Predicting Human Mobility via Self-Supervised Disentanglement Learning
IEEE TRANSACTIONS ON KNOWLEDGE AND DATA ENGINEERING(2024)
摘要
Deep neural networks have recently achieved considerable improvements in learning human behavioral patterns and individual preferences from massive spatial-temporal trajectory data. However, most of the existing research concentrates on fusing different semantics underlying sequential trajectories for mobility pattern learning which, in turn, yields a narrow perspective on comprehending human intrinsic motions. In addition, the inherent sparsity and under-explored heterogeneous collaborative items pertaining to human check-ins hinder the potential exploitation of human diverse periodic regularities as well as common interests. Motivated by recent advances in disentanglement learning, we propose a novel disentangled solution called SSDL for tackling the next POI prediction problem. SSDL primarily seeks to disentangle the potential time-invariant and time-varying factors into different latent spaces from massive trajectories, providing an interpretable view to understand the intricate semantics underlying human diverse mobility representations. To address the data sparsity issue, we present two realistic trajectory augmentation approaches to enhance the understanding of both the human intrinsic periodicity/habits and constantly-changing intents. In addition, we devise a POI-centric graph structure to explore heterogeneous collaborative signals underlying historical check-ins. Extensive experiments conducted on four real-world datasets demonstrate that SSDL significantly outperforms the state-of-the-art approaches-for example, it yields up to 8.57% averaged improvement on ACC@1.
更多查看译文
关键词
Trajectory,Semantics,Behavioral sciences,Task analysis,Neural networks,Collaboration,Representation learning,Location-based services,human mobility,graph neural network,disentanglement learning,variational Bayes
AI 理解论文
溯源树
样例
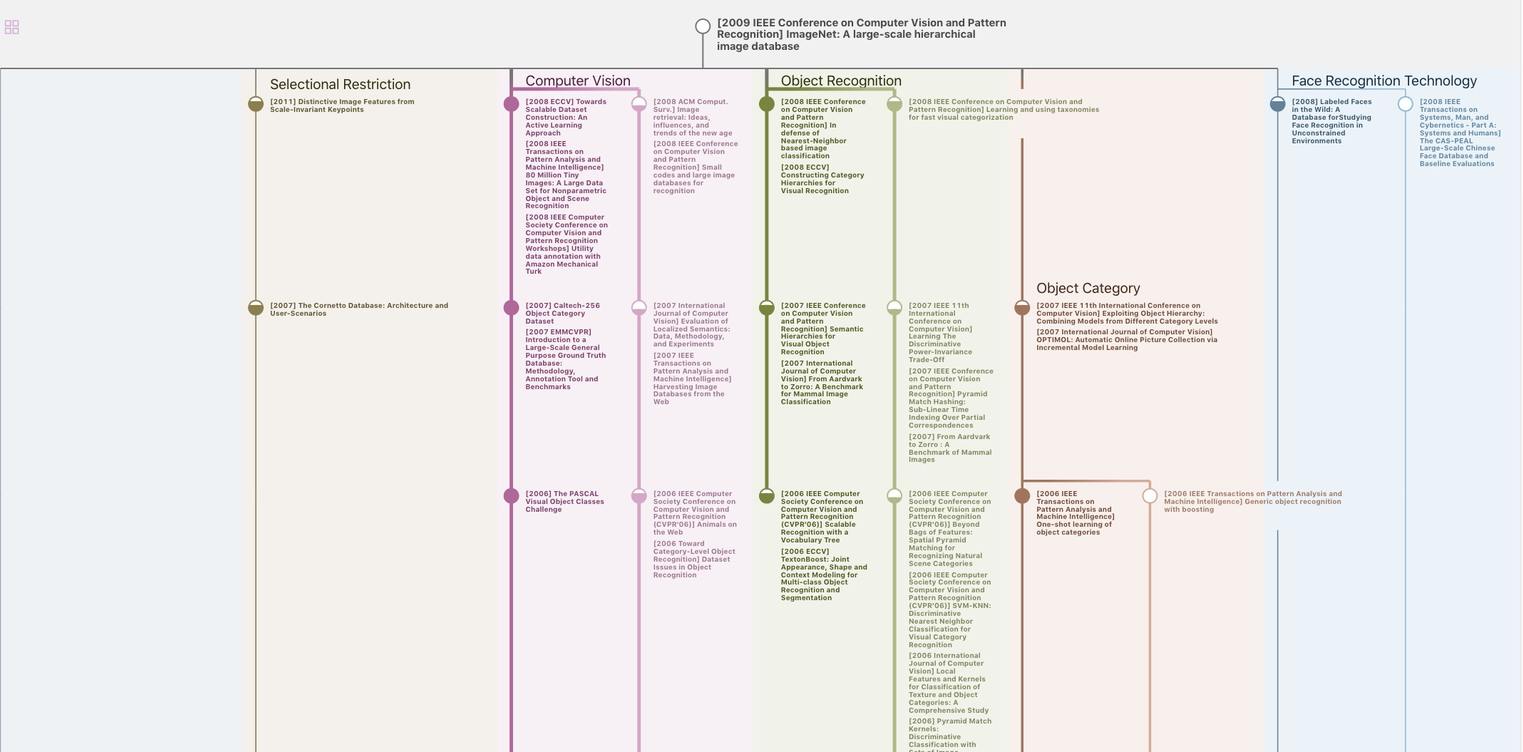
生成溯源树,研究论文发展脉络
Chat Paper
正在生成论文摘要