Efficiently Finding Adversarial Examples with DNN Preprocessing
arxiv(2022)
摘要
Deep Neural Networks (DNNs) are everywhere, frequently performing a fairly complex task that used to be unimaginable for machines to carry out. In doing so, they do a lot of decision making which, depending on the application, may be disastrous if gone wrong. This necessitates a formal argument that the underlying neural networks satisfy certain desirable properties. Robustness is one such key property for DNNs, particularly if they are being deployed in safety or business critical applications. Informally speaking, a DNN is not robust if very small changes to its input may affect the output in a considerable way (e.g. changes the classification for that input). The task of finding an adversarial example is to demonstrate this lack of robustness, whenever applicable. While this is doable with the help of constrained optimization techniques, scalability becomes a challenge due to large-sized networks. This paper proposes the use of information gathered by preprocessing the DNN to heavily simplify the optimization problem. Our experiments substantiate that this is effective, and does significantly better than the state-of-the-art.
更多查看译文
关键词
adversarial examples
AI 理解论文
溯源树
样例
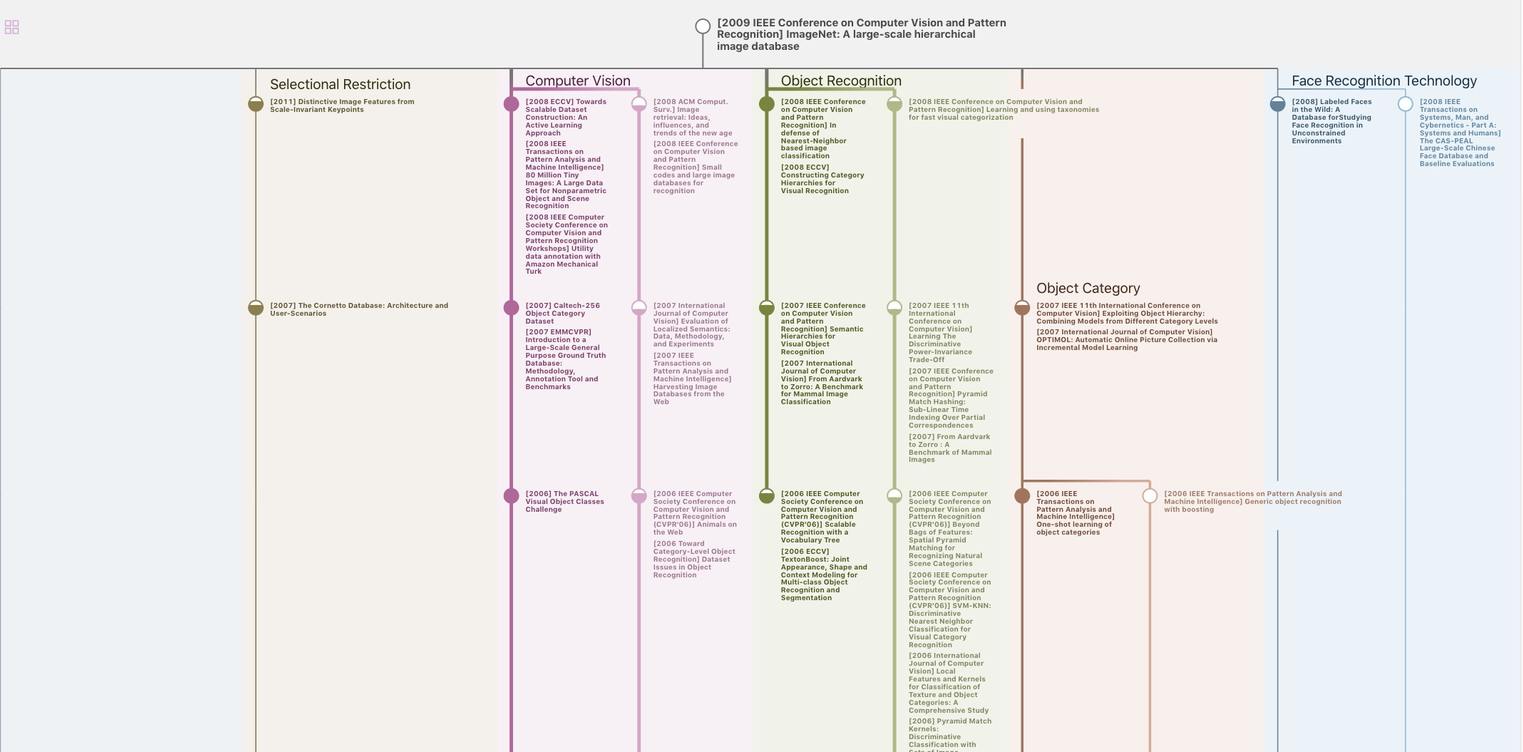
生成溯源树,研究论文发展脉络
Chat Paper
正在生成论文摘要