Inductive Representation Learning of Multiple ICD Codes for Healthcare
2022 IEEE 17th International Conference on Control & Automation (ICCA)(2022)
摘要
The International Classification of Disease (ICD) coding scheme authorized by the World Health Organization, is a standard used to classify medical diagnosis in health insurance claims. One of the key challenges in adopting machine learning techniques for health insurance claims processing lies in the sparse and complex nature of ICD codes. There are over 69,000 unique diagnosis codes in the 10th version of the ICD coding scheme where multiple codes are used to represent complex medical diagnosis. In this work, we address the challenge of embedding both single and multiple ICD codes into a vector space. This representation addresses the sparse representation of ICD codes by providing a more compact representation that captures their diagnosis relationship. Our proposed inductive multi-code representation introduces a novel application of inductive node embedding by representing multiple ICD codes as a multi-code node. This enables both single and multiple ICD codes to be represented in the same vector space. For multi-code relevance, our proposed approached surpassed the benchmark node2vec implementation by 34%, highlighting its effectiveness.
更多查看译文
关键词
inductive representation learning,multiple ICD codes,Disease coding scheme,health insurance claims processing,69 diagnosis codes,000 unique diagnosis codes,ICD coding scheme,multiple codes,inductive multicode representation,multicode node,multicode relevance
AI 理解论文
溯源树
样例
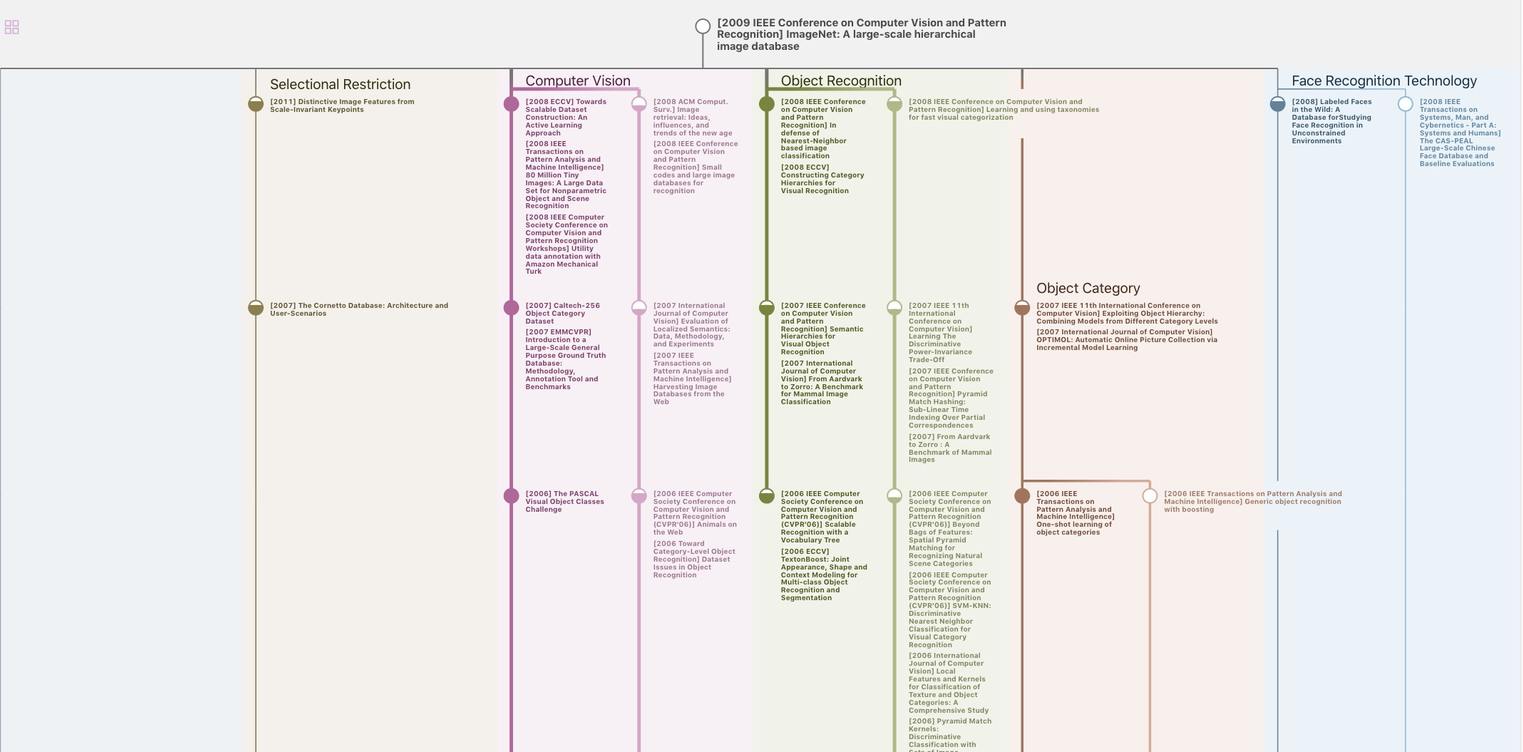
生成溯源树,研究论文发展脉络
Chat Paper
正在生成论文摘要