HigeNet: A Highly Efficient Modeling for Long Sequence Time Series Prediction in AIOps
arxiv(2022)
摘要
Modern IT system operation demands the integration of system software and hardware metrics. As a result, it generates a massive amount of data, which can be potentially used to make data-driven operational decisions. In the basic form, the decision model needs to monitor a large set of machine data, such as CPU utilization, allocated memory, disk and network latency, and predicts the system metrics to prevent performance degradation. Nevertheless, building an effective prediction model in this scenario is rather challenging as the model has to accurately capture the long-range coupling dependency in the Multivariate Time-Series (MTS). Moreover, this model needs to have low computational complexity and can scale efficiently to the dimension of data available. In this paper, we propose a highly efficient model named HigeNet to predict the long-time sequence time series. We have deployed the HigeNet on production in the D-matrix platform. We also provide offline evaluations on several publicly available datasets as well as one online dataset to demonstrate the model's efficacy. The extensive experiments show that training time, resource usage and accuracy of the model are found to be significantly better than five state-of-the-art competing models.
更多查看译文
关键词
long sequence time series
AI 理解论文
溯源树
样例
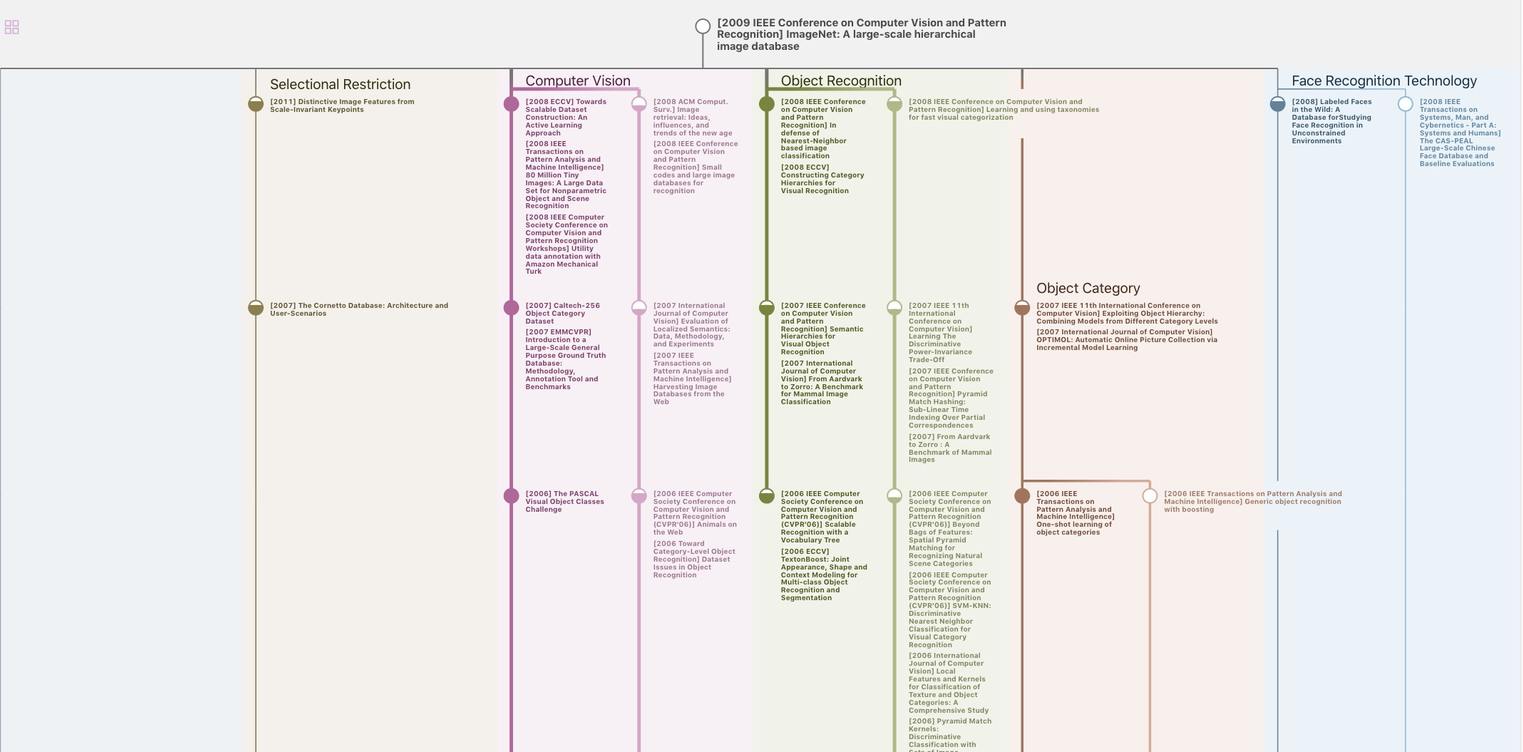
生成溯源树,研究论文发展脉络
Chat Paper
正在生成论文摘要