Event-Triggered Quantized Kernel Least Mean Square Algorithm
2022 IEEE 32nd International Workshop on Machine Learning for Signal Processing (MLSP)(2022)
摘要
The classical quantized kernel least mean square (QKLMS) algorithm curbs the linear growth of network structure effectively. However, the filtering accuracy of QKLMS highly depends on the predefined quantization threshold. To improve the filtering accuracy dynamically, an event-triggered mechanism is therefore designed to adjust the quantization threshold adaptively. The designed trigger updates the quantization threshold by monitoring the convergence rate of the learning curve. Applying the event-triggered mechanism into QKLM-S generates a novel event-triggered QKLMS (ETQKLMS) algorithm. In addition, the convergence analysis of quantization threshold is carried out. Examples of Chua's chaotic time series prediction is presented to verify the effectiveness of ETQKLMS.
更多查看译文
关键词
Kernel least mean square,event-triggered mechanism,adaptive quantization threshold,convergence rate
AI 理解论文
溯源树
样例
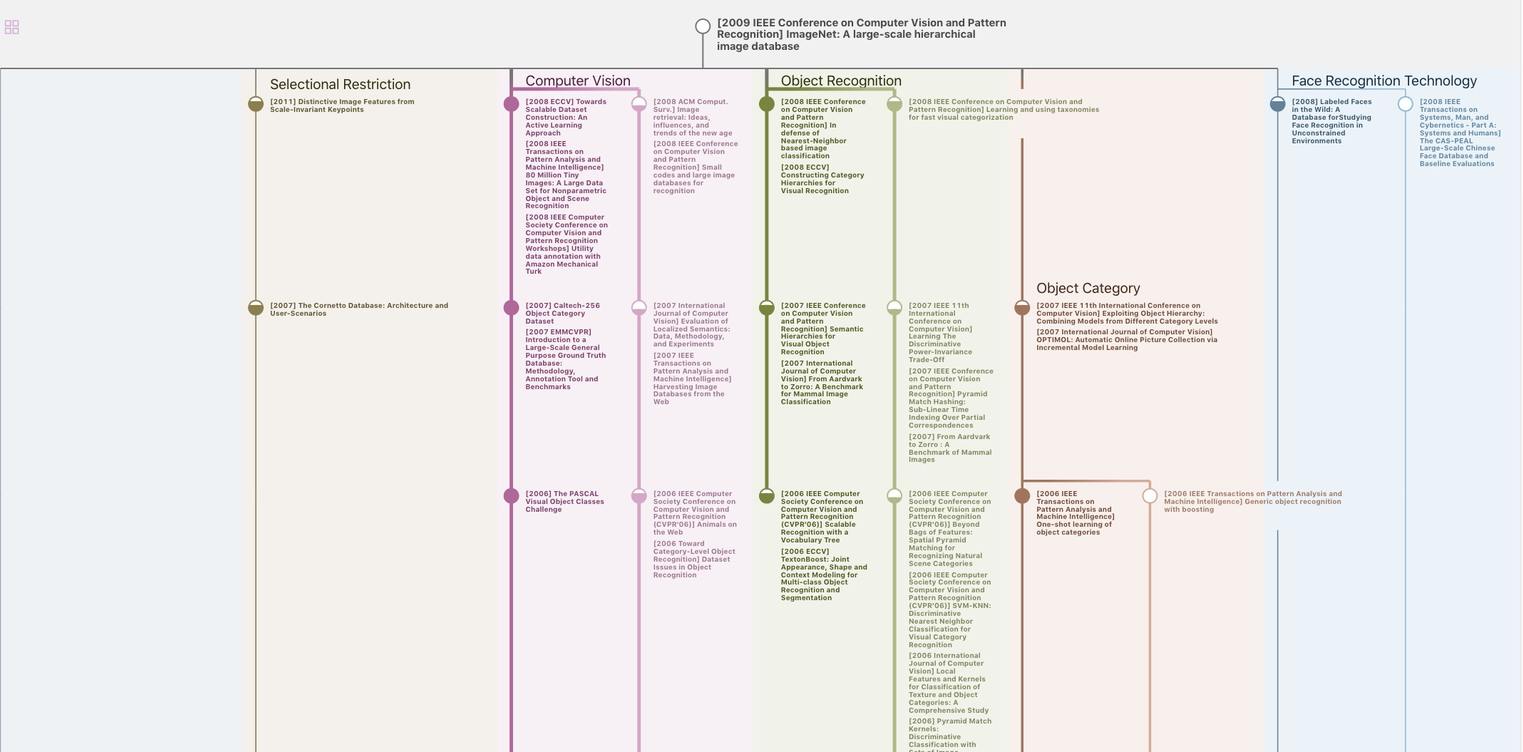
生成溯源树,研究论文发展脉络
Chat Paper
正在生成论文摘要