An online algorithm for contrastive Principal Component Analysis
arxiv(2022)
摘要
Finding informative low-dimensional representations that can be computed efficiently in large datasets is an important problem in data analysis. Recently, contrastive Principal Component Analysis (cPCA) was proposed as a more informative generalization of PCA that takes advantage of contrastive learning. However, the performance of cPCA is sensitive to hyper-parameter choice and there is currently no online algorithm for implementing cPCA. Here, we introduce a modified cPCA method, which we denote cPCA*, that is more interpretable and less sensitive to the choice of hyper-parameter. We derive an online algorithm for cPCA* and show that it maps onto a neural network with local learning rules, so it can potentially be implemented in energy efficient neuromorphic hardware. We evaluate the performance of our online algorithm on real datasets and highlight the differences and similarities with the original formulation.
更多查看译文
关键词
Contrastive principal component analysis,online algorithm,neural network,local learning rules
AI 理解论文
溯源树
样例
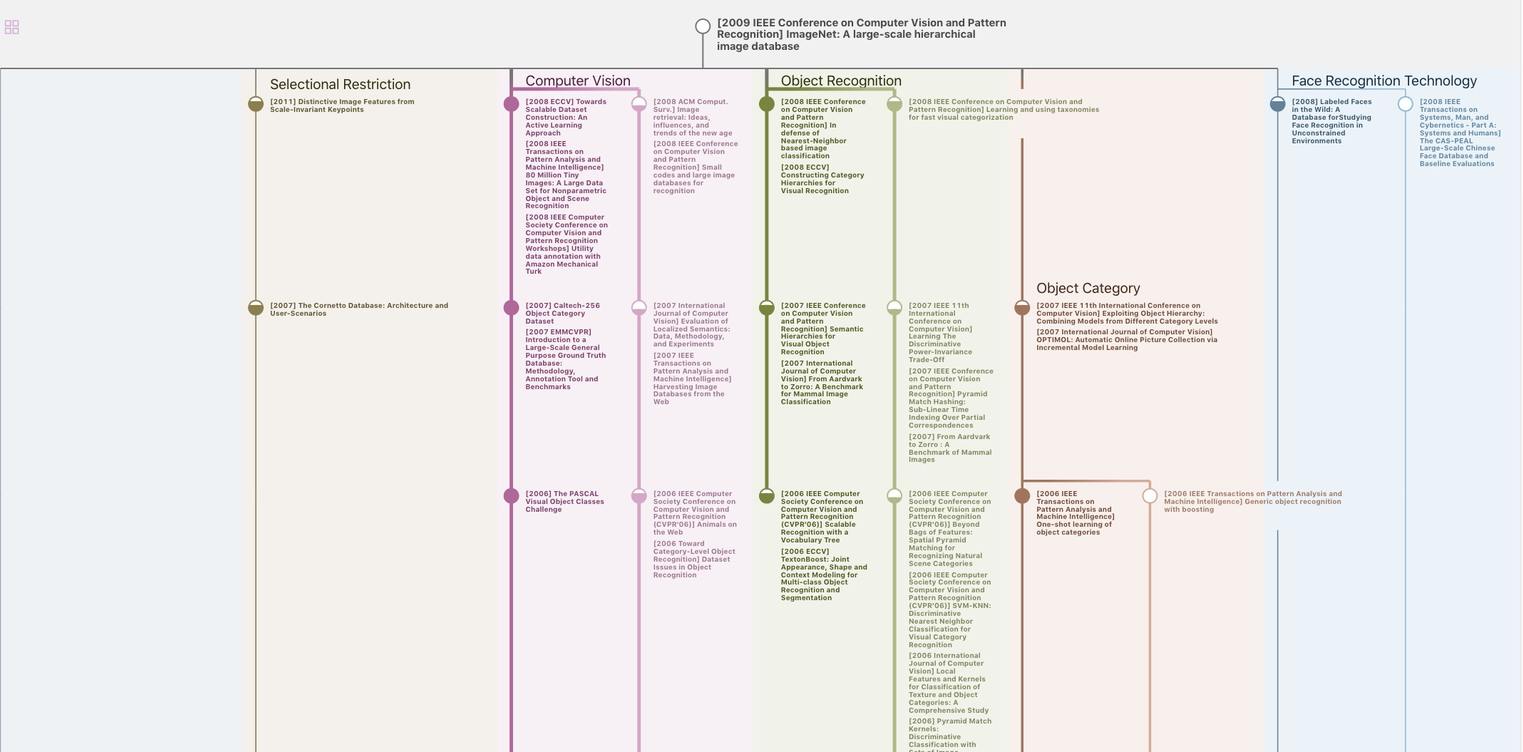
生成溯源树,研究论文发展脉络
Chat Paper
正在生成论文摘要