SHNN: A single-channel EEG sleep staging model based on semi-supervised learning
Expert Systems with Applications(2023)
摘要
Sleep staging is an essential step in the diagnosis and treatment of sleep-related diseases. Currently, most supervised learning models face the problem of insufficient labeled data. In addition, most sleep staging models are based on multi-channel EEG, and the models are too complex to be suitable for home sleep monitoring scenarios. To tackle these problems, this study proposes a sleep staging method based on pseudo-label optimization and a single-channel sleep hybrid neural network called SHNN. In the SHNN model, we design a multi-scale convolutional neural network (CNN) to extract the features from the single-channel EEG and use a Bi-directional recurrent gating unit (Bi-GRU) to obtain temporal context information of sleep data sequences. Extensive experiments based on the single-channel EEG (FPz-Cz, Pz-Oz, and Cz-A1) of the Sleep-EDFx and the DREAMS-SUB datasets validate the effectiveness of the SHNN model and the pseudo-label optimization algorithm therein outperforming current single-channel methods regarding the accuracy, kappa, and MF1 Score. Moreover, the pseudo-label optimization algorithm can achieve good results on other sleep staging methods. The SHNN code is available at https://github.com/Caowenpeng/SHNN.
更多查看译文
关键词
Automatic sleep staging,Single-channel,Pseudo-label optimization algorithm,Semi-supervised model
AI 理解论文
溯源树
样例
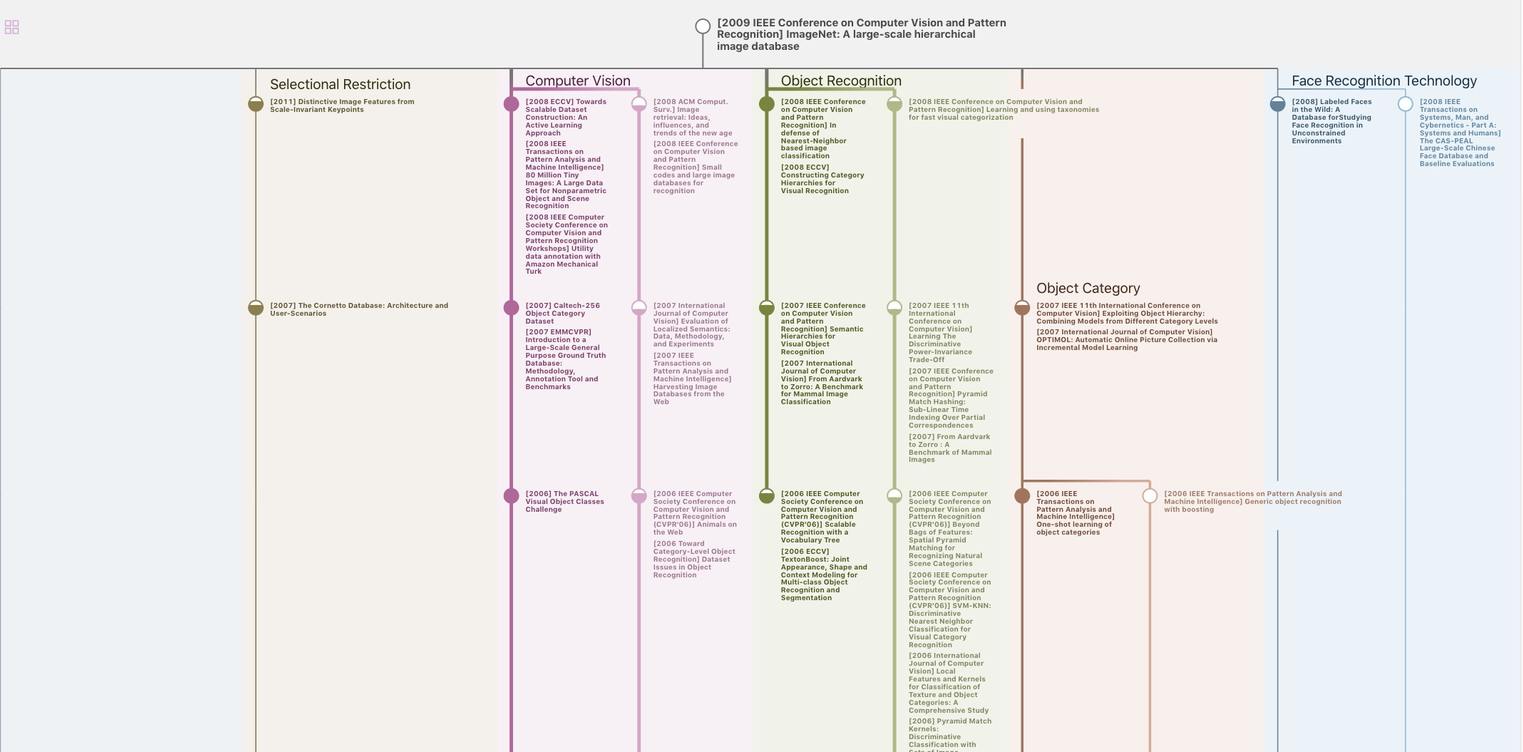
生成溯源树,研究论文发展脉络
Chat Paper
正在生成论文摘要