Strategyproof Allocation Mechanisms with Endowments and M-convex Distributional Constraints
ARTIFICIAL INTELLIGENCE(2023)
摘要
We consider an allocation problem of multiple types of objects to agents, where each type of object has multiple copies (e.g., multiple seats in a school), each agent is endowed with an object, and some distributional constraints are imposed on the allocation (e.g., minimum/maximum quotas). We develop two mechanisms that are strategyproof, feasible (they always satisfy distributional constraints), and individually rational, assuming the distributional constraints are represented by an M-convex set. One mechanism, based on Top Trading Cycles, is Pareto efficient; the other, which belongs to the mechanism class specified by Kojima et al. [1], satisfies a relaxed fairness requirement. The class of distributional constraints we consider contains many situations raised from realistic matching problems, including individual minimum/maximum quotas, regional maximum quotas, type-specific quotas, and distance constraints. Finally, we experimentally evaluate the performance of these mechanisms by a computer simulation.(c) 2022 The Authors. Published by Elsevier B.V. This is an open access article under the CC BY license (http://creativecommons.org/licenses/by/4.0/).
更多查看译文
关键词
Controlled school choice,M-convex set,Strategyproofness,Top trading cycles,Deferred acceptance,Distributional constraints
AI 理解论文
溯源树
样例
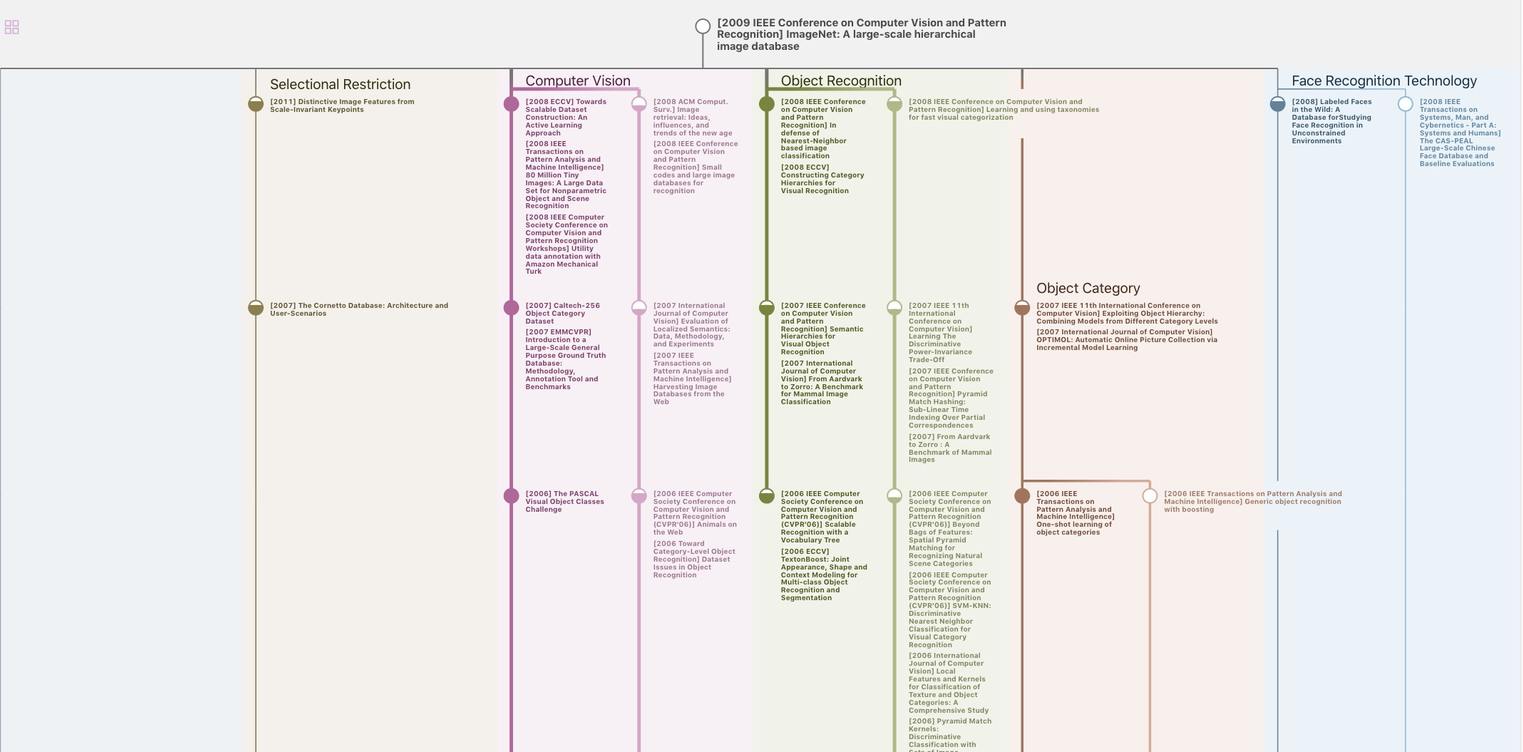
生成溯源树,研究论文发展脉络
Chat Paper
正在生成论文摘要