Model-Assisted Reinforcement Learning for Online Diagnostics in Stochastic Controlled Systems
2022 IEEE 17th International Conference on Control & Automation (ICCA)(2022)
摘要
A mechanism to protect a controlled system in the event of a priori unknown abnormalities (e.g. faults, attacks) is the key to designing resilient and robust control systems. We explore bi-level control design architectures in which a supervisory Reinforcement Learning (RL) agent augments an over-observed controlled system. The RL agent monitors sensor signals, detects and takes action to mitigate unknown sensor faults. We use the system dynamics to extract features and develop a design method for the cost function of the RL module. We theoretically show that the designed cost function has a unique optimal policy that enables the diagnosis of arbitrary constant sensor faults. To conceptualize our architecture, we consider a linear version of an over-observed chemical process, controlled by a Linear Quadratic Gaussian (LQG) Servo-Controller with Integral Action. Our experimental results, coupled with our theoretical analysis, show that the RL-agent is successful in identifying and mitigating the faults in one or more sensors in an online fashion.
更多查看译文
关键词
model-assisted Reinforcement Learning,online diagnostics,stochastic controlled systems,a priori unknown abnormalities,resilient control systems,robust control systems,bi-level control design architectures,supervisory Reinforcement,over-observed controlled system,RL agent monitors sensor signals,unknown sensor faults,system dynamics,design method,RL module,designed cost function,unique optimal policy,arbitrary constant sensor faults,over-observed chemical process,Linear Quadratic Gaussian Servo-Controller,RL-agent,online fashion
AI 理解论文
溯源树
样例
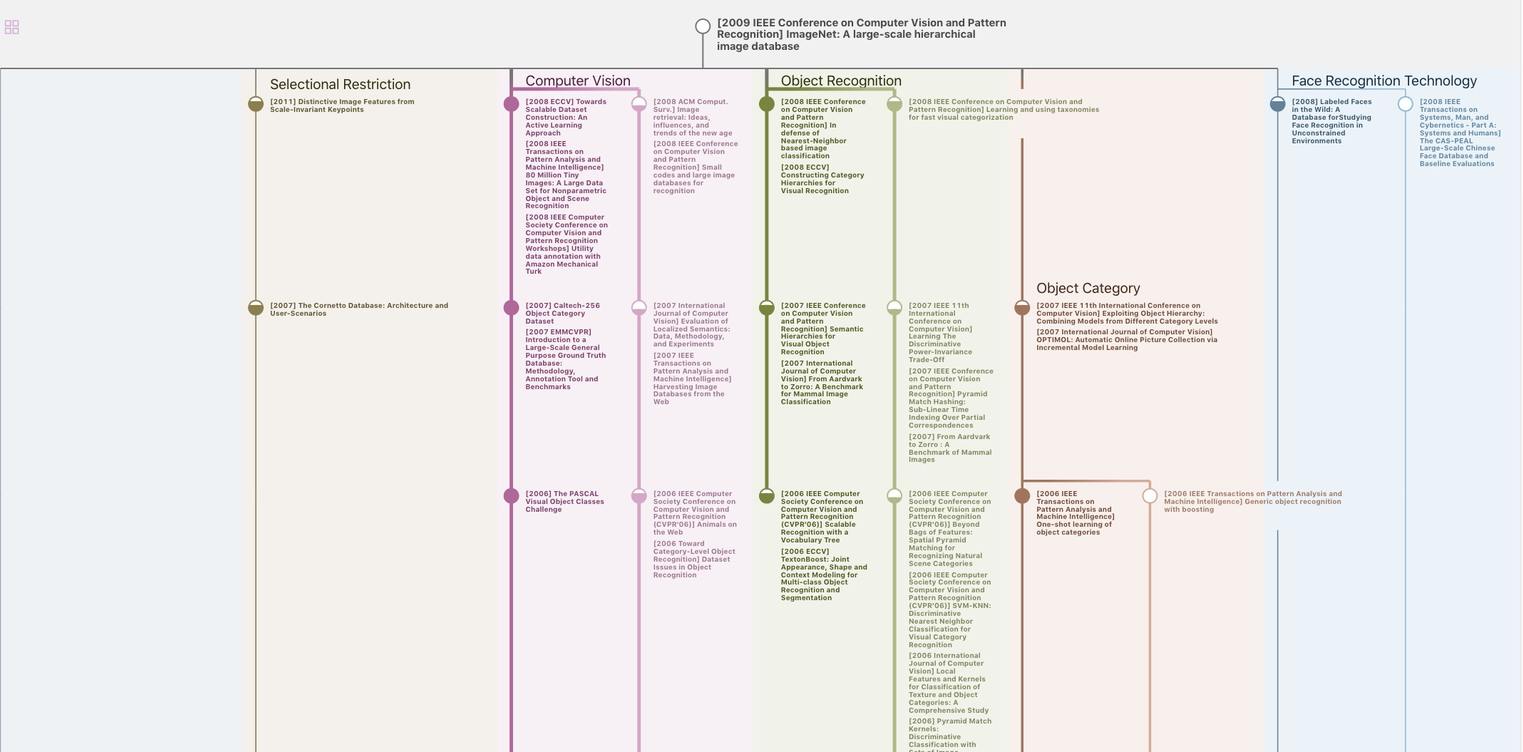
生成溯源树,研究论文发展脉络
Chat Paper
正在生成论文摘要