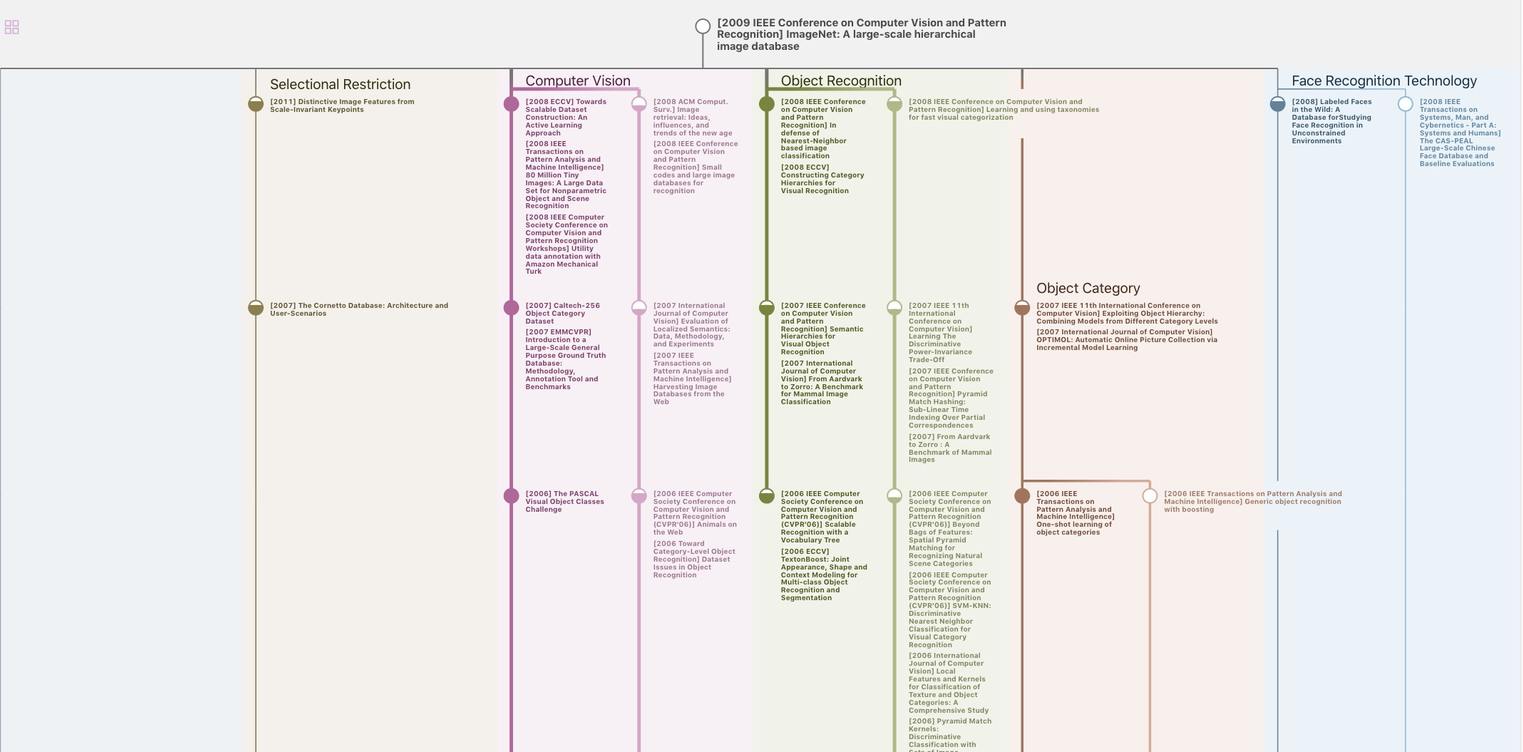
生成溯源树,研究论文发展脉络
Chat Paper
正在生成论文摘要