Solving clustering as ill-posed problem: experiments with K-Means algorithm
arxiv(2022)
摘要
In this contribution, the clustering procedure based on K-Means algorithm is studied as an inverse problem, which is a special case of the illposed problems. The attempts to improve the quality of the clustering inverse problem drive to reduce the input data via Principal Component Analysis (PCA). Since there exists a theorem by Ding and He that links the cardinality of the optimal clusters found with K-Means and the cardinality of the selected informative PCA components, the computational experiments tested the theorem between two quantitative features selection methods: Kaiser criteria (based on imperative decision) versus Wishart criteria (based on random matrix theory). The results suggested that PCA reduction with features selection by Wishart criteria leads to a low matrix condition number and satisfies the relation between clusters and components predicts by the theorem. The data used for the computations are from a neuroscientific repository: it regards healthy and young subjects that performed a task-oriented functional Magnetic Resonance Imaging (fMRI) paradigm.
更多查看译文
关键词
clustering,algorithm,ill-posed,k-means
AI 理解论文
溯源树
样例
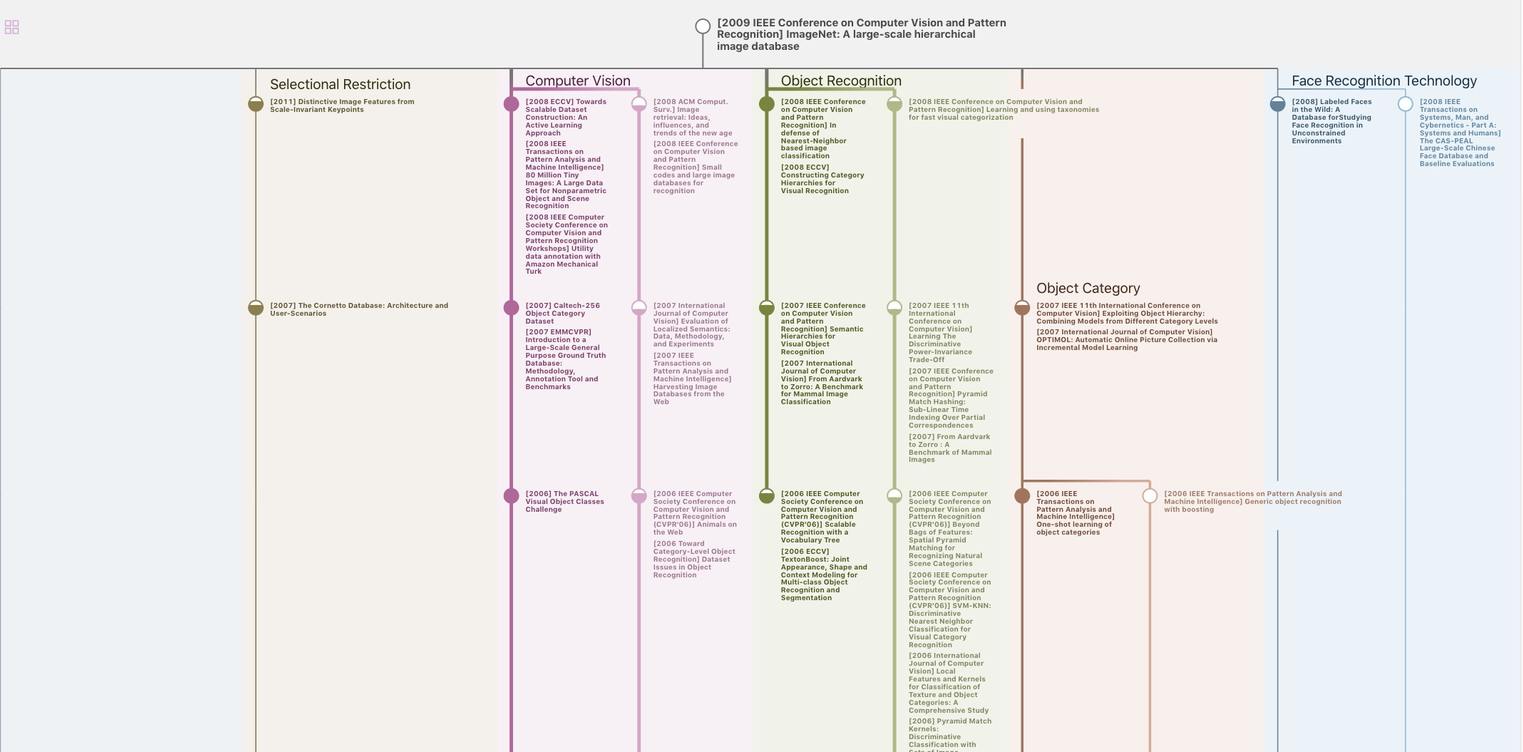
生成溯源树,研究论文发展脉络
Chat Paper
正在生成论文摘要