Consecutive Question Generation via Dynamic Multitask Learning
arxiv(2022)
摘要
In this paper, we propose the task of consecutive question generation (CQG), which generates a set of logically related question-answer pairs to understand a whole passage, with a comprehensive consideration of the aspects including accuracy, coverage, and informativeness. To achieve this, we first examine the four key elements of CQG, i.e., question, answer, rationale, and context history, and propose a novel dynamic multitask framework with one main task generating a question-answer pair, and four auxiliary tasks generating other elements. It directly helps the model generate good questions through both joint training and self-reranking. At the same time, to fully explore the worth-asking information in a given passage, we make use of the reranking losses to sample the rationales and search for the best question series globally. Finally, we measure our strategy by QA data augmentation and manual evaluation, as well as a novel application of generated question-answer pairs on DocNLI. We prove that our strategy can improve question generation significantly and benefit multiple related NLP tasks.
更多查看译文
关键词
generation,learning
AI 理解论文
溯源树
样例
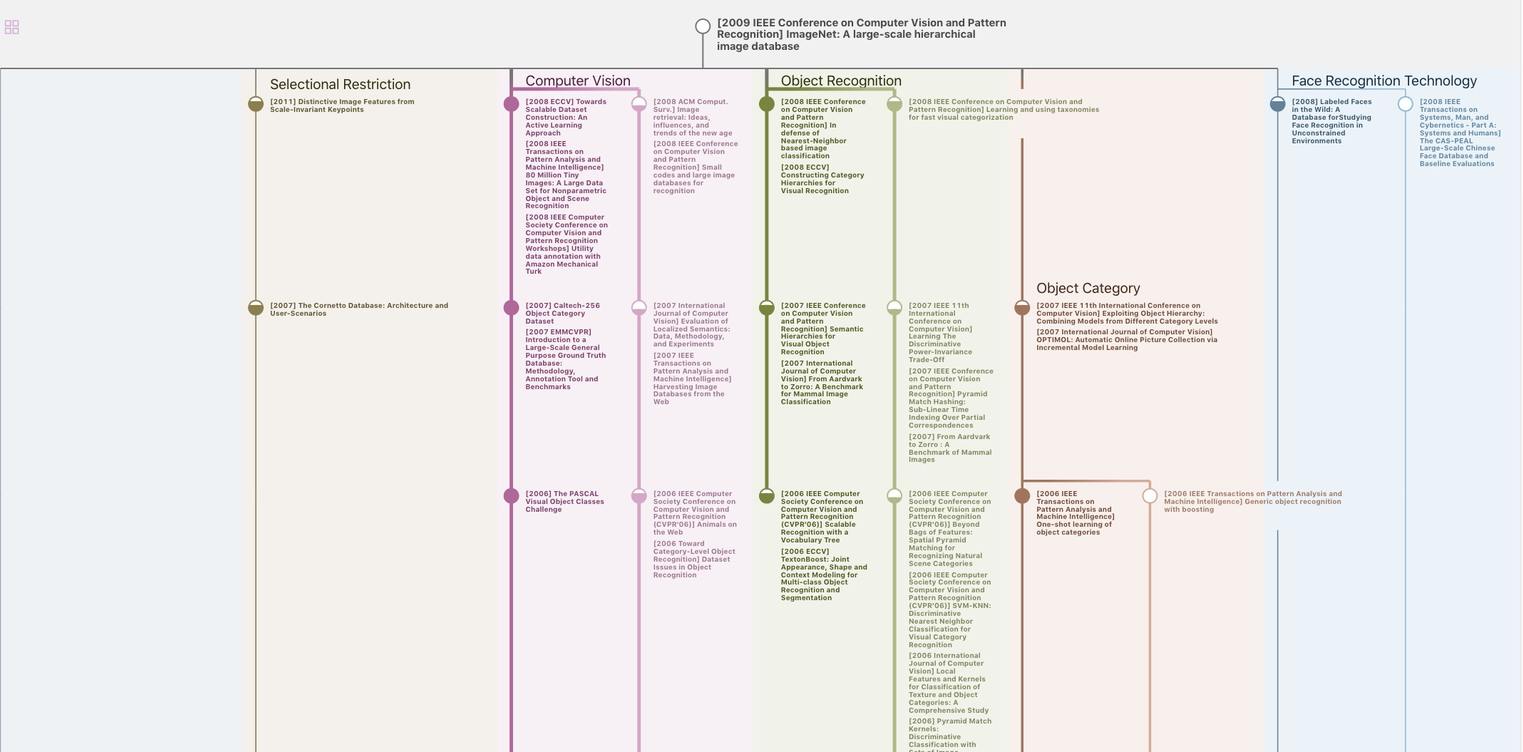
生成溯源树,研究论文发展脉络
Chat Paper
正在生成论文摘要