Bayesian Federated Neural Matching that Completes Full Information
arxiv(2023)
摘要
Federated learning is a contemporary machine learning paradigm where locally trained models are distilled into a global model. Due to the intrinsic permutation invariance of neural networks, Probabilistic Federated Neural Matching (PFNM) employs a Bayesian nonparametric framework in the generation process of local neurons, and then creates a linear sum assignment formulation in each alternative optimization iteration. But according to our theoretical analysis, the optimization iteration in PFNM omits global information from existing. In this study, we propose a novel approach that overcomes this flaw by introducing a Kullback-Leibler divergence penalty at each iteration. The effectiveness of our approach is demonstrated by experiments on both image classification and semantic segmentation tasks.
更多查看译文
关键词
matching
AI 理解论文
溯源树
样例
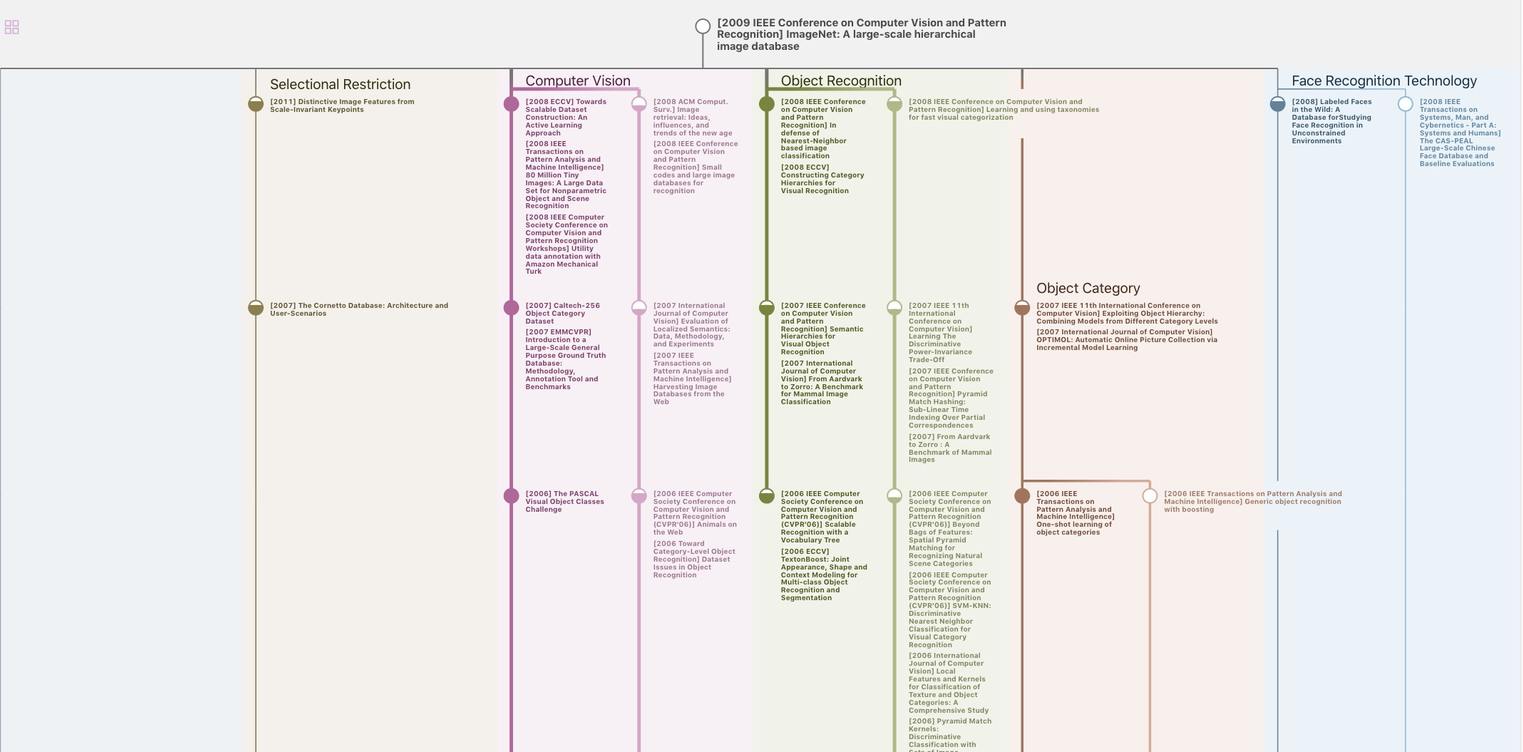
生成溯源树,研究论文发展脉络
Chat Paper
正在生成论文摘要